Suggestions or feedback?

MIT News | Massachusetts Institute of Technology
- Machine learning
- Sustainability
- Black holes
- Classes and programs
Departments
- Aeronautics and Astronautics
- Brain and Cognitive Sciences
- Architecture
- Political Science
- Mechanical Engineering
Centers, Labs, & Programs
- Abdul Latif Jameel Poverty Action Lab (J-PAL)
- Picower Institute for Learning and Memory
- Lincoln Laboratory
- School of Architecture + Planning
- School of Engineering
- School of Humanities, Arts, and Social Sciences
- Sloan School of Management
- School of Science
- MIT Schwarzman College of Computing
Download RSS feed: News Articles / In the Media / Audio
For developing designers, there’s magic in 2.737 (Mechatronics)
Mechatronics combines electrical and mechanical engineering, but above all else it’s about design.
September 3, 2024
Read full story →
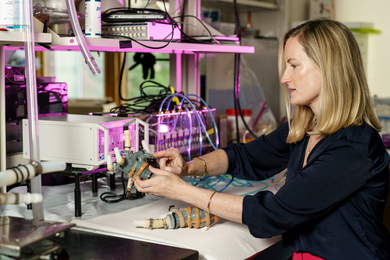
Engineering and matters of the heart
Professor Ellen Roche is creating the next generation of medical devices to help repair hearts, lungs, and other tissues.
August 21, 2024
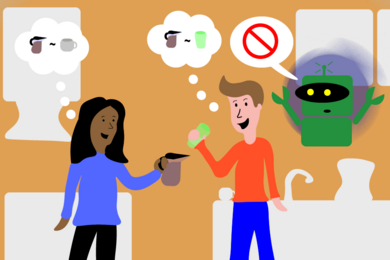
AI assistant monitors teamwork to promote effective collaboration
An AI team coordinator aligns agents’ beliefs about how to achieve a task, intervening when necessary to potentially help with tasks in search and rescue, hospitals, and video games.
August 19, 2024
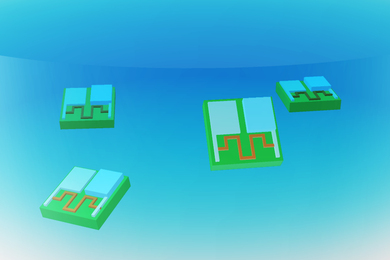
MIT engineers design tiny batteries for powering cell-sized robots
These zinc-air batteries, smaller than a grain of sand, could help miniscule robots sense and respond to their environment.
August 15, 2024
A new model offers robots precise pick-and-place solutions
SimPLE learns to pick, regrasp, and place objects using the objects’ computer-aided design model.
August 9, 2024
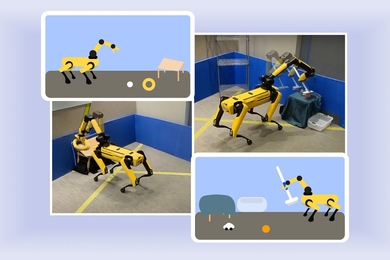
Helping robots practice skills independently to adapt to unfamiliar environments
A new algorithm helps robots practice skills like sweeping and placing objects, potentially helping them improve at important tasks in houses, hospitals, and factories.
August 8, 2024
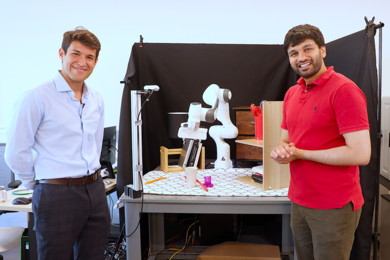
Precision home robots learn with real-to-sim-to-real
CSAIL researchers introduce a novel approach allowing robots to be trained in simulations of scanned home environments, paving the way for customized household automation accessible to anyone.
July 31, 2024
Flying high to enable sustainable delivery, remote care
Drone company founders with MIT Advanced Study Program roots seek to bring aerial delivery to the mainstream.
July 25, 2024

Creating and verifying stable AI-controlled systems in a rigorous and flexible way
Neural network controllers provide complex robots with stability guarantees, paving the way for the safer deployment of autonomous vehicles and industrial machines.
July 17, 2024
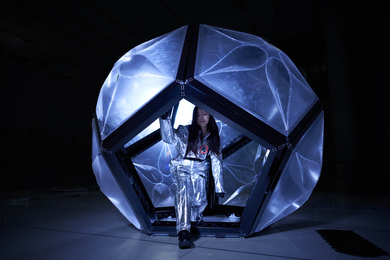
Designing for outer space
With NASA planning permanent bases in space and on the moon, MIT students develop prototypes for habitats far from planet Earth.
June 23, 2024

Researchers use large language models to help robots navigate
The method uses language-based inputs instead of costly visual data to direct a robot through a multistep navigation task.
June 12, 2024
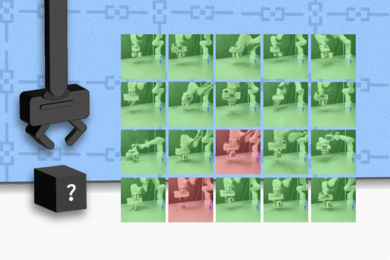
Helping robots grasp the unpredictable
MIT CSAIL’s frugal deep-learning model infers the hidden physical properties of objects, then adapts to find the most stable grasps for robots in unstructured environments like homes and fulfillment centers.
June 3, 2024
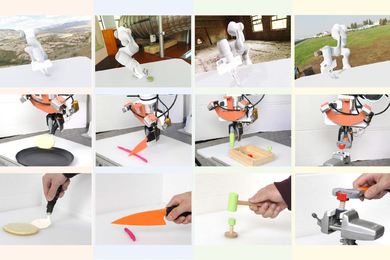
A technique for more effective multipurpose robots
With generative AI models, researchers combined robotics data from different sources to help robots learn better.
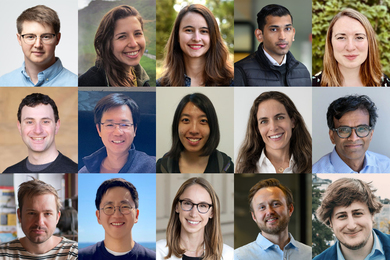
School of Engineering welcomes new faculty
Fifteen new faculty members join six of the school’s academic departments.
May 23, 2024
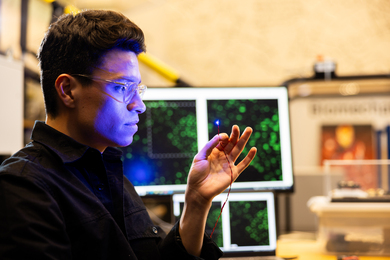
MIT scientists learn how to control muscles with light
A new study suggests optogenetics can drive muscle contraction with greater control and less fatigue than electrical stimulation.
May 22, 2024
Massachusetts Institute of Technology 77 Massachusetts Avenue, Cambridge, MA, USA
- Map (opens in new window)
- Events (opens in new window)
- People (opens in new window)
- Careers (opens in new window)
- Accessibility
- Social Media Hub
- MIT on Facebook
- MIT on YouTube
- MIT on Instagram
- Who’s Teaching What
- Subject Updates
- MEng program
- Opportunities
- Minor in Computer Science
- Resources for Current Students
- Program objectives and accreditation
- Graduate program requirements
- Admission process
- Degree programs
- Graduate research
- EECS Graduate Funding
- Resources for current students
- Student profiles
- Instructors
- DEI data and documents
- Recruitment and outreach
- Community and resources
- Get involved / self-education
- Rising Stars in EECS
- Graduate Application Assistance Program (GAAP)
- MIT Summer Research Program (MSRP)
- Sloan-MIT University Center for Exemplary Mentoring (UCEM)
- Electrical Engineering
- Computer Science
- Artificial Intelligence + Decision-making
- AI and Society
- AI for Healthcare and Life Sciences
- Artificial Intelligence and Machine Learning
- Biological and Medical Devices and Systems
- Communications Systems
- Computational Biology
- Computational Fabrication and Manufacturing
- Computer Architecture
- Educational Technology
- Electronic, Magnetic, Optical and Quantum Materials and Devices
- Graphics and Vision
- Human-Computer Interaction
- Information Science and Systems
- Integrated Circuits and Systems
- Nanoscale Materials, Devices, and Systems
- Natural Language and Speech Processing
- Optics + Photonics
- Optimization and Game Theory
- Programming Languages and Software Engineering
- Quantum Computing, Communication, and Sensing
- Security and Cryptography
- Signal Processing
- Systems and Networking
- Systems Theory, Control, and Autonomy
- Theory of Computation
- Departmental History
- Departmental Organization
- Visiting Committee
- Explore all research areas
Our research focuses on robotic hardware and algorithms, from sensing to control to perception to manipulation.
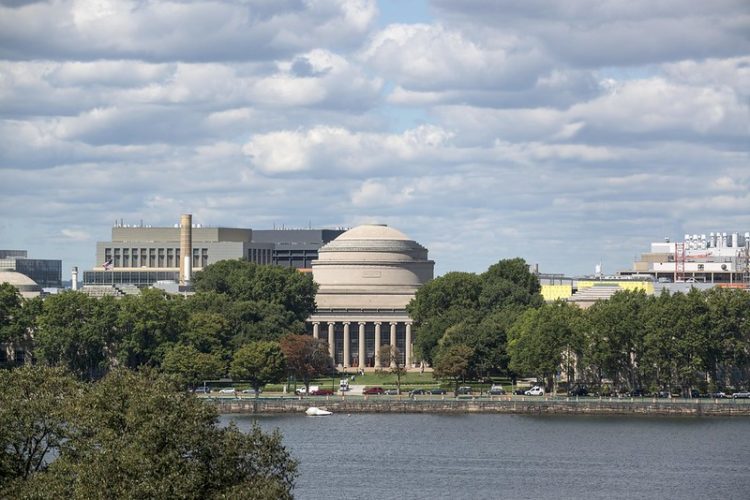
Latest news in robotics
Helping robots practice skills independently to adapt to unfamiliar environments.
A new algorithm helps robots practice skills like sweeping and placing objects, potentially helping them improve at important tasks in houses, hospitals, and factories.
Creating and verifying stable AI-controlled systems in a rigorous and flexible way
Neural network controllers provide complex robots with stability guarantees, paving the way for the safer deployment of autonomous vehicles and industrial machines.
A technique for more effective multipurpose robots
With generative AI models, researchers combined robotics data from different sources to help robots learn better.
QS ranks MIT the world’s No. 1 university for 2024-25
Ranking at the top for the 13th year in a row, the Institute also places first in 11 subject areas.
Five MIT faculty elected to the National Academy of Sciences for 2024
Guoping Feng, Piotr Indyk, Daniel Kleitman, Daniela Rus, Senthil Todadri, and nine alumni are recognized by their peers for their outstanding contributions to research.
Upcoming events
Ai and the future of your career, eecs career fair, five rings tech talk – demystifying proprietary trading .
Robotics is poised to revolutionize work, education, and everyday life in much the same way the Internet did over past decades.
Today, some of the most innovative and foundational robotics work is being done at SEAS and across Harvard by collaborative teams of computer scientists, mechanical engineers, electrical engineers, material scientists, applied mathematicians, designers, and medical experts.
Researchers at SEAS benefit from access to in-house resources such as our motional capture labs, flight labs, soft robotics lab, research cores , scientific shops , and the Harvard Move Lab . They have access to the advanced manufacturing capabilities provided by Harvard Center for Nanoscale Research . Some researchers at SEAS work collaboratively with the Harvard Medical School, The Harvard Graduate School of Design, and the Wyss Institute at Harvard .
Robotics research at SEAS spans topics that are both basic and applied. Some areas of focus include:
- soft wearable robots for physical rehabilitation, assistive movement, ergonomic support, and enhanced training.
- medical robots for automated and minimally invasive surgical procedures
- autonomous search and rescue robots to assist first responders in natural or man-made disasters
- automated assembly at scales ranging from micrometer to meter
- bioinspired robots across a range of physical forms
- industrial robots for the automation of manufacturing or shipping
- smart clothing that senses and responds to human needs
- metamaterials that move and transform is novel ways
Research Areas
- Bioinspired Robotics and Computing
- Robotics and Control
Featured Stories
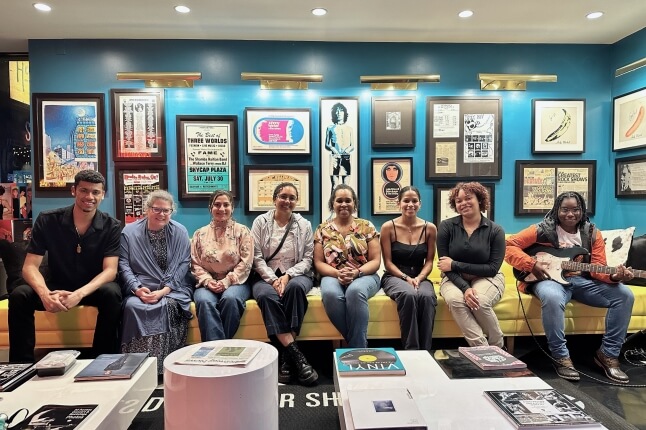
A bridge from undergraduate to graduate studies
Post-baccalaureate program help students transition to the next academic level
Academics , Applied Physics , Bioengineering , Diversity / Inclusion , Environmental Science & Engineering , Materials Science & Mechanical Engineering , Optics / Photonics , Quantum Engineering , Robotics
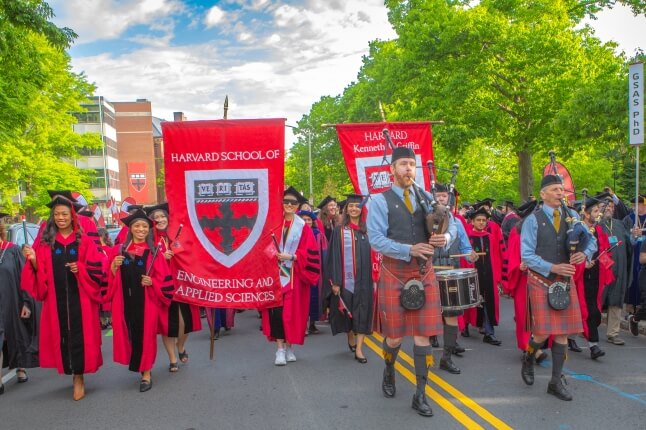
2024 Commencement photos
Images from the 373rd Harvard Commencement on Thursday, May 23
Academics , Applied Computation , Applied Mathematics , Applied Physics , Bioengineering , Computer Science , Environmental Science & Engineering , Events , Materials Science & Mechanical Engineering , Robotics
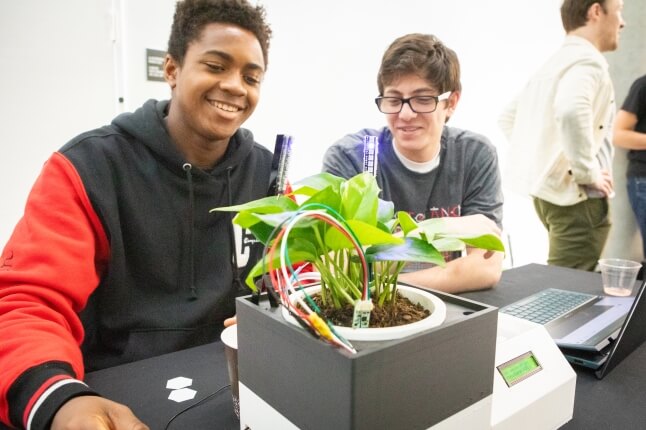
An engineer for every interest
Design Fair highlights range of engineering projects at SEAS
Academics , Active Learning Labs , REEF Makerspace , Allston Campus , Bioengineering , Design , Electrical Engineering , Environmental Science & Engineering , Materials Science & Mechanical Engineering , Robotics , Student Organizations
Student Stories
Alumni stories.
- Menu Close
- Search
Researchers are working to develop and deploy advanced robotics systems that function effectively in the real world.
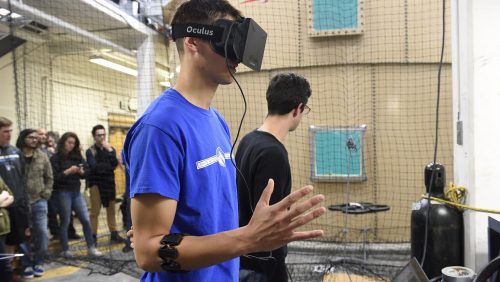
Robotics research focuses on understanding and designing intelligent robotic systems through rigorous analysis, system development, and field deployment.
Hopkins researchers develop techniques and tools for kinematics, dynamics, control, estimation, and motion planning for deterministic and stochastic systems. Novel design principles are developed using modular self reconfigurable robots, self-replicating robots, and hyper-redundant manipulators.
Navigation and interaction of robots in extreme environments – e.g. deep sea, space, and the human body – is a major focus, including applications in underwater vehicles, autonomous aerial vehicles and spacecraft, and surgical robotics.
Also important to our mission is the development of robotic systems inspired by principles of biomechanics and neural control of animal movement. Closely related application areas include medicine, environmental assessment, and molecular biology.
Many of our faculty are affiliated with The Laboratory for Computational Sensing and Robotics (LCSR) , one of the top robotics research sites in the world, particularly in the area of medical robotics.
Specialties
- Robotics in Extreme Environments
- Haptics and Medical Robotics
- Bio-Robotics
- Autonomous Systems, Control, and Optimization
- Human-Machine Collaborative Systems
Jeremy Brown
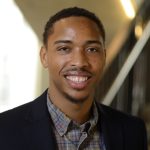
Axel Krieger
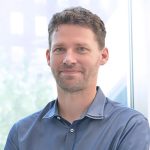
Marin Kobilarov
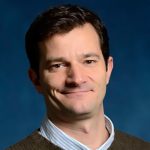
Louis Whitcomb
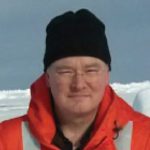
Affiliated Faculty
- Mehran Armand
- Emad Boctor
- Ralph Etienne-Cummings
- Gabor Fichtinger
- Gregory D. Hager
- Peter Kazanzides
- Allison M. Okamura
- Jerry Prince
- Suchi Saria
- Dan Stoianovici
- Russell H. Taylor
Affiliated Groups, Centers, and Institutes
- The Laboratory for Computational Sensing and Robotics
- Center for Speech and Language Processing
- The Malone Center for Engineering in Healthcare
- Haptics and Medical Robotics (HAMR) Laboratory (Brown)
- Robot and Protein Kinematics Laboratory (Chirikjian)
- Locomotion in Mechanical and Biological Systems (Cowan)
- Advanced Medical Instrumentation and Robotics (Iordachita)
- Intelligent Medical Robotic Systems and Equipment Lab (Krieger)
- Autonomous Systems, Control, and Optimization Lab (Kobilarov)
- Terradynamics Laboratory (Li)
- Medical Robotics Lab (Stoianovici)
- Dynamical Systems and Control Laboratory (Whitcomb)
Next-Gen Robotics
At the forefront of robotics research
Animal and Robot Locomotion in Complex Terrains - In the Terradynamics Laboratory led by Chen Li, researchers study the movement of cockroaches and snakes to teach robots to navigate complex terrains like building rubble and forest floors.
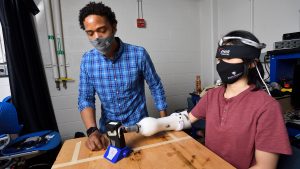
Get a Grip - Through neuroimaging, Jeremy Brown’s team discovers that prosthetics that provide haptic sensory feedback lessen the mental energy users expend when using the device.
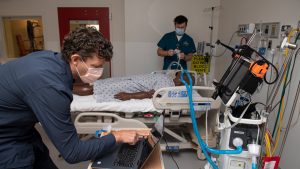
Remote Control for COVID-19 Patient Ventilators - Axel Krieger and team have built a robotic system that will give medical staff the ability to remotely operate ventilators and other bedside machines from outside intensive care rooms of patients with infectious diseases like COVID-19.
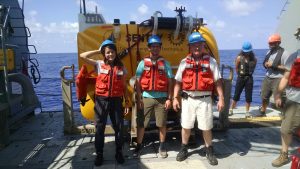
Autonomous Underwater Vehicles - Louis Whitcomb’s lab has participated in the development of underwater vehicles for oceanographic science missions, including the Nereid Under-Ice (NUI) hybrid underwater vehicle deployed under Arctic sea ice in 1014, 2016, and 2019.
Related News
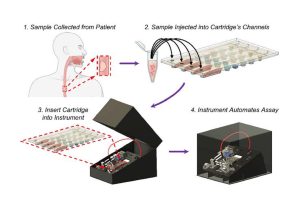
Collect, insert, diagnose: Revolutionizing ACCESS to cancer screening
- Autonomous Systems
- Biotechnology
- Student Experience
Shoebox-sized device promises earlier esophageal cancer detection in low-resource areas
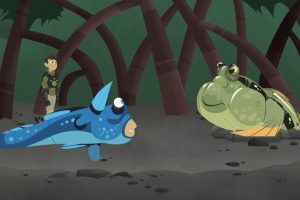
PBS’s Wild Kratts Visit Chen Li’s Terradynamics Lab
- Faculty News
“A Fish Out of Water,” featuring team’s mudskipper and robotics research, now streaming
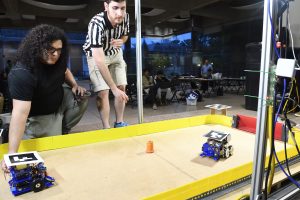
Robotics teams shoot it out in JHockey Tournament
- Undergraduate News
Back Bay Midnight Pedalers claim victory over Puck Me after three-hour-long, double-elimination contest
The future of robotics

Guest Jeannette Bohg is an expert in robotics who says there is transformation happening in her field brought on by recent advances in large language models.
The LLMs have a certain common sense baked in and robots are using it to plan and to reason as never before. But they still lack low-level sensorimotor control – like the fine skill it takes to turn a doorknob. New models that do for robotic control what LLMs did for language could soon make such skills a reality, Bohg tells host Russ Altman on this episode of Stanford Engineering’s The Future of Everything podcast.
Listen on your favorite podcast platform:
Related : Jeannette Bohg , assistant professor of computer science
[00:00:00] Jeannette Bohg: Through things like ChatGPT, we have been able to do reasoning and planning on the high level, meaning kind of on the level of symbols, very well known in robotics, in a very different way that we could do before.
[00:00:17] Russ Altman: This is Stanford Engineering's The Future of Everything, and I'm your host, Russ Altman. If you enjoy The Future of Everything, please hit follow in whatever app you're listening to right now. This will guarantee that you never miss an episode.
[00:00:29] Today, Professor Jeannette Bohg will tell us about robots and the status of robotic work. She'll tell us that ChatGPT is even useful for robots. And that there are huge challenges in getting reliable hardware so we can realize all of our robotic dreams. It's the future of robotics.
[00:00:48] Before we get started, please remember to follow the show and ensure that you'll get alerted to all the new episodes so you'll never miss the future, and I love saying this, of anything.
[00:01:04] Many of us have been thinking about robots since we were little kids. When are we going to get those robots that can make our dinner, clean our house, drive us around, make life really easy? Well, it turns out that there's still some challenges and they're significant for getting robots to work. There are hardware challenges.
[00:01:20] It turns out that the human hand is way better than most robotic manipulators. In addition, robots break. They work in some situations like factories, but those are dangerous robots. They just go right through whatever's in front of them.
[00:01:34] Well, Jeannette Bohg is a computer scientist at Stanford University and an expert on robotics. She's going to tell us that we are making good progress in building reliable hardware and in developing algorithms to help make robots do their thing. What's perhaps most surprising is even ChatGPT is helping the robotics community, even though it just does chats.
[00:01:58] So Jeannette, there's been an increased awareness of AI in the last year, especially because of things like ChatGPT and what they call these large language models. But you work in robotics, you're building robots that sense and move around. Is that AI revolution for like chat, is that affecting your world?
[00:02:15] Jeannette Bohg: Yeah. Um, yeah, very good question. It definitely does. Um, in, um, surprising ways, honestly. So I think for me, my research language has always been very interesting, but somewhat in the, you know, in the background from the kind of research I'm doing, which is like on robotic manipulation. And with the, um, with this rise of things like ChatGPT or large language models, suddenly, um, doors are being opened, uh, in robotics that were really pretty closed.
[00:02:46] Russ Altman: Metaphorical or physical or both?
[00:02:48] Jeannette Bohg: Physically. That's exactly, that's a very good question because physically robots are very bad at open doors, but metaphorically speaking, these, uh, we can talk about that as well, metaphorically speaking through things like ChatGPT, we have been able to do reasoning and planning on the high level. Meaning kind of on the level of symbols, very well known in robotics in a very different way that we could do before.
[00:03:12] So let's say, for example, you're in a kitchen and you want to make dinner. Um, and, uh, you know, there are so many steps that you have to do for that, right? And they don't have to do something, they don't necessarily have to do something with how you move your hands and all of this.
[00:03:27] It's really just like, I'm laying out the steps of what I'm going to do for making dinner. And this kind of reasoning is suddenly possible in a much more open-ended way, right? Because we can, uh, these language models, they have this common-sense knowledge kind of in baked in them. And now we can use them in robotics to do these task plans, right? That, um, that are really consisting of so many steps and they kind of make sense. It's not always correct.
[00:03:55] Russ Altman: Right, right.
[00:03:55] Jeannette Bohg: Um, I mean, if you try ChatGPT, you know, it's hallucinating thing. It's like making stuff up. Um, but, um, that's the challenge, uh, actually, and how to use these models in robotics. But the good thing is they open up these doors, metaphorically speaking again, um, to just do this task planning in an open-ended way. Um, and you know, and they can just like, um, they also allow to have this very natural interface between people and robots as well. That's another,
[00:04:26] Russ Altman: Great. So that's really fascinating. So. If I understood your answer, you said that like for a high level, here's kind of the high-level script of how to make dinner, you know, get the dishes, get the ingredients. Um, do you find that there's a level of detail, I think implied in your answer, is that there's a level of detail that you need to get the robot to do the right things that it's not yet able to specify.
[00:04:49] Are you optimistic that it will be able to do that? Or do you think it's going to be an entirely different approach to like, you know, move the manipulator arm to this position and grasp it gently? Do you think that that's going to be in the range of ChatGPT or will that be other algorithms?
[00:05:03] Jeannette Bohg: Yeah. So I think to some extent, again, like these, you know, common sense, um, understanding of the world is in there. So for example, the idea that a glass could be fragile and you have to pick it up in a gentle way, or, uh, let's say you have to grasp a hammer by the handle or, you know, the tool tip of, uh, that tool is like over here or something like this.
[00:05:26] These are things that actually, um, help a robot to also plan its motion. Not just kind of this high-level task planning, but actually understand where to grasp things and maybe how much pressure to apply. Um, but they still, uh, they still cannot be directly generate an action, right? Like, so the action that a robot needs to compute is basically how do I move my hand? Like where exactly, like every millisecond, uh, or at least every ten milliseconds or something like that. And that is not what these models do. Um, and that's totally fine because to do that, they need completely different, they would need completely different training data that actually has this information in there.
[00:06:09] Um, like the actual motion of the robot arm needs to be given to these models in order to do that kind of prediction. Um, and so I think, um, so yeah, so that is actually the biggest, one of the biggest challenges in robotics to get to the same level of data that you have in areas like natural language processing or computer vision, that these, uh, models like ChatGPT, have consumed so far, right?
[00:06:38] So that, these models have been trained on trillions of tokens, right? Like multiple trillions of tokens. I don't know what the current maximum is. Um, but it's like, yeah, a lot. And in robotics, we have, uh, more like in the order of hundred thousands data of data points, hundred thousands. This is like millions of, um, it's a, by, uh, the difference is a factor of millions.
[00:07:06] Russ Altman: Now let me just ask you about that because I'm surprised you say that because I think about in many cases robots are trying to do things that we see in video by humans all the time. Like probably on television you could find many, many examples of somebody picking up a glass or opening a door, but it sounds to me like that's not enough for you. Like, in other words, these pictures of people doing things that doesn't turn into useful training data for the robot. And I guess that kind of makes sense. Although I'm a little surprised that we haven't figured out a way to take advantage of all of that human action to inform the video action. So talk to me a little bit about that.
[00:07:43] Jeannette Bohg: Yeah, yeah. This is like a very interesting question. So the data that I said is too little right now, uh, in comparison to natural language processing and computer vision, that's really data that has been directly collected on the robot.
[00:07:54] Russ Altman: Okay. So it's robot examples of them reaching, them touching.
[00:07:58] Jeannette Bohg: Yeah. And so that's like painstakingly collected with like joysticks and stuff like this, right? Like it's very tedious. That's why it's, I don't think possible to get to the same level of data, but you bring up a very good point, right? Like on YouTube. I mean, I'm watching YouTube all the time to just figure out like how to do something right?
[00:08:16] And how to repair something or do this and that, and yeah, we are learning from that and we are learning when we are growing up from our parents or whoever is like showing us how to do things. And, um, we want robots to do exactly the same. Uh, and that is like a super interesting research question. Uh, but the reason why it's a research question and not solved, um, is that in a video, um, you see a hand of a person, for example. But this hand, like our hand, sorry, I actually cut myself.
[00:08:46] Russ Altman: Yes, I see that. For those who are listening, there's a little Band-Aid on Jeannette's hand.
[00:08:51] Jeannette Bohg: But our hand is actually amazing, right? Like we have these five fingers, we have like, I don't know, it's even difficult to actually count how many degrees of freedom and joints our hand has, but it's like twenty-seven or something like that. It's soft, it can be very stiff, but it can also be very compliant. It's like, an amazing universal tool. And our robot hands are completely different. Unfortunately, I don't have one here, but basically, it's like, like a gripper. Very easy, very, um, very simple. Um, and it's because of that, it's very limited in what it can do. Um, and it might also need to do, um, things that a person does or tasks that a person does in a completely different way.
[00:09:30] Russ Altman: I see, I see.
[00:09:31] Jeannette Bohg: To, um, you know, to achieve the same task if it's even possible at all. And so if a robot looks at a video of a person, it needs to somehow understand like, okay, how does this map, uh, to my, my body right. Like my body only has two.
[00:09:47] Russ Altman: Yeah, no, that's a really, so it's like, if somebody was watching Vladimir Horowitz play the piano, it's not very useful to show them a YouTube of Vladimir and say, just play it like that because he can do things that we can't do.
[00:09:59] Jeannette Bohg: Right. That's exactly right. And I've heard that Rachmaninoff, for example, uh, has like these insane, had these insanely big hands and therefore, um, he could play, uh, his pieces. But they had like, uh, you're basically in order to play it, you had like to have a very specific difference between your thumb and your pinky, for example, like the distance,
[00:10:20] Russ Altman: Span, the span of your,
[00:10:21] Jeannette Bohg: Yeah.
[00:10:21] Russ Altman: Okay. So that's a really good answer to my question is that the videos are relevant, but we, they're not dealing with beautiful human hands. And so there would have to be a translation of whatever was happening in the video to their world and it's and that would be difficult.
[00:10:37] Jeannette Bohg: Yes, that is difficult. But people are looking into this, right? Like that's a super interesting research question on actually how.
[00:10:43] Russ Altman: And because the positive the upside as we've talked about is that you would then have lots and lots of training data. If you could break that code of how to turn human actions in video into instructions for robot. Okay, that's extremely helpful.
[00:10:57] But I want to get to some of the technical details of your work because it's fascinating, but before we get there, another backup, background question is the goal for the robots. Are we trying to, I know you've written a lot about autonomous robots, but you've also talked about how robots can also work with humans to augment them.
[00:11:16] And I want to ask if those are points on a continuum. Like, it seems like autonomous would be different from augmenting a human, but maybe in your mind they work together. So how should we think about, and what should we expect the first generation or the second generation of robotic assistants to be like?
[00:11:34] Jeannette Bohg: Yeah, this is a very good question. So first of all, I would say, yes, uh, this is like, um, points on a spectrum, right? There are solutions, uh, on a spectrum from, uh, teleoperation, I would say, where you basically puppeteer a robot to do something that's typically done for data collection. Um, or, uh, you know, the, on the other end of the spectrum, you have this fully autonomous, it's basically a humanoid that we see in movies. Right.
[00:11:59] Russ Altman: That's like the vacuum cleaner in my living room, my Roomba.
[00:12:02] Jeannette Bohg: Right, right. Exactly. Yeah. That one is definitely autonomous.
[00:12:05] Russ Altman: It seems fully autonomous to me. I have no idea when it's going to go on or off or where it's going to go.
[00:12:12] Jeannette Bohg: Yeah. Nobody knows. Nobody knows.
[00:12:15] Russ Altman: Forgive me. Forgive me.
[00:12:16] Jeannette Bohg: You bought it. I also had one once, uh, back in the days and you know, I just turn it on and then I left because I knew it would take hours and hours to do what it needed to do. Um,
[00:12:26] Russ Altman: I'm sorry, that was a little bit of a distraction. But yeah, tell me about the, this, um, spectrum.
[00:12:31] Jeannette Bohg: Yeah. So I think there are ways in which, um, robots can really augment people in that, uh, they can, for example, um, they, uh, theoretically, they could have more strength, right? Like, so, uh, um, that there are lots of people who, it's not my area, but there are lots of people who built these exoskeletons or prosthetic devices, which I actually also find really interesting. They're typically very lightweight, uh, have an easy interface. Um, so that's interesting, but they can also kind of support people who have to lift heavy things, for example. So I think that's one way on how you can think about augmentation of people to help them. Another one is maybe still autonomous, but it's still augmenting people in a way.
[00:13:15] So one example I want to bring up, this is a shout out to, uh, Andrea Thomaz and Vivian Chu who are like, um, leading this, um, startup called Diligent Robotics and I recently heard a keynote from her at a conference. And I thought they did something really smart, which is they went first into hospitals, uh, to observe what nurses are doing all the, all day, right?
[00:13:37] Like, what are they doing with their hours? And to their surprise, what nurses really spend a lot of time on was just like shuttling around supplies between different places instead of actually taking care of patients, right? Which is what they're like trained to do and really good at, why are we using them to shuttle stuff around?
[00:13:55] And so what they decided is like, oh, we actually don't need a robot to do the patient care or do the stocking or whatever. What we actually need is a robot that just shuttled stuff around in a hospital, uh, where it still needs a hand to actually push elevator buttons and push door buttons and things like that. Or like maybe opening a door again, right? Um, like we had in the beginning. And I thought like, oh, this is such a great augmentation if you want, right? Like that. The nurses can actually now spend time on what they're really good at and what they're needed for and what they're trained for, which is patient care, and just stop worrying about where the supplies are, where things like blood samples or things have to go.
[00:14:36] Russ Altman: And it sounds like it might also create a, I don't know, I'm not going to say anything is easy, but a slightly more straightforward engineering challenge to start.
[00:14:45] Jeannette Bohg: Right. So I think we're so far away from general purpose robots, right? Like we, I, I don't know how long it's going to take, but it's still going to take a lot of time. And I think a smart way to bring robotics into our everyday world is to actually, uh, ideas like the ones from Diligent Robotics, where you really go and analyze what people quote unquote waste their time on. It's not really a waste of time, of course. But you know, it could be done in like a, in an automated way actually, um, to give people time for the things they're actually really good at and where robots are still very bad at.
[00:15:18] Um, yeah. So I think, um, we will probably see, hopefully see more of this, right? Like in the future, like very small things. You can think of Roomba, for example, doing something kind of very small and I don't know how good it is, like it's good enough,
[00:15:37] Russ Altman: Compared to ignoring our floors, which was our strategy for the first twenty-five years, this is a huge improvement. Because now, even if it's not a perfect sweep, it's more sweeping than we would do under normal circumstances.
[00:15:49] Jeannette Bohg: Yeah, I agree with that. So I think like these small ideas, right, like that are not again, like this general purpose robot. But, uh, like some very, uh, smart ideas about where robots can help people with things that they find really annoying, um, and are doable for current robotic technology. I think that's what we will see in the next a few years. Um, and again, like it's a, they are still autonomous again, but they are augmenting people in this way.
[00:16:16] Russ Altman: Right. That resolves that what I thought was attention, but you just explained why it's not really attention. This is the future of everything with Russ Altman. More with Jeannette Bohg next.
[00:16:41] Welcome back to The Future of Everything. I'm Russ Altman, your host, and I'm speaking with Professor Jeannette Bohg from Stanford University.
[00:16:47] In the last segment, we went over some of the challenges of autonomous versus augmenting robots. We talked a little bit about the data problems. And in this next segment, we're going to talk about hardware. What are robots going to look like? How are they going to work? How expensive are they going to be? I want to get into kind of a fun topic, which is the hardware. You made a brief mention of the hands, uh, and how amazing human hands are, but the current robotic hands, uh, they're not quite human yet.
[00:17:14] Um, where are we with hardware and what are the challenges and what are some of the exciting new developments?
[00:17:20] Jeannette Bohg: Yeah. Uh, hardware is hard. It's one thing that I've been told is a saying in Silicon Valley recently. But yeah, uh, I think hardware and robotics is one of the biggest challenges. And I think we have very good hardware when it comes to automation in, uh, places like factories that are, um, you know, building cars and all of this. And it's very reliable, right? And that's what you want. But when it comes to the kinds of platforms that we want to see at some point in our homes or in hospitals, again, um, these platforms have to be equally robust and durable and repeatable and all of this. Uh, but we're not there. We're not there. Like literally, uh, I'm constantly, uh, talking to my students and they're constantly repairing whatever else, whatever new things broken again with our robots. I mean, it's constant. Um,
[00:18:12] Russ Altman: But it's interesting to know, just interrupt you. But the guys at Ford Motor Company and the big industry, they have figured out, is it a question of budget? Is it a question that they just spend a lot of money on these robots or are they too simple compared to what you need? I just want to explore a little bit why those industrial ones are so good.
[00:18:30] Jeannette Bohg: Yeah, so that is a very good question. I think they are, um, first of all, they are still very expensive, uh, robots actually. So they still cost like, uh, multiple ten thousands of dollars. Um, but yeah, they are also, they have a very, they follow a very specific methodology, which is they have to be, um, very stiff, uh, meaning that not like our arms, uh, which are kind of naturally kind of, um, squishy and give in to any kind of things we may be bumping in. Uh, these robots are not, right? Like they're going to go no matter what, to a specific point you sent them to. And, um, that is just the way they are built. And maybe that's also why they are so robust, uh, as well. Um, but they are dangerous, right?
[00:19:15] Russ Altman: Yes.
[00:19:15] Jeannette Bohg: So that's why they're in cages. And, uh, people can't get close to them. Uh, and that's of course not what we want in the real world. So the kinds of robots that we work in the research world with are more geared towards like, oh, when can we bring them into someone's home, uh, or have them at least work alongside a person in warehouses or things like that. Um, and so these, this technology I think is just not quite as mature and as robust. Um, and also not produced in that, at that, um, you know, there are just not so many copies of those as there are of these industrial robots. And I think they're just not as optimized yet.
[00:19:53] Russ Altman: So when you said the robots cost tens of thousands of dollars, are those the robots you're using?
[00:19:58] Jeannette Bohg: Uh, yeah.
[00:19:59] Russ Altman: That your students are fixing all day?
[00:20:01] Jeannette Bohg: Yes, unfortunately, this is exactly right. Like I spent so much money from my lab on, on buying forty thousand dollar robot arms, um, or seventy thousand dollar robot arms, right? Like that's the kind of, uh, money we need to spend to have these research platforms that we need to show our results and test our results. And, um, actually, um, yeah. So for example, um, one of the projects we have, um, is, uh, a mobile manipulator. Uh, so it's, uh, a robot arm on top of a mobile platform. Think of a Roomba with an arm, maybe just like way more expensive. It's more like,
[00:20:36] Russ Altman: A forty thousand dollar Roomba. I gotcha.
[00:20:39] Jeannette Bohg: At least. Yeah. So, um, think about that. And that project was really fun. It's like, uh, using this mobile manipulator to clean up your house. So it's, um, uh, it's basically talking to you to figure out like, oh, what are your preferences? Where are your dirty socks going? Where are your, you know, Coke cans, your empty Coke cans going? Um, and then it, uh, kind of from your few examples, compresses that down to like some general categories of where to put stuff.
[00:21:05] And so that's the robot we, uh, we did a project on, and people are very excited about it. They loved it. It's even throwing stuff into bins. It's like a basketball star in a way. Um, and people really love it. And also researcher loves it. Researchers loved it, because there's this mobile base. Uh, so the, basically the, um, you know, the thing on wheels, basically,
[00:21:29] Russ Altman: Yeah, it can move around.
[00:21:30] Jeannette Bohg: Um, that one, uh, is very unique. It was a donation from some company. Um, and it's, uh, it has like specific capabilities, but it's like three of a kind exist in the world and, um, we, and people can't buy it and it's very disappointing. So, um, but again, yeah, these are the arms that we are constantly, uh, constantly like repairing and it's like scary even because if we lose this platform, we can't do our research.
[00:21:58] Russ Altman: Right.
[00:21:58] Jeannette Bohg: So one of the things I'm doing for the first time in my lab, actually, and again, I'm a computer scientist, not a mechanical engineer. But, uh, with one of my students, we're looking at how to develop a low-cost version of this, uh, mobile base that has like these special abilities and is very maneuverable.
[00:22:17] Um, and I'm, my hope is that with this platform first, I hope it's reliable, but if not, at least you can like cheaply repair it, um, and can get in there, right? Like even if you're a student with, who is a computer scientist, not a mechanical engineer, and I hope that it just allows you to buy many of these platforms rather than just one, uh, you know, that you have to baby around all the time, but you can maybe hopefully buy many of them, you will hopefully open source all of this design.
[00:22:47] And then, uh, my, what I'm really excited about is to use this low-cost platform, um, to do maybe swarm based manipulation of many robots, uh, collaborating with each other.
[00:23:00] Russ Altman: So in your current view, what would be the basic functionality of one of these units or is that flexible? But is it a hand? Is it two hands? Is it, uh, is it mobile like a Roomba?
[00:23:12] Jeannette Bohg: Yeah, it's basically, uh, um, yeah, you could think of it as a Roomba plus plus basically, which has an arm. So it's not just like, uh, vacuum your floor, but it's actually putting things away. Right? Like if you, uh, for those who have children, right, like I, I think they are always most excited about, about this, what we call TidyBot, um, because it's just like putting things into the right places instead of you stepping on these Lego pieces in the middle of the night, right.
[00:23:39] So that's what you, um, that's what we're going for. Uh, and it would be one mobile base with one arm and one hand. And then let's say you have multiple of them. So, uh, for, you could, for example, think of when you have to move, right? Like I personally think moving to another place is, I mean, it's the worst, right?
[00:23:59] Russ Altman: Packing, packing and unpacking is the worst.
[00:24:01] Jeannette Bohg: Packing, unpacking, but also like carrying stuff around. So imagine if you have like this fleet of robots, right? That just helps you getting the sofa through like these tight spaces and all of this. So that's kind,
[00:24:11] Russ Altman: Paint a picture for me in this version one-point-oh, how tall is it? Are we talking two feet tall or five feet tall? How big is it?
[00:24:19] Jeannette Bohg: Now you're getting me with the feets and the inches.
[00:24:22] Russ Altman: I'm sorry. You can draw meters, whatever, whatever works.
[00:24:25] Jeannette Bohg: Okay. Yeah. So actually, uh, so the base is actually fairly, uh, low. Um, and actually pretty heavy so that it has like a low center of mass. It's probably like, I guess a foot tall. Um, I let's say twenty centimeters.
[00:24:39] Russ Altman: Yeah.
[00:24:39] Jeannette Bohg: Um, and then the arm, if it's fully stretched out and just pointing up, it is probably like one and a half meters long on top of that.
[00:24:48] Russ Altman: That's five feet or so.
[00:24:50] Jeannette Bohg: Really like fully stretched out, which it usually isn't to do stuff. It's like,
[00:24:54] Russ Altman: But then it could reach things on tables. That's the, that's what I was trying to get to. It could reach tables. It could maybe reach into the dryer or the washing machine or stuff like that. It might be within range.
[00:25:05] Jeannette Bohg: Uh, all of this then, uh, also just making your bed. Uh,
[00:25:09] Russ Altman: Yeah, I hate that.
[00:25:11] Jeannette Bohg: Yeah, terrible.
[00:25:11] Russ Altman: So let me ask, uh, since we're talking about what it looks like. Um, in so much of the sci fi, robots seem to have to look like humans. What's your take on that? Like, is it important that the robot, is it, maybe it's not, maybe it's important that it not look like a human, where are you in this whole humanoid debate?
[00:25:29] Jeannette Bohg: Okay, this is a very good question. And I'm probably going to say something contentious, uh, or maybe not, I don't know. But yeah, I think building a humanoid robot is really exciting from a research standpoint. Um, and I think it's just looks cool. So it gives you like these super cool demos that you see from all these startups right now,
[00:25:49] Russ Altman: Right, right.
[00:25:49] Jeannette Bohg: On Twitter and all. I mean, this looks very cool. I just personally, um, don't think that it's like the most, um, like economical way maybe to think, uh, about like, what's the most useful robot. I think the arguments are typically like, oh, but, um, the spaces that we walk in and work in and live in, they're all designed for people. So why not making a robot platform that is having the same form factor and can squeeze through tight places and use all the tools and all of that. It kind of makes sense to me.
[00:26:25] Um, but again, like coming back to my earlier point, right? Where I'm thinking like general purpose robots are really, really far away. Um, and I think the, um, like narrow, like the, uh, let's say closer future, not the future of everything, but the future in like the next few years. Uh, it's maybe, um, it's maybe going to look more at like very specific purpose robots that are maybe on wheels because that's just easier, right? Like you don't have to worry about this. Um, and they can do relatively specialized things in like one environment, like going to a grocery store and doing restocking, um, or things like that. Right? Um,
[00:27:04] Russ Altman: I've also heard that you have to be careful about making it humanoid because then humans might impute to it human capabilities, human emotions. And by having it look like a weird device, it reminds you that indeed this is a device and maybe the user interaction might be more natural and less misled because you start, you know, you don't treat it like it's a human and that might not be the goal. In other cases, like for care of the elderly, maybe you want it to look humanoid because it might be more natural. But okay, that's a very, very helpful answer.
[00:27:37] Jeannette Bohg: Yeah, I think this is a very good point, actually, that people probably attribute much more intelligence, uh, whatever, whatever way we want to define that to a humanoid robot rather than to something like TidyBot that we had, right? Which is just one arm. It really looks very robot, I have to say.
[00:27:55] Russ Altman: So what is the outlook to finish up in the last minute or so? Where are we with this platform? And when are you going to start shipping?
[00:28:04] Jeannette Bohg: We published this on Twitter basically. There were lots of people like how much money, like when can I buy this? And, uh, and yeah, again, like it's, we're pretty far away from having like a robot that we can just literally, uh, give you and then it's gonna work, right?
[00:28:19] Like, I think there's so much engineering. I think you can probably bring it up like similar to autonomous driving, right? Like fairly, maybe easily to ninety percent, but then the rest of it is all these corner cases, right? That you have to deal with and it's going to be really hard. So I don't want to make a prediction of when we're going to have this. Again, I think it's going to be more like more special purpose, uh, robots. Um, again, maybe a Roomba is maybe not so far away with an arm, right?
[00:28:48] Russ Altman: I love it. I love it. And I know that in the academic world, ninety percent and cheap will lead to a lot of innovation.
[00:28:56] Jeannette Bohg: Right. That's the other point, like, when is it affordable, right? Like nobody's going to buy a robot that is as much as a luxury car, right?
[00:29:04] Russ Altman: Right.
[00:29:05] Jeannette Bohg: That can't even do anything really well.
[00:29:07] Russ Altman: Right.
[00:29:08] Thanks to Jeannette Bohg. That was The Future of Robotics.
[00:29:11] Thanks for tuning into this episode too. With more than 250 episodes in our archives, you have instant access to a whole range of fascinating conversations with me and other people. If you're enjoying the show, please remember to consider sharing it with friends, family, and colleagues. Personal recommendations are the best way to spread the news about The Future of Everything. You can connect with me on X or Twitter, @RBAltman. And you can connect with Stanford Engineering @StanfordENG.
Related Departments
New stanford hydrogel to reduce damage of california wildfires.
- Media mentions
This breakthrough gel could help save homes during wildfire season

The future of Russia and Ukraine
Advances and perspectives in collaborative robotics: a review of key technologies and emerging trends
- Open access
- Published: 29 August 2023
- Volume 2 , article number 13 , ( 2023 )
Cite this article
You have full access to this open access article
- Swapnil Patil 1 ,
- V. Vasu 1 &
- K. V. S. Srinadh 1
6868 Accesses
6 Citations
Explore all metrics
This review paper provides a literature survey of collaborative robots, or cobots, and their use in various industries. Cobots have gained popularity due to their ability to work with humans in a safe manner. The paper covers different aspects of cobots, including their design, control strategies, safety features, and human–robot interaction. The paper starts with a brief history and evolution of cobots, followed by a review of different control strategies and Safety features such as collision detection and avoidance, and safety-rated sensors are also examined. Further to this, a systematic review of Ergonomics is also taken into account. Additionally, the paper explores the challenges and opportunities presented by cobot’s technology, including the need for standards and regulations, the impact on employment, and the potential benefits to industry. The latest research in human–robot interaction is also discussed. Finally, the paper highlights current limitations of cobot’s technology and the need for further research to address technical and ethical challenges. This synthesis document is an invaluable resource for both academics and professionals interested while developing and application of cobot’s technology.
Similar content being viewed by others
Collaborative Robots in the Critical Infrastructure Area Review
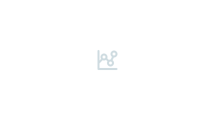
Collaborative Robotics: A Survey From Literature and Practitioners Perspectives
Intelligent and Collaborative Robots
Explore related subjects.
- Artificial Intelligence
Avoid common mistakes on your manuscript.
1 Introduction
Collaborative robots, commonly known as cobots, are transforming the way humans and robots collaborate in shared workspaces. The need for enhanced productivity and efficiency in industries, including manufacturing, logistics, and healthcare, has fuelled the development of cobots. Cobots are distinct from conventional industrial robots as they are intended to operate securely and efficiently in conjunction with human workers, providing greater flexibility and adaptability in the workplace.
One of the key challenges in developing collaborative robots is creating systems that can effectively perceive and respond to their environment. To address this challenge, researchers are exploring the utilization of computer vision and sensory modalities to boost the abilities of cobots in collaborative workspaces. Computer vision allows cobots to perceive their environment through visual data, while sensory modalities such as force-torque sensors and lidars provide additional feedback on the cobots’ movements and interactions with their environment.
To gain a better context and appreciate the importance of collaborative robots, it is crucial to comprehend industrial robots. Industrial robots are programmable and autonomous machines comprising electronic, electrical, and mechanical components, capable of executing a complex set of operations. These robots are massive, inflexible, and are usually installed to perform dangerous and physically demanding tasks that may be hazardous for human, such as transporting heavy loads in factories. Generally, industrials robots are designed for specific applications, kept separate from human workers, and occupy a distinct workspace. In contrast, collaborative robots, also known as co-bots, are intended to operate alongside human workers in the same workspace. These robots weigh a lot less compared to traditional industrial robots, enabling greater mobility and ease of movement within the factory or workspace or industry that they are installed in. One of the advantages that cobots offer over industrial robots is their flexibility, as they can be used to perform multiple tasks, making them highly adaptable to changing work requirements.
This review's objective is to give sufficient information on the state of the art for HRI in artificial cobotic fields. A collaborative system is created to conduct business with a living being within a predetermined collaborative workspace where mechanical hazards are most likely to arise. This is because when humans and robots share a workplace, it is feasible for implicit, non-functional (and undesirable) linkages to form. While collaborative robots offer several crucial safety precautions that permit the execution of safe operations, this status typically changes as they are incorporated into a working environment and outfitted with various end-effectors kinds. Because of this, it's important to properly enforce safety rules regarding the design of the work cell as well as devices for preventing collisions and/or contact mitigation.
The psychophysical and social well-being of drivers is a part of ergonomics, often known as human factors. Physically speaking, collaborative robots can lighten the pressure on drivers by helping them with laborious and repetitive duties. As opposed to that, a close partnership can stress out drivers' brains. In fact, the unidentified robot movements may hurt drivers' abilities and performances.
Because of this, cognitive ergonomics in collaborative robots is a genuinely new and sometimes overlooked concept. Drivers may experience mental stress due to teamwork. In fact, the unidentified robot movements may hurt drivers' abilities and performances. Because of this, cognitive ergonomics in collaborative robots is a genuinely new and sometimes overlooked concept. So as to move collaborative robotics from the laboratory to the workshop or manufacturing facility of the industry, the purpose of this study is to examine the state-of-the-art in collaborative robotics safety and ergonomics and to pinpoint those research areas that are very significant (Fig. 1 ).
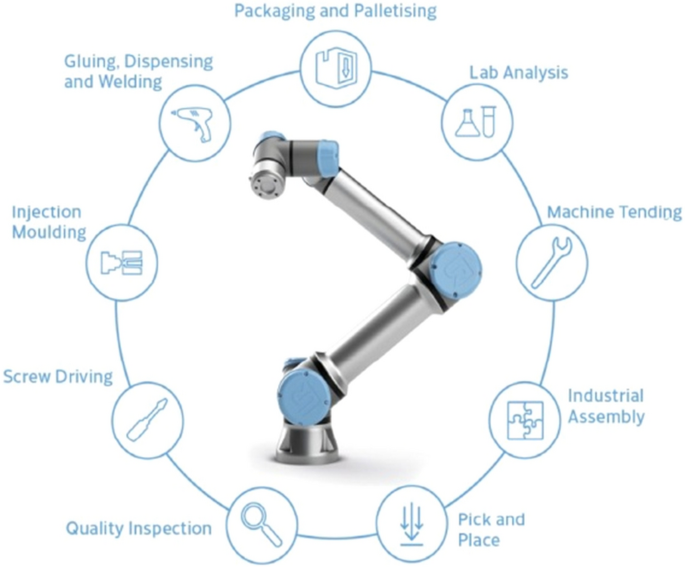
Application of COBOTS
1.1 History of collaborative robots
The history of industrial revolutions sheds light on where collaborative robots stand in terms of industrial technological advancements. Industrial revolutions are defined as changes in technology used in manufacturing and production industries during a specific time period. The seventeenth century saw the beginning of the industrial revolution, saw the introduction of water and steam power to mechanize machines, which revolutionized manufacturing and allowed for mass production and assembly lines. The second industrial revolution, in the late eighteenth century added electricity to the equation and replaced steam engines with electrical ones. The third industrial revolution, in the late nineteenth century, saw the introduction of computers and automated machines, leading to further automation and increased manufacturing and assembly line capacities with increased productivity.
Industry 4.0, the latest and most advanced concept of industrial revolution, was coined in Germany in 2011. Industry 4.0 uses digitization and networked production, incorporating IoT, cyber-physical systems, and cloud computing to create “Smart Factories.” Although the concept of collaborative robots predates Industry 4.0, they have become increasingly relevant to the production and manufacturing industry with the advent of this latest revolution. In shared workspaces, collaborative robots are made to function effectively and safely next to human workers. They are programmed to perform a range of tasks, such as assembly, welding, packaging, and inspection, among others. Cobots have a range of sensors on board and technologies that allow them to detect and avoid collisions with human workers and adjust their movements based on human input (Figs. 2 , 3 ).
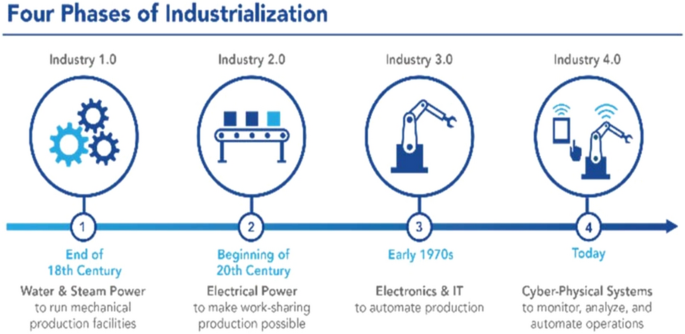
History of COBOTS
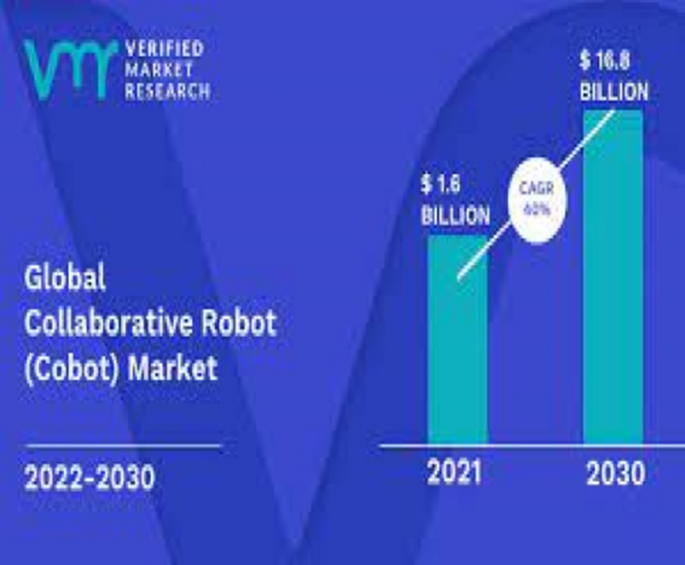
Market of COBOT
1.2 Difference between robots and cobots
See Table 1
1.3 Types of cobots
Independent
Robots that can work independently and collaboratively with humans are created for different manufacturing processes and on different job items. So as to make sure that the cobots can operate securely and effectively without the need for cages or fences, this sort of collaboration often uses sensors and other safety elements. Robots that can work independently and collaboratively with humans are created for different manufacturing processes and on different job items.
So as to make sure that the cobots can operate securely and effectively without the need for cages or fences, this sort of collaboration often uses sensors and other safety elements.
Simultaneous
A human operator and a collaborative robot (cobot) work simultaneously on different production processes at the same work piece in simultaneous collaborative robots. There is no task or time dependency in this kind of collaboration between humans and cobots. Concurrently working on the same piece of work reduces transit time and boosts productivity and space efficiency.
By allowing cobot to carry out potentially hazardous duties for a human operator, simultaneous collaboration can also increase safety in dangerous circumstances.
Sequential collaborative robots are used to undertake successive production procedures on the same work item with a human operator. The operator’s operations and those of the cobots are time-dependent, with the cobot being tasked with handling more time-consuming or repetitive activities, which may also improve the operator's working conditions.
This kind of cooperation is beneficial for boosting output, cutting down on errors, and cutting down on idle time in between activities. Working together sequentially is frequently employed in processes like assembly, welding, and material handling.
Supportive collaborative robots are a subset of collaborative robots that allow an operator and cobots to collaborate while working on the same task or piece of work. As one cannot complete the task without the other, there may be complete dependencies between the human and the cobots in this type of situation. Together, the cobot and human operator strive to accomplish a single objective, each balancing the other's advantages and disadvantages.
Some common applications of supportive collaborative robots include assembly tasks, pick-and-place operations, and quality control inspection.
2 Literature survey
This section offers a survey of latest studies on interactions between human and robots in commercial collaboration robotics. Additionally, it suggests dividing the information in these works’ content into two groups: Safety and Ergonomics. The Safety category includes works focused on developing safe human–robot interaction systems and ensuring the safety of human workers in shared workspaces. The Ergonomics category includes works focused on improving the ergonomic design of collaborative robots to enhance the comfort and efficiency of human workers. Furthermore, this chapter addresses the challenges associated with industrial Cobots and identifies potential areas for future research.
One major challenge is the development of effective communication systems that enable seamless cooperation between machines and people. Additionally, there is a need for the development of advanced sensing technologies that enable robots to perceive and respond to their environment in real-time.
In conclusion, this chapter highlights the emergence of collaborative robots and the need for new human–robot interaction systems to fully utilize their capabilities. It also provides a classification of recent works in the field and addresses challenges and future research directions.
Materials and methods
There are several papers and journals and hence to take the most relevant into account review should be carried out using systematic, scientific, and transparent and in reliable method.
To carry out scientific review on Collaborative Robotics we followed following steps for study:
Step 1: defining the study's or reviews scientific objectives;
Step 2: defining the explorations amorphous borders;
Step 3: setting the conditions for data collection;
Step 4: validation of result and classification.
Defining the study’s or reviews scientific objectives
The following research questions allowed us to determine the study's goals:
RQ1. What are the main research themes or areas of research in collaborative robots?
RQ2. Classification of research themes and identifying the most prominent out of Safety and Ergonomics.
RQ3. What are the research gaps and research challenges?
In the most recent scientific literature, researchers have mostly focused on safety and ergonomics (or human aspects) for cobots intended for industrial usage. This review study will assist us in understanding and examining the most recent research issues and areas in safe and comfortable collaborative robotics. To use collaborative and participatory workplaces successfully in assiduity, we specifically want to comprehend how the exploratory results obtained in recent times can be dispersed and where we need to focus in the future.
Defining the exploration's amorphous borders
When reviewing literature on cobots, it is important to establish conceptual boundaries to ensure that the review is focused and relevant to the research question or topic at hand. Some possible conceptual boundaries to consider include:
Type of collaboration: collaborative robots can interact with humans in a variety of ways viz Supportive, Sequential, Simultaneous or Independent. Researchers may choose to focus on a particular type of collaboration to better understand the specific issues related to that type of interaction.
Safety and ergonomics: safety and ergonomics are critical considerations in the design and implementation of collaborative robots. Researchers may choose to focus specifically on these aspects of collaborative robot research understand the latest state of the art and identify areas for improvement.
Technical approaches: collaborative robot research can involve a range of technical approaches, including control algorithms, sensing technologies, and human–robot interface design. By focusing on a specific technical approach, researchers can gain a deeper understanding of the strengths and limitations of that approach and identify areas for future research and development.
Human factors: collaborative robots are designed to work alongside humans, and as such, understanding human factors is essential to their successful implementation. Researchers may choose to focus specifically on human factors research related to collaborative robots, such as user acceptance and trust workload and cognitive demands, and the impact of robot behavior on human performance.
These are just a few possible conceptual boundaries to consider when reviewing literature on collaborative robots.
Setting the conditions for data collection
We linked pertinent documents for our investigation in several ways.
As a preliminary step, we used the following search phrases for the title, abstract, and keywords to link the literature in the collaborative robotics field: Cobots, collaborative robots, human-robots, collaborative robotics, etc. All types of topics and documents were included in this initial step.
The terms “industry,” “artificial,” “manufacturing,” “assembly,” and “product” were included to the hunt terms in this alternative stage because we focused on collaborative robotics in the artificial sector as robotic results.
The following stage was to concentrate our investigation on pertinent engineering or computer science exploration studies.
The search was limited to using the journal as a source in order to solely examine high caliber content. To concentrate the research on problems related to the design of collaborative workplaces, we further limited the search to the topic categories “Engineering” and “Computer Science.”
In the fourth step, we split the results of the hunt into two groups, one for a workshop discussing safety and the other group discussing ergonomics. As a result, we divided the data into two groups, one using the phrase “safety” and the other using “ergonomics” or “human factors”.
Validation of result and classification
As per research questions, our prime objective are to segregate the state of the art literature of collaborative robotics into different clusters and sub clusters and identifying the most prominent cluster.
In this stage, initially thorough reading of abstracts of each literature is conducted to identify the relevance of literature to our study. In consequent steps, proof reading to journals and papers were done and certain literature were eliminated which were not relevant to the objectives of study.
In next section, Discussion of the content of the scientific literature on collaborative robots is elaborated which broadly classified into two groups viz. Safety and Ergonomics. Further each group is classified into two sub clusters (Fig. 4 ).
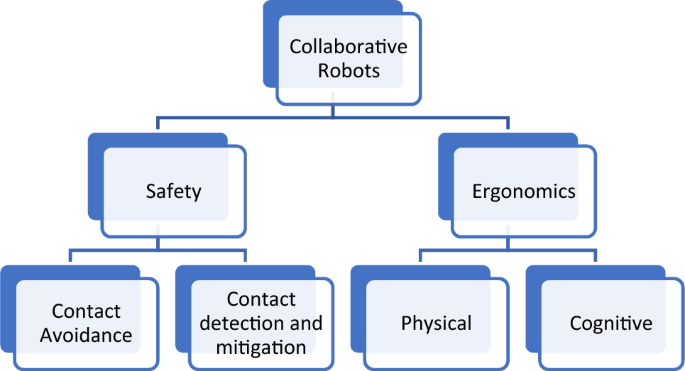
Identification of groups and subgroups
“It’s impossible for a robot to hurt a human being” and “A robot cannot, through its inaction, enable a human being to endanger himself” are the first and third laws of robotics, respectively. This emphasizes how crucial safety is with the evolution of industrial cobots, robots are now capable of working alongside humans and performing tasks in close proximity. However, doing so necessitates disregarding security protocols and eliminating human–robot physical separation. The fast movement and use of dangerous tools by robots can pose a threat to humans. Moreover, in extreme environmental conditions or in case of system failure, the dangerous behavior of these systems can lead to catastrophic consequences.
Authored by De Santis and Siciliano’s the paper provides a comprehensive review of safety issues related to human–robot cooperation in manufacturing systems. The authors identify four main categories of safety issues: physical safety, functional safety, social safety, and psycho safety. One of the key contributions of the paper is its emphasis on the need for a comprehensive, multidisciplinary approach to addressing safety issues in human–robot collaboration [ 1 ].
Bicchi et al. discuss the safety issues related to the increasing trend of physical interaction between humans and robots. Author proposes the concept of “safe robot behavior” which is based on the robot's ability to sense the environment, monitor its own actions, and adjust them to ensure safety. The authors further discuss the various factors that influence the safety of physical human–robot interaction, such as the level of interaction, the type of task, the environment, and the human operator's experience and skills. The chapter concludes by presenting various approaches and technologies that can be used to enhance the safety of physical human–robot interaction, such as compliance control, force feedback, and proximity sensors. The authors underline the importance of further research and development in this field to ensure safe and effective human–robot collaboration [ 2 ].
Wang et al. provided a brief review of safety strategies for physical human–robot interaction (PHRI). They highlighted the importance of developing safety measures to ensure that PHRI can be integrated safely into various applications, including manufacturing, healthcare, and home assistance. The authors identified various types of safety strategies, including mechanical, electrical, and software-based measures. They also discussed the importance of integrating sensing and monitoring systems into PHRI applications to detect and react to any potential collisions or hazards [ 3 ].
The International Organization for Standardization (ISO) published a technical study called ISO 10218-2:2011 that details the safety standards for industrial robots and robotic devices, specifically the robot systems and integration. This standard offers instructions for designing, installing, running, and maintaining robotic systems, together with the necessary safety precautions for human–robot interaction (HRI). The report includes provisions for both functional and environmental safety, including protection against electric shock, fire, and explosion, and requirements for emergency stop functions, protective barriers, and safety interlocks. The standard also provides guidelines for risk assessment and reduction, as well as for the design and verification of safety-related control systems [ 4 ].
The paper by Gualtieri et al. discusses how to create collaborative assembly workstations that are both safe and ergonomic while also meeting the demands of production efficiency; system integrator designers need new design criteria. In this article, design rules and prerequisites are collected and categorised based on international standards, research, and actual use cases. This effort will aid in the future creation of a simple technique for the assessment of both new design concepts and applications based on the fulfilment of several criteria listed in a tick list. From the perspective of occupational health and safety, this check list will also give a preliminary assessment of how well certain of the required Machinery Directive standards have been met [ 5 ].
Further, safety of cobotics system in divided into two broad categories.
2.1.1 Contact avoidance
The idea of Contact Avoidance focuses on preemptively addressing the mechanical risks to operators by implementing preventive methods and systems to avoid hazardous contact. The ultimate goal is to prioritize the safety of the operators in industrial settings where they are working alongside machinery and equipment.
Schmidt and Wang et al. proposed a novel approach for active collision avoidance in human–robot collaboration scenarios. Their work focused on the development of a collision detection and avoidance system that uses force feedback to adjust the robot's trajectory in real-time. The proposed system consisted of three main components: a force sensor, a control unit, and a collision avoidance algorithm [ 6 ].
Chablat and Girin et al. developed an industrial security system that ensures safe and secure human–robot coexistence in manufacturing environments. The authors proposed an industrial security system that combines a range of sensing technologies, including cameras, laser scanners, and pressure sensors. The system is designed to detect the presence of humans in the robot's workspace and respond accordingly [ 7 ].
Bedolla and Belingardi et al. addressed the challenge of developing safe and efficient human–robot collaboration (HRC) assembly process in the automotive industry. The authors proposed a safety design framework that consists of three main phases: risk assessment, safety design, and safety verification. The risk assessment phase involves identifying and evaluating the risks associated with the HRC assembly process, such as collisions or entrapment. The safety design phase involves developing safety measures and controls to mitigate the identified risks, such as force-limited operation or proximity sensors. The safety verification phase involves testing and validating the effectiveness of the safety measures and controls [ 8 ].
Authored by Chen et al. the paper presents an approach to object recognition within the context of human–robot shared workspace collaboration. They propose a new approach based on deep learning algorithms, which can automatically learn and recognize objects in real-time [ 9 ].
The paper by De Luca and Flacco et al. discusses an integrated control approach for PHRI. The authors emphasize the need for effective collision avoidance, detection, reaction, and collaboration in order to ensure safety during human–robot interaction. The proposed control approach is based on a combination of active and passive compliance control. The authors also describe the use of vision and force feedback sensors to improve situational awareness and to enable the robot to adapt its behavior in real-time based on the human’s actions [ 10 ].
Fiacco et al. presented a depth space approach for evaluating the distance to objects in a human–robot collaborative workspace. The “Depth space,” is a metric space that represents the distances between the robot and objects in the workspace. They proposed a method for computing depth space using RGB-D data and a mathematical formulation that allows the robot to assess the distance to objects in real-time. The study contributes to the literature on human–robot collaboration by proposing a new approach for evaluating distance to objects in the workspace, which can aid in collision avoidance and ensure the safety of humans and robots working together [ 11 ].
Authored by Navarro et al. paper presents a novel approach for achieving safe human–robot interaction based on adaptive damping control. The authors propose an ISO10218-compliant controller for robotic manipulators, which is capable of reducing the damping coefficient during the interaction with a human operator to minimize the risk of injury in the event of a collision. The controller estimates the external force applied by the human operator and adapts the robot's damping coefficient accordingly to limit the collision force. The authors evaluate the performance of the proposed controller using a KUKA robot arm and a force/torque sensor [ 12 ].
Authored by Morato et al. proposed a framework for safe human–robot collaboration using multiple kinects for real-time human tracking. The proposed framework integrated the RGB and depth information of multiple Kinects to create a 3D model of the workspace and the humans present in it. The 3D model was then used to track the human movements in real-time, and the robot was programmed to respond accordingly. The study suggests that the use of multiple kinects for real-time human tracking can significantly improve the safety of human–robot collaboration. However, the study did not address the limitations of the kinect technology, such as occlusions, accuracy issues, and noise in the depth data, which could affect the reliability of the proposed framework in practical settings [ 13 ].
Authored by Avanzini et al. proposed a novel approach for safety control of industrial robots using a distributed distance sensor. The proposed solution involved the use of a network of sensors that can detect the proximity of any obstacle or person within the robot workspace. The system was designed to operate in real-time and provide continuous feedback to the robot controller, allowing it to adapt its movements and speed to avoid any potential collision. The results showed that the distributed distance sensor was able to detect obstacles accurately and provide timely feedback to the robot controller, allowing it to modify its movements and avoid collisions [ 14 ].
Authored by Bdiwi et al. presents a strategy for ensuring the safety of human–robot interaction in industrial settings. The proposed strategy involves dividing the interaction between the human and the robot into three levels: low, medium, and high. For each level, the authors propose specific safety measures that should be implemented to ensure the safety of humans during the interaction. These measures include limiting the speed and force of the robot, using proximity sensors to detect the presence of humans, and implementing emergency stop systems [ 15 ].
2.1.2 Contact detection and mitigation
The idea of Contact Detection and Mitigation is focused on ensuring the safety of operators in terms of mechanical risk by reducing the energy exchanged during unexpected or accidental contact between humans and robots. This is accomplished through the implementation of systems and methodologies aimed at detecting and mitigating such collisions.
Authored by Heo and Lee et al. the paper proposes a deep learning-based approach to collision detection for industrial collaborative robots. The authors propose a deep learning-based approach that uses convolutional neural networks (CNNs) to predict collisions between the robot and its environment. They train the CNN on a dataset of simulated collision scenarios, and demonstrate that the model can accurately predict collisions in real-time with low computational overhead [ 16 ].
Authored by Wang et al. the paper presents an overview of the state-of-the-art technologies and approaches for implementing physical human–robot interaction (pHRI) such as force sensing, tactile sensing, and vision-based sensing in collaborative manufacturing systems. To evaluate the effectiveness of pHRI in manufacturing, the authors conducted a case study involving a collaborative assembly task. The study involved the use of a force-sensing and camera-sensing robot to work alongside human workers in the assembly of a product [ 17 ].
Authored by Liu et al. the paper presents a collision detection and identification method for robot manipulators based on an extended state observer (ESO). The authors propose a collision detection and identification method based on an ESO. The ESO is used to estimate the state of the robot manipulator, including the position, velocity, and acceleration. By comparing the estimated state with the expected state, the method is able to detect and identify collisions [ 18 ].
The paper by Schiavi et al. discusses the integration of active and passive compliance control for ensuring safe human–robot coexistence. The authors argue that active compliance control can ensure safety during interactions with high forces or impacts, while passive compliance control can provide stability and safety during interactions with low forces or impacts and presents a hybrid controller that combines both active and passive compliance control and allows for safe interaction with a human operator [ 19 ].
Authored by De Luca et al. the paper focuses on the development of a lightweight manipulator arm equipped with sensors to detect potential collisions and to react appropriately to prevent damage to the robot and injury to humans. The paper describes the collision detection system which is based on a combination of force and torque sensors, and visual information from cameras mounted on the robot. The authors also propose a safe reaction algorithm to avoid or minimize the impact of collisions [ 20 ].
Authored by Haddadin et al. the paper provides an in-depth review of the collision detection and reaction approaches for ensuring safe physical human–robot interaction. The authors present a novel approach for collision detection and reaction using three-layer safety architecture. The first layer is the control layer, which monitors the robot's motion and signals an alarm in the event of a collision. The second layer is the decision layer, which evaluates the severity of the collision and triggers the appropriate safety measure. The third layer is the reaction layer, which executes the safety measure and stops the robot in case of an emergency [ 21 ].
Authored by De Benedictis et al. proposed a control strategy for regulating force impulses during human–robot interactions. The authors proposed a control strategy based on impedance control, which uses a combination of force and position control to regulate the force impulse during an impact. The proposed method was implemented and tested using a robotic manipulator and a force sensor. The results showed that the proposed strategy effectively regulated the force impulse during impact, leading to improved safety during human–robot interactions [ 22 ].
The paper by Indri et al. presents a collision detection method between an industrial robot and its environment. The approach consists of three main steps: first, the environment is modeled using a mesh structure; second, the robot is represented as a set of convex polyhedral; and finally, collision detection is performed using an efficient algorithm that takes into account the relative motion between the robot and the environment. Experimental results demonstrate that the effectiveness of the proposed approach and on comparison approach found to be more efficient [ 23 ].
Authored by Lee and Song et al. the paper proposed a novel method for detecting collisions between a robot manipulator and its surroundings without the need for sensors. The proposed method utilizes a friction model to estimate the contact force between the robot manipulator and the environment. This force is then used to detect collisions based on a threshold value set by the user. The authors tested the method on a three-axis robot arm and showed that it was able to successfully detect collisions with high accuracy and without the need for additional sensors [ 24 ].
Authored by Ren and Dong et al. presented a new approach for collision detection and identification of robot manipulators based on an extended state observer (ESO). The proposed method used the ESO to estimate the external disturbance caused by the collision and identified the collision parameters, including the collision position, direction, and magnitude. The main contribution of this work is the use of an ESO for collision detection and identification of robot manipulators [ 25 ].
2.2 Ergonomics
Ergonomics is the study of designing work environments and systems that are optimized for human use. Collaborative robots are designed to work safely and effectively with human workers in a shared workspace. Therefore, ergonomics is essential in the design and implementation of collaborative robots for several reasons such as Safety, Efficiency, Comfort, Productivity and Adaptability. Overall, the importance of ergonomics in collaborative robots cannot be overstated and leads to better outcomes for both human and robot workers.
Bortot’s et al. focuses on the ergonomic aspects of human–robot coexistence in the context of production. The thesis identifies several key ergonomic factors that are important for ensuring safe and effective human–robot collaboration in production settings. These include physical factors such as the design and placement of robotic systems, as well as cognitive and social factors such as the level of automation and the quality of communication between humans and robots [ 26 ].
Authored by Fraboni et al. focus of this article is on establishing secure and productive human–robot collaborations, which help us, understand how to implement and evaluate collaborative robotic systems in organizations. This means that the interaction between people and cobots should be planned and carried out in a way that minimizes hazards to employees while still increasing system performance and productivity. In general, successful human–robot collaboration entails finding a balance between protecting workers’ physical and mental health and reaching the appropriate levels of productivity and performance. The study emphasizes crucial tactics for assuring employees' psychological well-being, maximizing performance, and fostering the seamless integration of new technology. This has broad implications for sustainability in organizations [ 27 ].
2.2.1 Physical ergonomics
Physical Ergonomics in the field of human–robot interaction in industrial settings involves reducing biomechanical workload through the use of collaborative robots as advanced tools, aimed at improving the physical well-being of the operators.
Authored by Sadrfaridpour and Wang et al. proposes an integrated framework for HRI in collaborative assembly tasks within Hybrid manufacturing cells which consists of three key components: task planning, motion planning, and control. The task planning involves determining the optimal sequence of tasks for the human and robot, taking into account factors such as task complexity and worker/robot capabilities. The motion planning involves generating trajectories for the robot and human worker to perform their respective tasks, while ensuring that collisions are avoided and the task is completed efficiently. The control involves implementing feedback control to ensure that the robot and human worker perform their tasks accurately and effectively [ 28 ].
Authored by Cherubini et al. the paper presents a framework for collaborative manufacturing pHRI which consists of three main components: task planning, pHRI control, and safety monitoring. The task planning involves determining the optimal sequence of tasks for the human worker and robot to perform, taking into account factors such as task complexity and worker/robot capabilities. The pHRI control involves implementing feedback control to ensure that the robot and human worker perform their tasks accurately and effectively, while ensuring that the human worker is not at risk of injury. The safety monitoring involves continuously monitoring the environment and behavior of the human worker and robot to ensure that any potential safety risks are identified and mitigated [ 29 ].
Authored by Dannapfel et al. the paper presents a systematic planning approach for enabling heavy-duty human–robot cooperation in the automotive flow assembly process which consists of five main steps: (1) process analysis and classification, (2) task allocation, (3) workspace design, (4) robot selection, and (5) safety analysis [ 30 ].
The Robonaut is a humanoid robot designed for working in space environments with astronauts. Bluethmann et al. present the development of Robonaut and its potential applications in space missions. The robot’s design includes human-like arms, hands, and fingers that can mimic human movements and perform complex tasks. The robot is also equipped with sensors, cameras, and computer vision systems that allow it to interact with its environment and perform various tasks. The paper discusses the design challenges associated with creating a humanoid robot for space missions, including the need to ensure safety, reliability, and compatibility with the existing space infrastructure [ 31 ].
Authored by Müller et al. investigates how collaborative robots (cobots) can be integrated into assembly lines and how they can work together with human workers to increase efficiency and productivity. The authors analyzed the assembly tasks and identified those that could be performed by robots and those that required human involvement. The study proposed a process-oriented task assignment algorithm to determine what tasks are expected to the robot and what tasks are expected by the human worker. The algorithm takes into account the complexity of the task, the skill level of the worker, and the robot's capabilities [ 32 ].
Maurice et al. present a literature review on human-oriented design for collaborative robots. They begin by defining the characteristics of cobots and highlighting the challenges involved in designing them. They then discuss the various design considerations that must be taken into account when creating cobots that are safe and easy to use. These include the robot's size, weight, speed, and mobility, as well as its sensing and control capabilities. The authors then discuss several case studies that illustrate how human-oriented design can be applied in practice. They also discuss the use of motion capture technology to develop cobots that can mimic human movements and collaborate with workers in real-time [ 33 ].
Authored by Heydaryan et al. the article discusses implementation of a HRC system in an automotive assembly line. The authors present the safety measures adopted for the system design and development to ensure the protection of the human operator during the collaboration process. It then introduces the case study of a real-world HRC assembly process in the automotive industry, and the safety design strategies and tools applied during the development of the system [ 8 ].
The paper by Tang and Webb et al. paper presents a gesture control system that allows operators to control robots without physically touching any interface. The authors suggest that this system may improve ergonomics and reduce the risk of repetitive strain injuries. The authors describe the design of their system, which is based on a combination of depth cameras and machine learning algorithms. The system uses the cameras to capture and interpret the operator's gestures in real time, and then uses this information to control the robot’s movements [ 34 ].
Authored by Faber et al. article presents a method for generating assembly plans that take into account the cognitive capabilities of human workers and the physical capabilities of robotic collaborators. The authors propose a planning framework that incorporates information about the tasks to be performed, the characteristics of the human workers, and the capabilities of the robots, with the aim of creating assembly sequences that are both ergonomic and efficient [ 35 ].
2.2.2 Cognitive ergonomics
Cognitive ergonomics pertains to minimizing mental stress and psychological discomfort for operators while working alongside robots. This principle is essential in ensuring interaction acceptability. Additionally, physical ergonomics focuses on reducing biomechanical workload and improving operators' physical well-being by utilizing collaborative robots as advanced tools. Organizational ergonomics, on the other hand, aims to optimize social-technical systems in terms of organizational structures, policies, and processes. By improving these factors, organizations can facilitate safe and efficient collaboration between human workers and robots.
Authored by Long et al. the paper presents an industrial security system designed to ensure safe human–robot coexistence in an industrial environment. The authors propose an industrial security system that includes three main components: a secure communication protocol, a secure operating system, and a secure monitoring system. The secure communication protocol is designed to prevent unauthorized access to the robot system by using encryption and authentication mechanisms. The secure operating system is designed to prevent malware and other attacks on the robot by enforcing strict security policies and isolating the robot's software environment from other systems. The secure monitoring system is designed to detect and respond to security breaches in real-time by analyzing system logs and monitoring the behavior of the robot and human operators [ 7 ].
Authored by Faber et al. the paper presents an approach to enhance human–robot collaboration in self-optimizing assembly cells by incorporating cognition into assembly sequence planning. The author proposes a cognition-enhanced assembly sequence planning approach that incorporates cognitive models of human behavior into the planning process. The approach uses a cognitive architecture called ACT-R (Adaptive Control of Thought-Rational) to model human behavior and simulate the performance of assembly tasks in collaboration with robots [ 35 ].
Solvang and Sziebig’s et al. paper presents a review of the literature on the use of industrial robots in cognitive info-communication. The paper explores the potential for robots to function as cognitive systems that can interact with humans in complex manufacturing environments. The author explains the concept of cognitive info-communication, which refers to the exchange of information and knowledge between humans and machines. They argue that cognitive info-communication is critical for effective human–robot collaboration in manufacturing, as it enables robots to understand and respond to human intentions and goals [ 36 ].
Authored by Shravani and Rao et al. discusses the challenges faced by industries while introducing robots and automation without creating fear of unemployment and high costs. The review included studies on the social and psychological impact of automation on the workforce and the economy. The framework also emphasizes the need for creating a supportive work environment that encourages human–robot collaboration and facilitates the transition to a more automated workplace [ 37 ].
Authored by De Santis’ et al. the paper presents literature review focuses on the modeling and control of physical and cognitive aspects in human–robot interaction (HRI). The paper explores the current state of HRI research and the challenges faced in modeling and controlling robot behavior in physical and cognitive aspects to improve human–robot collaboration. The author discusses the need for robots to have cognitive capabilities to facilitate communication and collaboration with humans in different environments. The review emphasizes the importance of designing robots that can adapt to different tasks and environments while ensuring the safety and comfort of humans [ 38 ].
Authored by Medina, Lorenz, and Hirche et al. the paper proposes a new approach to human–robot collaboration based on anticipatory haptic assistance. The paper presents a framework for human–robot collaboration that incorporates anticipatory haptic assistance, based on a stochastic model of human behavior. The authors then describe how this framework can be used to synthesize appropriate haptic cues that help guide the human operator towards a desired task outcome [ 39 ].
Authored by Matsas et al. presents a prototyping approach for proactive and adaptive techniques for human–robot collaboration in manufacturing using virtual reality. The authors propose a methodology for designing and evaluating human–robot collaborative tasks that integrates the use of virtual reality simulations and machine learning techniques. The paper focuses on the development of a proactive and adaptive approach to haptic feedback for collaborative tasks, which takes into account the uncertainty in human behavior [ 40 ].
Authored by Maurtua et al. discusses the key issues and challenges of human–robot collaboration in industrial settings, with a focus on safety, interaction, and trust. The authors provide an overview of various safety measures that can be taken to ensure safe HRC, including safety sensors and safety controllers. They also discuss the importance of communication between humans and robots, highlighting the need for robots to be able to understand human intentions and for humans to trust the robot's actions [ 41 ].
Authored by Charalambous et al. aimed to identify the key organizational human factors that influence the introduction of human–robot collaboration (HRC) in industry. The study involved semi-structured interviews with industry experts who had experience in HRC implementation… These factors included organizational culture, management support, employee involvement and training, job design, and communication. The authors noted that these factors were interrelated and had an impact on each other [ 42 ].
Authored by Rahman and Wang et al. proposes a framework for subtask allocation in human–robot collaboration based on mutual trust. The proposed framework is composed of three primary modules: the communication, the trust evaluation, and the subtask allocation. The authors validate their framework through simulation and experiments on a lightweight assembly task. The findings indicate that the proposed framework leads to enhanced collaboration performance and increased mutual trust between the human and robot [ 43 ].
Authored by Koppenborg et al. investigation into how human–robot collaboration in an industrial setting is impacted by movement speed and predictability. The author conducted a study with 32 participants who were instructed to put something together collaboratively with a robot. The authors concluded that movement speed and predictability are important factors to consider when designing human–robot collaboration systems in industrial settings, and that slower and more predictable robot movement can improve performance and perceived safety and trust [ 44 ].
3 Discussion
In this section, we will begin by presenting and analyzing the descriptive findings of our study. We will then proceed to examine the results derived from the content analysis, aiming to identify the most prominent research themes that have emerged within the field of safety and ergonomics in industrial collaborative robotics. Lastly, we will acknowledge and discuss the limitations associated with this study.
In total, 45 papers were analyzed in detail, with the following breakdown for each cluster, the total number of publications classified (including papers classified in additional clusters):
For Contact Avoidance 10 papers, 10 papers for Contact Mitigation and, 4 papers covering both contact avoidance and detection and mitigation of contacts.
One paper for Physical and Cognitive and Organizational Ergonomics, nine papers for Physical Ergonomics and, 11 papers for Cognitive and Organizational Ergonomics.
According to Fig. 5 , 53.33% of the publications found are about "safety," while 46.66% are about "ergonomics." This indicates that contemporary researchers made investments in. More work should be put into developing safety measures rather than researching HRI ergonomics situations.
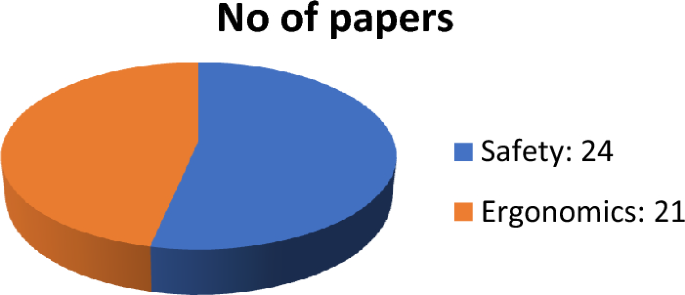
Number of papers
3.1 Challenges and future development
In this segment, Based on the most important and intriguing research themes found in each cluster, we highlight the limitations of our analysis and suggest options for future research.
3.1.1 Safety
Regarding safety in Human–Robot commerce (HRI), the primary ideal is to guard drivers from unlooked-for collisions between mortal body corridor and robot systems or workspace rudiments, while contemporaneously icing optimal performance of product systems.
Contact Avoidance crucial exploration themes that hold significance and pledge for Contact Avoidance includes Motion Planning and Control, Sensor Systems for Object Tracking, and Safety Management. These findings affirm the prevailing trend of developing safety systems that prioritize driver protection through preventative ways. Accordingly, a coordinated integration of vision systems, robot control, and line planning methodologies becomes pivotal. Safety Management also assumes significance as it supports the operation and evaluation of proposed safety measures, enabling better collision vaticination and minimizing the liability of similar incidents.
Contact Discovery and Mitigation Notable exploration themes for Contact Discovery and Mitigation comprise Motion Planning and Control, Robot System Design, and detector systems for contact operation. These themes parade essential correlations as advancements in protection- grounded safety measures bear concurrent development in robot tackle, contact discovery detector systems, and line planning. Similar combined sweats grease effective collision operation and reduce associated consequences.
3.1.2 Ergonomics
The part of ergonomics in HRI involves aiding humans in reducing biomechanical and cognitive load associated with work, without introducing new health and safety hazards stemming from relations with robot systems.
Fitness Ergonomics Task Scheduling (of high significance) and Motion Planning and Control (of moderate significance) are two important investigation subjects for physical ergonomics. These findings are consistent with the idea of human-centered workspaces supported by sophisticated robotization technologies. The creation of adaptive real-time task scheduling, as well as motion planning and control, should be given priority by unborn exploration. By adapting work cycles and robot system performance based on drivers' physical conditions (e.g. anthropometric features, age, gender, dominant branch, special limits or disabilities, weariness, etc.), these developments would facilitate workload reduction. Such a strategy aids in the implementation of sustainable product systems, improves the welfare of drivers, and makes it possible to hire older or otherwise disadvantaged workers. Still, it calls for the gathering of specific real-time data relating to drivers' psychophysical states.
Metrics and Tests, Motion Planning and Control, and Simulation and Modeling are some of the main research areas in cognitive ergonomics. Cognitive aspects should concentrate on minimizing work- related psychosocial pitfalls arising from participated conditioning and workspaces. Also, icing the adequacy of robot systems by mortal associates is pivotal. Balancing the advantages and implicit discomfort associated with varying degrees of commerce becomes essential. Methodologies for assessing and testing cooperative systems could prop in relating and mollifying implicit sources of psychosocial pitfalls. Also, the design of crucial features and performance related to cooperative systems should consider these aspects. Simulations and modeling play a vital part in supporting and validating these design choices.
3.1.3 Other challenges
A cobot must be built similarly to a traditional robot in order to maximize task execution quality, the ergonomics of a human coworker, and ensure his safety.
Millions of artificial robots have been used in production environments across the globe. Therefore, it would be more desirable to develop technologies that can transform them into mortal-safe robotic systems with no attack variations rather than replacing all of these traditional robots with safe cobots at a huge expense. Reprogram capability, scalability and literacy capability of cooperative robots is also a big challenge. On the same line, erecting a stoner-friendly mortal—robot interfaces come up with a challenge.
Real- time constraints are a critical aspect of mortal- robot commerce (HRI) in the realm of artificial cobotics. Meeting these constraints is essential to insure the trust ability and effectiveness of the system. When calculations exceed predefined thresholds, it can affect in- deterministic geste and potentially lead to system failures with severe consequences. These limitations have significant counteraccusations for colorful aspects of HRI, including mortal action recognition, contemporaneous discovery of multiple conduct, anti-collision strategies, control armature, and 3D vision.
A significant challenge in the advancement of mortal- robot commerce (HRI) within artificial cobotic systems is the fault- forbearance paradigm. This paradigm aims to incorporate individual capabilities André-planning capacities, allowing the system to acclimatize stoutly grounded on the available coffers and trustability. Expansive exploration has been devoted to the fault forbearance model, but a comprehensive approach that seamlessly integrates this paradigm into the design of HRI and control infrastructures is still lacking.
There are colorful constraints and challenges associated with artificial cobotic systems that need to be addressed. These include achieving dependable discovery of mortal stir to enable the development of accurate prophetic systems, icing robust discovery of contact between robots and humans in multiple locales, and developing responsive regulators able of real- time liner-planning in complex and cluttered surroundings.
3.2 Summary of the discussion
According to the statistics, the most cutting-edge debate subjects for contact avoidance are Safety Management, Sensor Systems for Object Tracking, and Motion Planning and Control. Case studies, operations, help systems, and artificial intelligence all have minimal effects. The main themes of Motion Planning and Control include Mortal-stir Vaticinator, Line Modification, and Stir Control Techniques. The main topics covered in Sensor Systems for Object Tracking include the creation and fusion of monitoring and computer vision systems for gesture recognition, workplace management, and human localization. The design of methods, standards, and guidelines for connection obstruction operation is one of the main themes in safety management.
The statistics show that Motion Planning and Control, Robot System Design, and sensor systems for contact operation are the most cutting-edge dissertation issues for Contact Discovery and Mitigation. Case studies, operations, safety management, simulation and modeling provide only minor contributions. The primary topics for Motion Planning and Control are control strategies. The development of robot attack and design methods is the focus of Robot System Design. The development of sensor bias and methodology for discovery are the primary contents for sensor systems for contact operation.
Task Scheduling Strategy is the most sophisticated physical ergonomics discourse theme, according the data. Motion Planning and Control and Assistance Systems provide a small contribution. The main focus of Task Scheduling Strategy is on assigning and organizing robot-
Human task sequences while incorporating physical ergonomics.
Metrics and Tests, Motion Planning and Control, and Simulation and Modeling appear to be the most sophisticated discussion themes for Cognitive and Organizational Ergonomics. Task scheduling strategy and assistance systems provide insignificant contributions. The development of an evaluation technique for robot acceptability and the establishment of an organizational framework for effective HRI operations performance are the major topics covered in Metrics and Tests. The primary topics for Motion Planning and Control are control tactics connected to cognitive components of HRI. The creation of virtual reality exploitation for the assessment of the cognitive aspects of HRI is one of the key topics covered in Simulation and Modeling.
The current trend in artificial cobotics is concentrated on developing flexible systems that enable safe and cooperative relations between humans and robots to negotiate colorful tasks. This growing trend encourages diligence to consider integrating similar cobotic systems into their being manufactories. In the coming times, cobots are anticipated to play a pivotal part and become the dominant technology for named operations, potentially filling the maturity of the remaining 90 of workstations. It's worth noting that a significant number of exploration studies have been devoted to addressing safety and security enterprises in cobotic systems, exercising different technologies and approaches to insure the well- being of mortal workers and the overall system integrity.
Table shown below Tables 2 and 3 concisely represents the entire summary of review of safety and ergonomics consisting both sub clusters for each.
Summary of literature related to Safety cluster of Collaborative robots:
Summary of literature related to Ergonomics cluster of Collaborative robots:
4 Conclusion
Over the past few years, industrial collaborative robotics has attracted a great deal of attention, and human–robot interaction (HRI) has become a vital area of study. This study did a thorough analysis of the literature and developed a tentative classification system, classifying and sub classifying significant works and new research in this field. This study's main goal was to identify and evaluate the burgeoning topics and research problems in safety and ergonomics in industrial collaborative robotics.
For each selected article, a summary was provided, outlining the problem addressed, the proposed approach, the main outcomes obtained, and potential future directions for research. The study also acknowledged the existence of a significant gap between the research carried out in laboratory settings and the practical implementation of cobotic technology in real industrial environments, particularly in the context of smart factories.
The findings of the review indicated that safety was the most extensively explored research category, although ergonomics has witnessed notable growth in recent years. Interestingly, the majority of high-level themes identified were more closely related to safety aspects rather than ergonomics. Within the realm of safety, there was a greater emphasis on prevention rather than protection measures.
Several difficulties and problems encountered by researchers studying HRI in industrial cobots were noted and emphasized towards the end of the work. Considering the continuous growth of the industrial collaborative robot market, these innovations hold promise for the implementation of collaborative production systems that are safe, ergonomic, trustworthy, and efficient.
Data availability
Not applicable.
De Santis A, Siciliano B. Safety issues for human-robot cooperation in manufacturing systems. Tools and Perspectives in Virtual Manufacturing. VRT.
Bicchi A, Peshkin MA, Colgate JE. Safety for physical human–robot interaction. In: Siciliano B, Khatib O, editors. Springer handbook of robotics. Heidelberg: Springer; 2008. p. 1335–48.
Chapter Google Scholar
Wang N, Zeng Y, Geng J. A brief review on safety strategies of physical human-robot interaction. In: ITM Web of Conferences. Vol. 25. EDP Sciences; 2019. p. 01015.
International Organization for Standardization S Geneva. ISO 10218-2:2011 robots and robotic devices—safety requirements for industrial robots—part 2: robot systems and integration. 2016. (Tech Rep).
L Gualtieri. Safety, ergonomics and efficiency in human-robot collaborative assembly: design guidelines and requirements, 30th CIRP Design 2020 (CIRP Design 2020), Industrial engineering and automation.
Schmidt B, Wang L. Vision-guided active collision avoidance for human-robot collaborations. Manuf Lett. 2013;1(1):5–8.
Article Google Scholar
Long P, Chevallereau C, Chablat D, Girin A. An industrial security system for human–robot coexistence. Ind Robot: Int J. 2018;45(2):220–6.
Heydaryan S, SuazaBedolla J, Belingardi G. Safety design and development of a human–robot collaboration assembly process in the automotive industry. Appl Sci. 2018;8(3):344.
Chen Xi. Industrial robot control with object recognition based on deep learning. Procedia CIRP. 2018;76:149–54.
De Luca A, Flacco F. Integrated control for PHRI: collision avoidance, detection, reaction and collaboration. In: 2012 4th IEEE RAS & EMBS International Conference on Biomedical Robotics and Biomechatronics (BioRob). IEEE; 2012. p. 288–95.
Flacco F. A depth space approach for evaluating distance to objects. J Intell Rob Syst. 2014;80(S1):1–16.
Google Scholar
Navarro B, Cherubini A, Fonte A, et al. An iso10218-compliant adaptive damping controller for safe physical human-robot interaction. In: 2016 IEEE International Conference on Robotics and Automation (ICRA). IEEE. 2016. p. 3043–8.
Morato C, Kaipa KN, Zhao B, et al. Toward safe human robot collaboration by using multiple kinects based real-time human tracking. J Comput Inf Sci Eng. 2014;14(1):011006.
Avanzini GB, Ceriani NM, Zanchettin AM, et al. Safety control of industrial robots based on a distributed distance sensor. IEEE Trans Control Syst Technol. 2014;22(6):2127–40.
Bdiwi M, Pfeifer M, Sterzing A. A new strategy for ensuring human safety during various levels of interaction with industrial robots. CIRP Ann. 2017;66(1):453–6.
Heo YJ, Lee W. Collision detection for industrial collaborative robots: a deep learning approach. IEEE Robot Autom Lett. 2019. https://doi.org/10.1109/LRA.2019.2893400 .
Wang XV. Overview of human-robot collaboration in manufacturing. In: Proceedings of the 5th international conference on the industry 4.0 model for advanced manufacturing at: Belgrade, Serbia. 2020. https://doi.org/10.1007/978-3-030-46212-3_2 .
Zhang P, Jin P, Du G, Liu X. Ensuring safety in human–robot coexisting environment based on two-level protection. Ind Robot: Int J. 2016;43(3):264–73.
Schiavi R, Bicchi A, Flacco F. Integration of active and passive compliance control for safe human-robot coexistence. In: 2009 IEEE International Conference on Robotics and Automation. IEEE; 2009. p. 259–64.
De Luca A, Albu-Schaffer A, Haddadin S, et al. Collision detection and safe reaction with the DLR-III lightweight manipulator arm. In: 2006 IEEE/RSJ International Conference on Intelligent Robots and Systems. IEEE; 2006.
Haddadin S, Albu-Schaffer A, De Luca A, et al. Collision detection and reaction: a contribution to safe physical human-robot interaction. In: 2008 IEEE/RSJ International Conference on Intelligent Robots and Systems. IROS 2008. IEEE; 2008. p. 3356–63.
De Benedictis C, Franco W, Maffiodo D, et al. Control of force impulse in human-machine impact. In: International Conference on Robotics in Alpe-Adria Danube Region. Springer. 2017. p. 956–64.
Indri M, Trapani S, Lazzero I. A general procedure for collision detection between an industrial robot and the environment. In: 2015 IEEE 20th Conference on Emerging Technologies & Factory Automation (ETFA). IEEE. 2015. p. 1–8.
Lee SD, Song JB. Sensorless collision detection based on friction model for a robot manipulator. Int J Precis Eng Manuf. 2016;17(1):11–7.
Ren T, Dong Y, Wu D, Chen K. Collision detection and identification for robot manipulators based on extended state observer. Control Eng Pract. 2018;79:144–53.
Bortot DF. Ergonomic human-robot coexistence in the branch of production [PhD thesis]. Technische Universität München; 2014.
Fraboni F. Evaluating organizational guidelines for enhancing psychological well being, safety and performance in technology integration. Sustainability. 2023. https://doi.org/10.3390/su15108113 .
Sadrfaridpour B, Wang Y. Collaborative assembly in hybrid manufacturing cells: an integrated framework for human–robot interaction. IEEE Trans Autom Sci Eng. 2018;15(3):1178–92.
Cherubini A, Passama R, Crosnier A, Lasnier A, Fraisse P. Collaborative manufacturing with physical human–robot interaction. Robot Comput-Integr Manuf. 2016;40:1–13.
Dannapfel M, Bruggräf P, Bertram S, Förstmann R, Riegauf A. Systematic planning approach for heavy-duty human–robot cooperation in automotive flow assembly. Int J Electr Electron Eng Telecommun. 2018;7(2):51.
Bluethmann W, Ambrose R, Diftler M, et al. Robonaut: a robot designed to work with humans in space. Auton Robots. 2003;14(2–3):179–97.
Article MATH Google Scholar
Müller R, Vette M, Mailahn O. Process-oriented task assignment for assembly processes with human-robot interaction. Procedia CIRP. 2016;44:210–5.
Maurice P, Padois V, Measson Y, et al. Humanoriented design of collaborative robots. Int J Ind Ergon. 2017;57:88–102.
Tang G, Webb P. The design and evaluation of an ergonomic contactless gesture control system for industrial robots. J Robot. 2018. https://doi.org/10.1155/2018/9791286 .
Faber M, Mertens A, Schlick CM. Cognition-enhanced assembly sequence planning for ergonomic and productive human–robot collaboration in self-optimizing assembly cells. Prod Eng. 2017;11(2):145–54.
Solvang B, Sziebig G. On industrial robots and cognitive info-communication. In: 2012 IEEE 3rd International Conference on Cognitive Infocommunications (CogInfoCom). IEEE; 2012. p. 459–64.
Shravani NK, Rao SB. Introducing robots without creating fear of unemployment and high cost in industries. Int J Eng Technol Sci Res. 2018;5(1):1128–38.
De Santis A. Modelling and control for human-robot interaction: physical and cognitive aspects. In: 2008 IEEE International Conference on Robotics and Automation. IEEE; 2008.
Medina JR, Lorenz T, Hirche S. Synthesizing anticipatory haptic assistance considering human behavior uncertainty. IEEE Trans Robot. 2015;31(1):180–90.
Matsas E, Vosniakos GC, Batras D. Prototyping proactive and adaptive techniques for human–robot collaboration in manufacturing using virtual reality. Robot Comput-Integr Manuf. 2018;5:168–80.
Maurtua I, Ibarguren A, Kildal J, Susperregi L, Sierra B. Human–robot collaboration in industrial applications: safety, interaction and trust. Int J Adv Robot Syst. 2017;14(4):1729881417716010.
Charalambous G, Fletcher S, Webb P. Identifying the key organisational human factors for introducing human–robot collaboration in industry: an exploratory study. Int J Adv Manuf Technol. 2015;81(9–12):2143–55.
Rahman SM, Wang Y. Mutual trust-based subtask allocation for human–robot collaboration in flexible lightweight assembly in manufacturing. Mechatronics. 2018;54:94–109.
Koppenborg M, Nickel P, Naber B, Lungfiel A, Huelke M. Effects of movement speed and predictability in human–robot collaboration. Hum Fact Ergon Manuf Serv Ind. 2017;27(4):197–209.
Download references
Author information
Authors and affiliations.
Department of Mechanical Engineering, National Institute of Technology Warangal, Warangal, 506004, India
Swapnil Patil, V. Vasu & K. V. S. Srinadh
You can also search for this author in PubMed Google Scholar
Contributions
All Authors reviewed the manuscript.
Corresponding author
Correspondence to V. Vasu .
Ethics declarations
Competing interests.
The authors declare no competing interests.
Additional information
Publisher's note.
Springer Nature remains neutral with regard to jurisdictional claims in published maps and institutional affiliations.
Rights and permissions
Open Access This article is licensed under a Creative Commons Attribution 4.0 International License, which permits use, sharing, adaptation, distribution and reproduction in any medium or format, as long as you give appropriate credit to the original author(s) and the source, provide a link to the Creative Commons licence, and indicate if changes were made. The images or other third party material in this article are included in the article's Creative Commons licence, unless indicated otherwise in a credit line to the material. If material is not included in the article's Creative Commons licence and your intended use is not permitted by statutory regulation or exceeds the permitted use, you will need to obtain permission directly from the copyright holder. To view a copy of this licence, visit http://creativecommons.org/licenses/by/4.0/ .
Reprints and permissions
About this article
Patil, S., Vasu, V. & Srinadh, K.V.S. Advances and perspectives in collaborative robotics: a review of key technologies and emerging trends. Discov Mechanical Engineering 2 , 13 (2023). https://doi.org/10.1007/s44245-023-00021-8
Download citation
Received : 31 May 2023
Accepted : 11 August 2023
Published : 29 August 2023
DOI : https://doi.org/10.1007/s44245-023-00021-8
Share this article
Anyone you share the following link with will be able to read this content:
Sorry, a shareable link is not currently available for this article.
Provided by the Springer Nature SharedIt content-sharing initiative
- Collaborative robots
- Human Robot Interaction (HRI)
- Collision avoidance
- Contact detection
- Find a journal
- Publish with us
- Track your research
Having a machine learning agent interact with its environment requires true unsupervised learning, skill acquisition, active learning, exploration and reinforcement, all ingredients of human learning that are still not well understood or exploited through the supervised approaches that dominate deep learning today. Our goal is to improve robotics via machine learning, and improve machine learning via robotics. We foster close collaborations between machine learning researchers and roboticists to enable learning at scale on real and simulated robotic systems.
Recent Publications
Some of our teams.
We're always looking for more talented, passionate people.
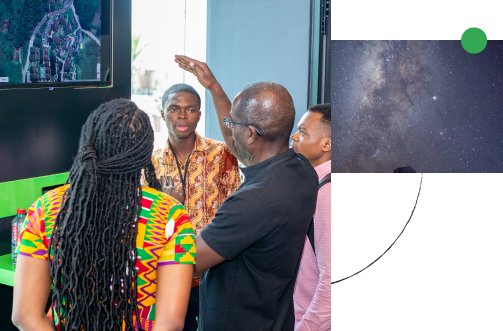
- Skip to right header navigation
- Skip to primary navigation
- Skip to secondary navigation
- Skip to main content
- Skip to primary sidebar

University of California, Berkeley Mechanical Engineering
- From the Chair
- Honors and Rankings
- Visitor Information
- Equity and Inclusion
- 150 Years of Women in ME
- Make a Gift
- Faculty by Research Area
- Faculty Books
- Administrative
- Information Technology
- Student Services
- Student Machine Shops
- Community Spotlight
- External Advisory Board
- Lecturer Positions
- Visiting Scholars
- Curriculum Flowchart
- Degree Requirements
- ME + Business
- ME/MSE Joint Major
- ME/NE Joint Major
- Aerospace Engineering Minor
- Fifth Year B.S./M.S. Program
- Simultaneous Degrees
- Semesterly Advising
- Faculty Adviser Assignments
- Faculty Office Hours
- Career Planning Maps
- Applying to Engineering
- The Application
- Junior Transfers
- Credit from Exams
- Drake Scholarship
- Financial Resources
- Tentative ME Course Schedule
- Technical Electives
- Design Course Transition
- Quantitative Science
- Humanities & Social Sciences Courses
- Undergrad Course Syllabi
- ME DeCal Courses
- Credit for Research
- Research Samples
- Past Prize Winners
- Student Life Resources
- Jobs and Internships
- Program Objectives and Outcomes (ABET)
- Ph.D. & D.Eng.
- Master of Science
- Master of Engineering
- 5th Year Masters Program Handbook
- Special Programs
- M.S., Ph.D., D.Eng. & CWO Application
- MEng Application
- 5th Year Masters Admissions
- Application Tips
- Fees and Financial Support
- Readmission / Change of Major
- Graduate Handbook
- Graduate Forms
- Prelim Exams
- Research Areas and Major Fields
- Major Field Advisors
- Grant Writing
- Grad Division Resources
- COE Graduate Guide
- GSI/Reader Forms
- Tentative ME course schedule
- Graduate Course Syllabi
- Laboratories
- Research Centers
- Student Academic Resources
- Graduate Resources
- ME Student Groups
- Virtual Career Panel Series
- Room Reservations
- Safety Information
- Key Requests
- Sexual Violence & Sexual Harassment Prevention
- Mail & Office Administration
- Faculty & Staff Resources
- Financial Services
- IT Services
- Shop Training
- Services Provided
- Shop Equipment
- Shop Safety
- Alumni Newsletter
- Mechanical Engineering Seminars
- Search for: Search Button
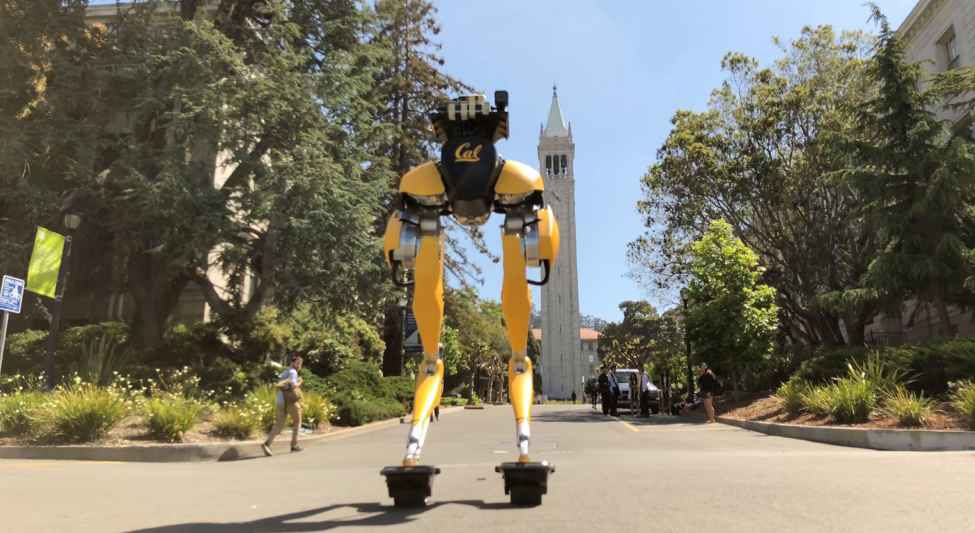
Core Faculty:
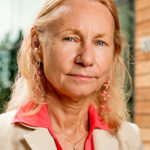
Alice M. Agogino
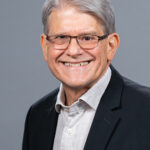
Roberto Horowitz
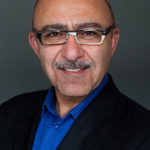
Homayoon Kazerooni
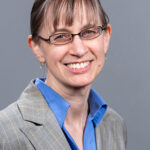
Sara McMains
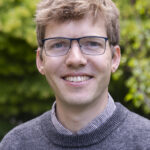
Mark W. Mueller
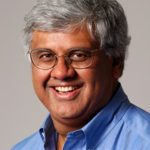
S. Shankar Sastry
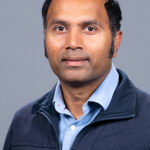
Koushil Sreenath
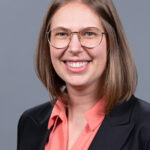
Hannah Stuart
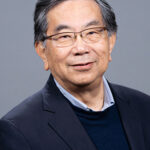
Masayoshi Tomizuka
Robotics labs:.
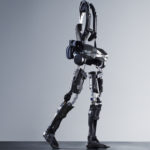
Berkeley Robotics & Human Engineering Laboratory
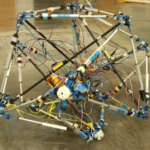
Embodied Dexterity Group
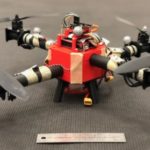
High Performance Robotics Lab
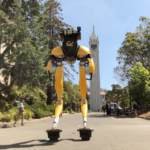
Hybrid Robotics
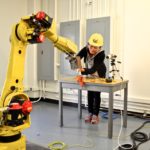
Mechanical Systems Control Lab
Berkeley robotics research in the news:.
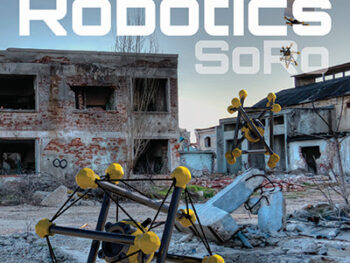
ME Professor and Ph.D. Student’s Research Featured in Soft Robotics
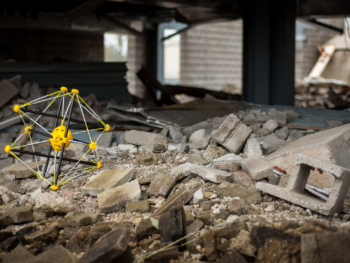
Entrepreneurship at Berkeley
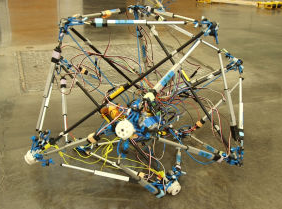
This device from Berkeley’s Squishy Robotics looks like a toy, but acts like an action hero
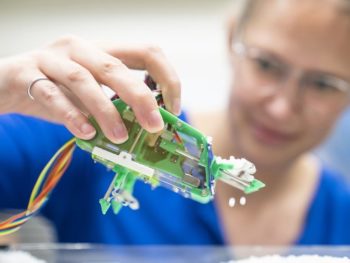
ME Assistant Professor Hannah Stuart Wins NSF CAREER Award
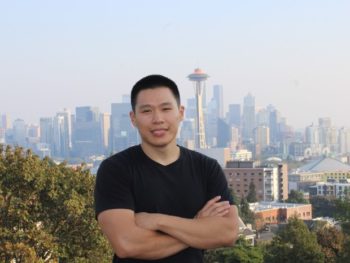
Patrick Cheng, MEng ’22 (ME): “You can’t grow without facing uncomfortable situations”
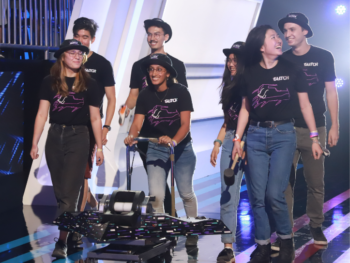
ME Student Group, Berkeley Combat Robotics, Competes on BattleBots
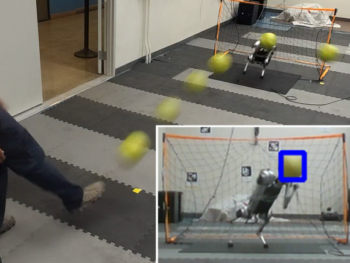
Goalkeeping Robot Dog Tends Its Net Like a Pro
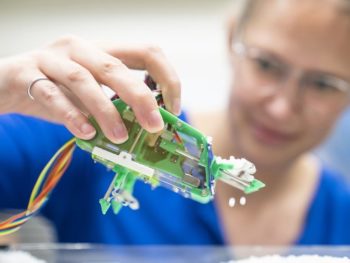
Digging Deep: Inspired by nature, the burrowing mole crab robot is a feat of engineering with real-world applications
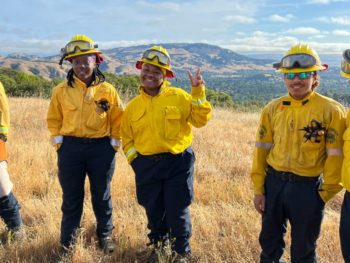
Fired Up for the Future
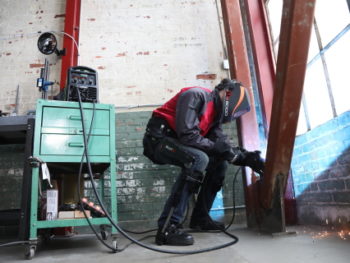
Ottobock Acquires Exoskeleton Specialist suitX
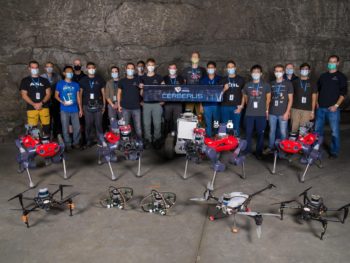
Team CERBERUS Wins DARPA Subterranean Challenge
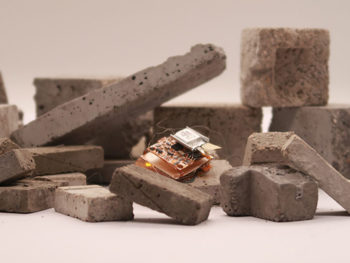
Insect-sized robot navigates mazes with the agility of a cheetah
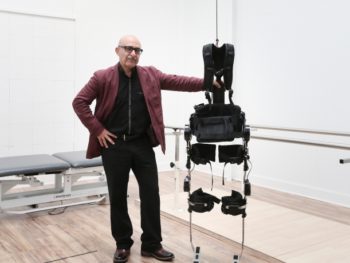
The ‘Iron Man’ body armour many of us may soon be wearing
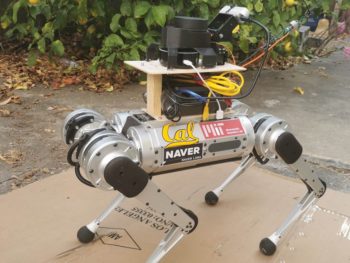
UC Berkeley researchers create robotic guide dog for visually impaired people
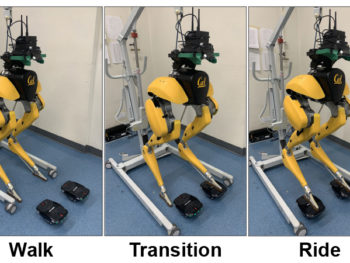
Capstone Project Profile: Adapting Humanoid Robots to Aid First Responders
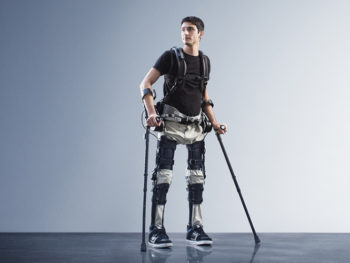
UC Berkeley Researchers Motivated by Society, Personal Interests, Scientific Community
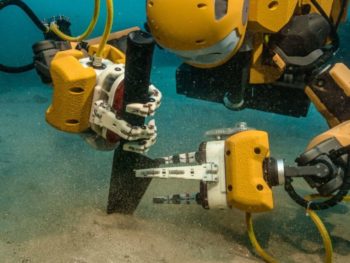
The EDG Lab Discovers How to Better Design Skin for Handling Wet and Submerged Objects
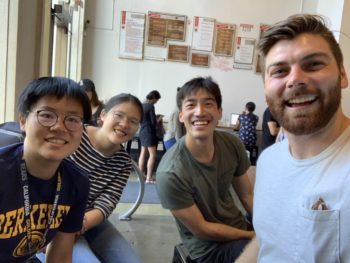
Q&A with the Capstone Winners of 2020 Fung Institute Mission Award
Robotics - Mechanical Engineering - Purdue University

Robotics
21st century robots are just as likely to be hovering in the air, or swimming through someone’s bloodstream, as working in an automotive factory. That’s why Purdue researchers pursue robotics on all fronts: manufacturing, biomedicine, design, nanotechnology, and more. From large scale automation, such as in manufacturing; to microrobots moving individual cells; every aspect of robotics is explored at Purdue. Especially important is human-computer interaction, so that people from every background can utilize the newest technology in the most productive way.
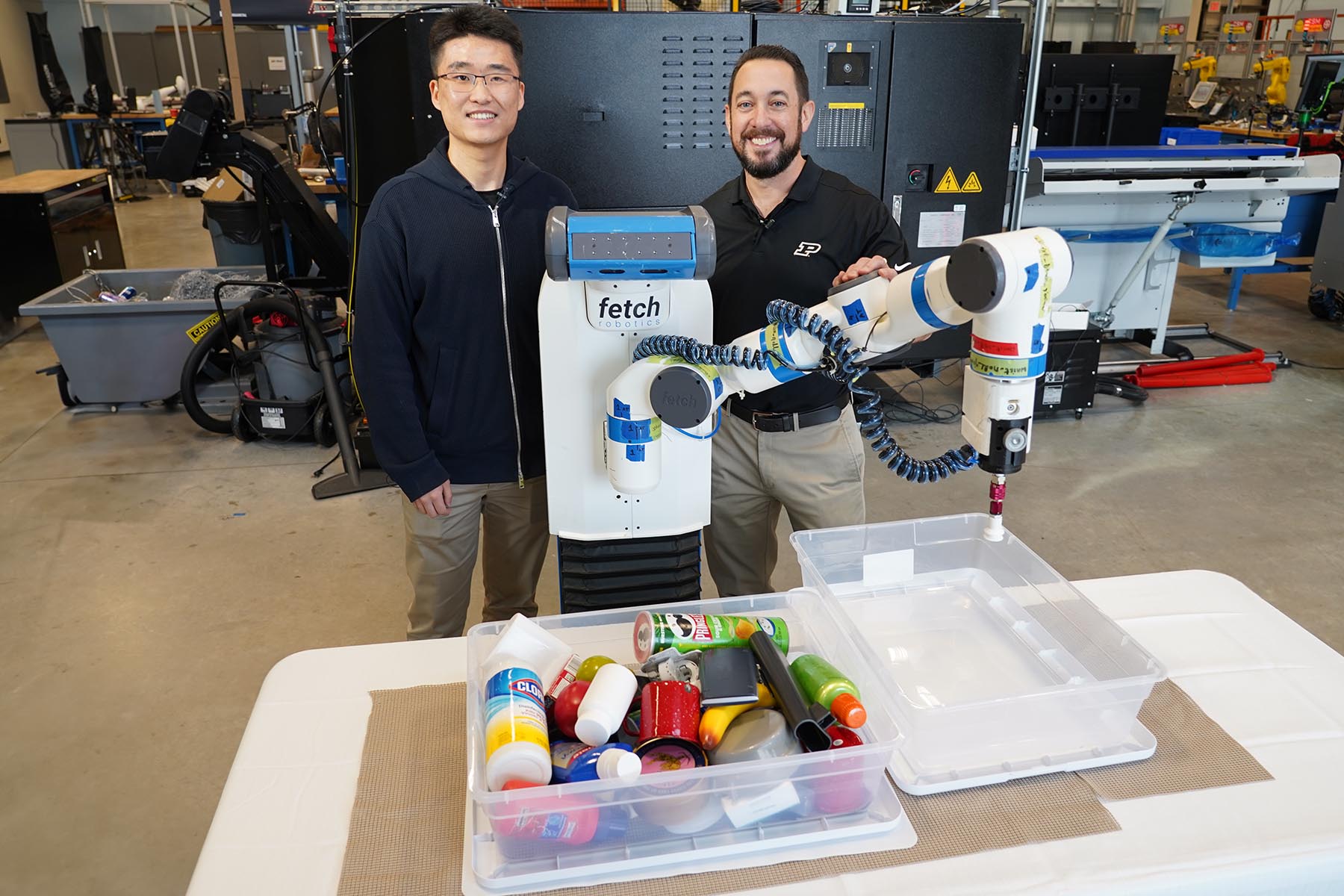
Autonomous grasping robots will help future astronauts maintain space habitats

Helping robots find their sea legs
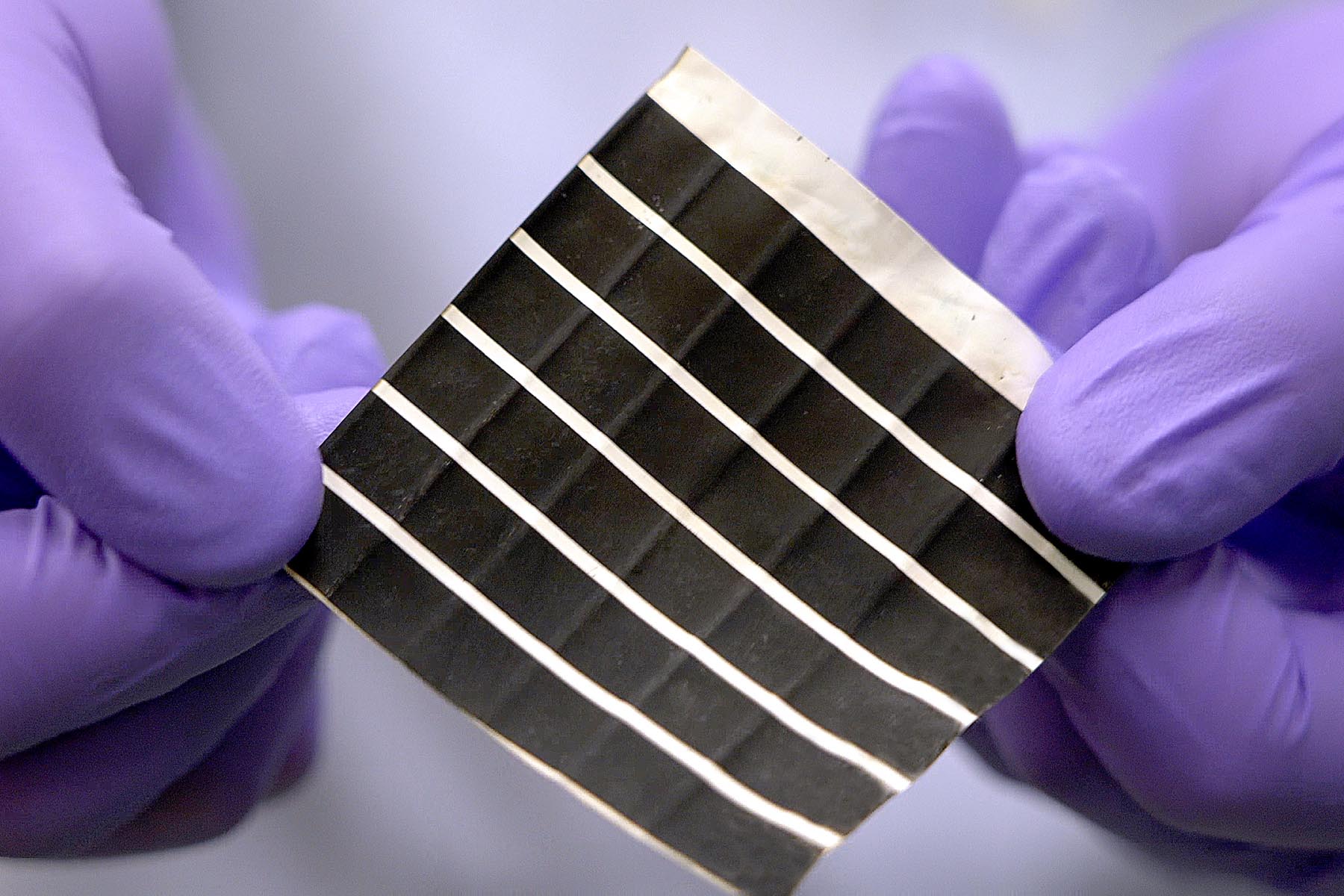
Soft morphing robotics inspired by pixel displays
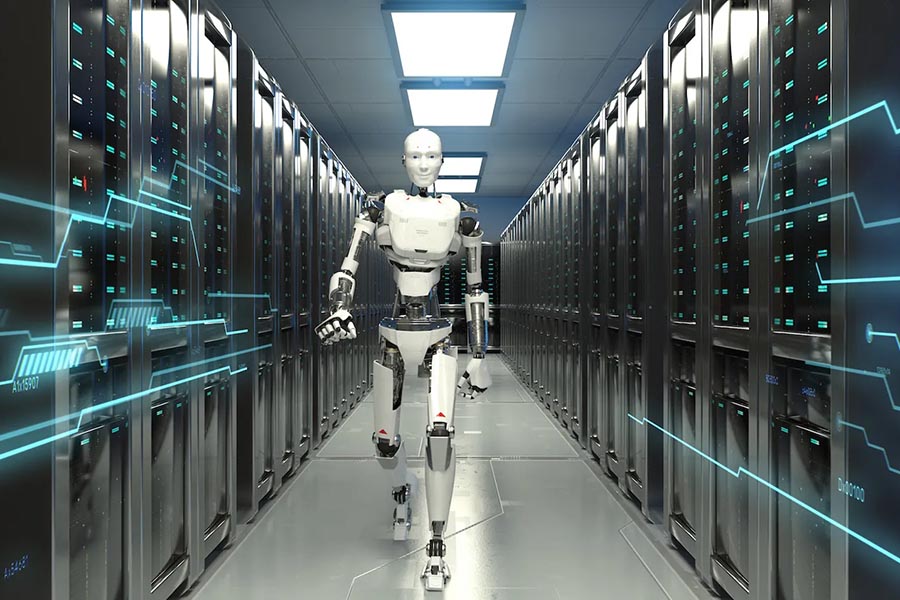
Robots get their sea legs
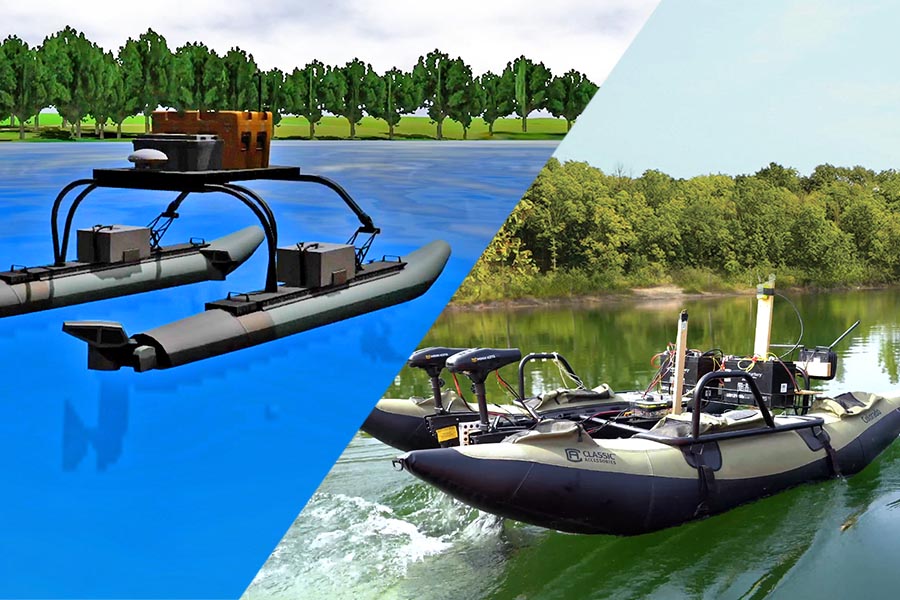
Purdue wins virtual maritime robotics competition
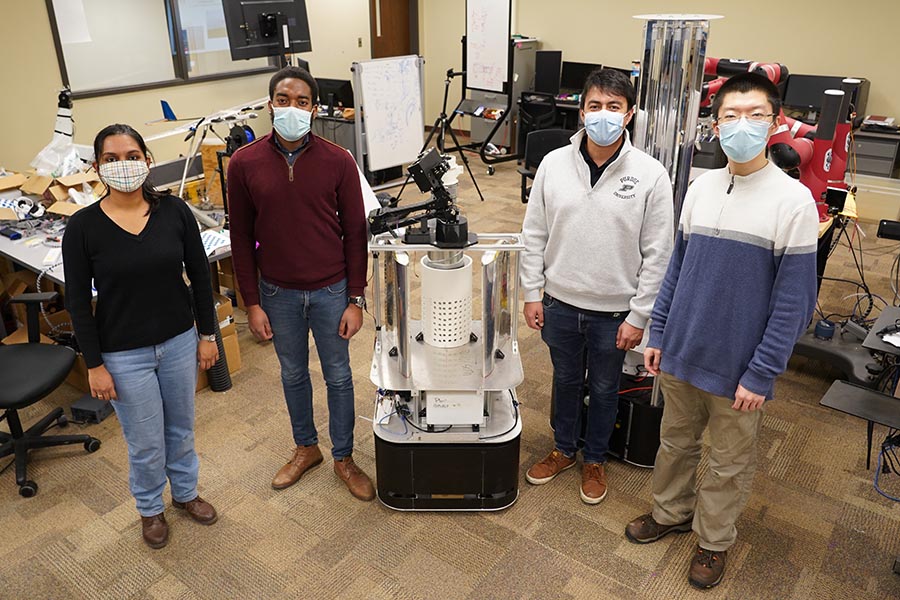
This Robot Disinfects Classrooms
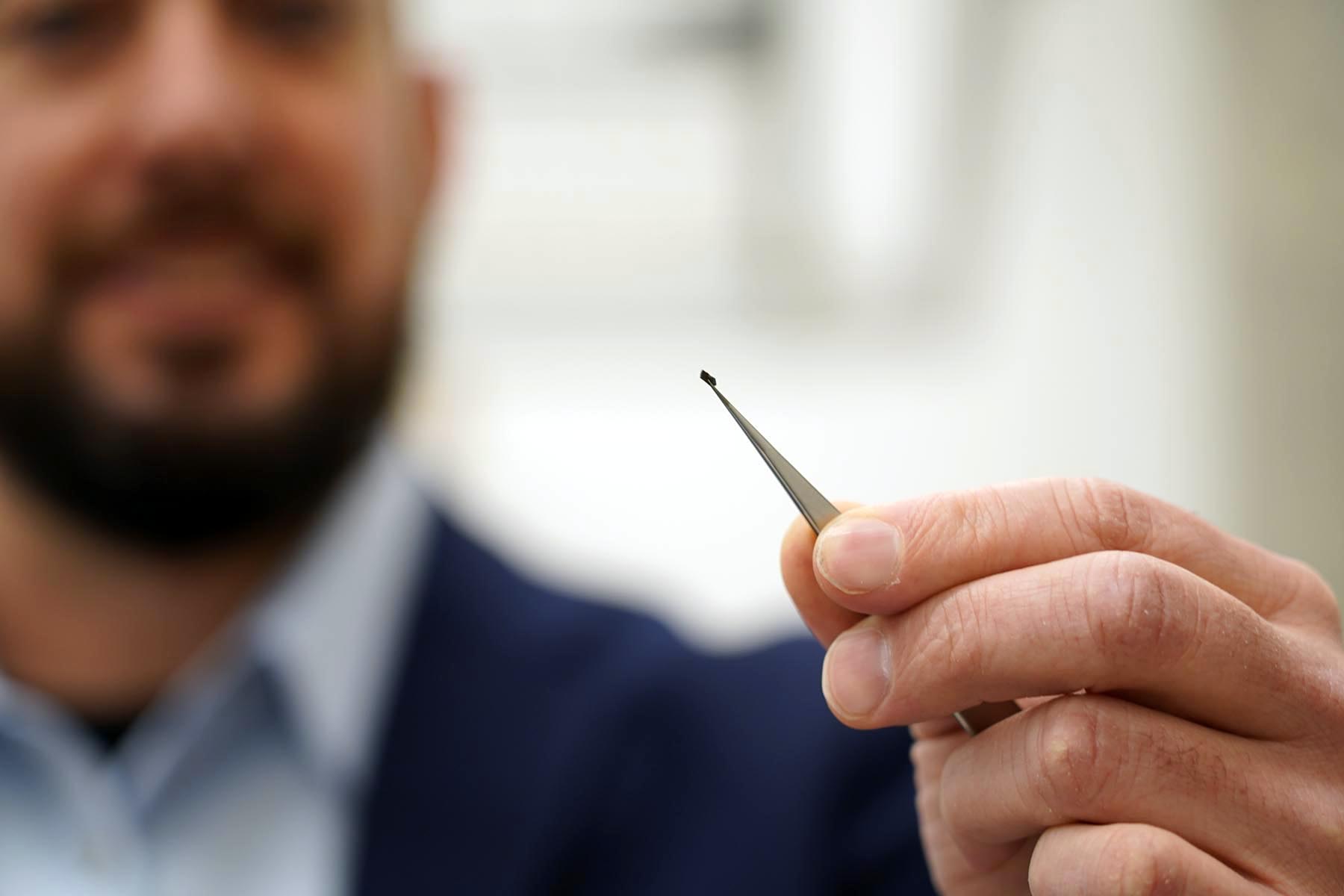
Tackling inflammatory bowel disease with tumbling microrobots
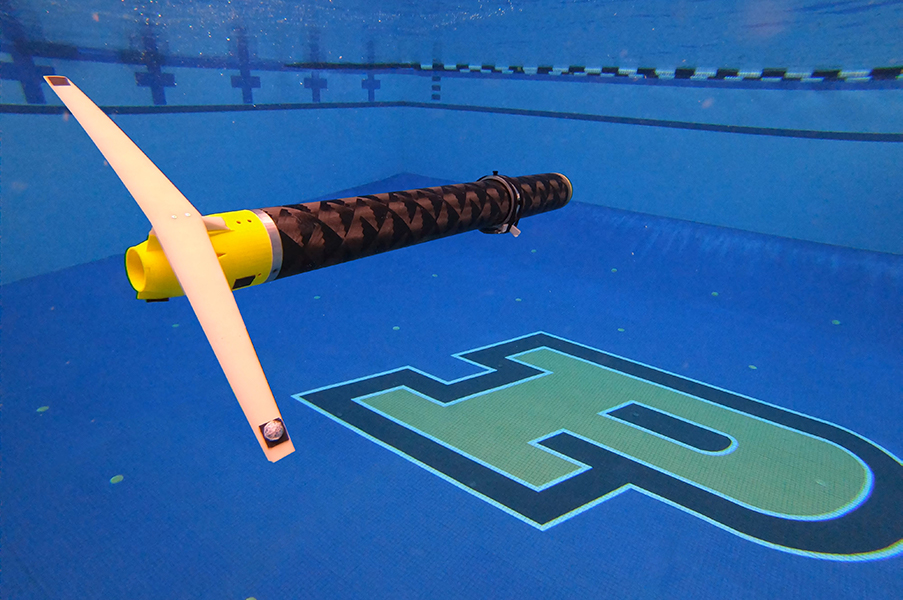
Underwater glider quietly surveys the seas
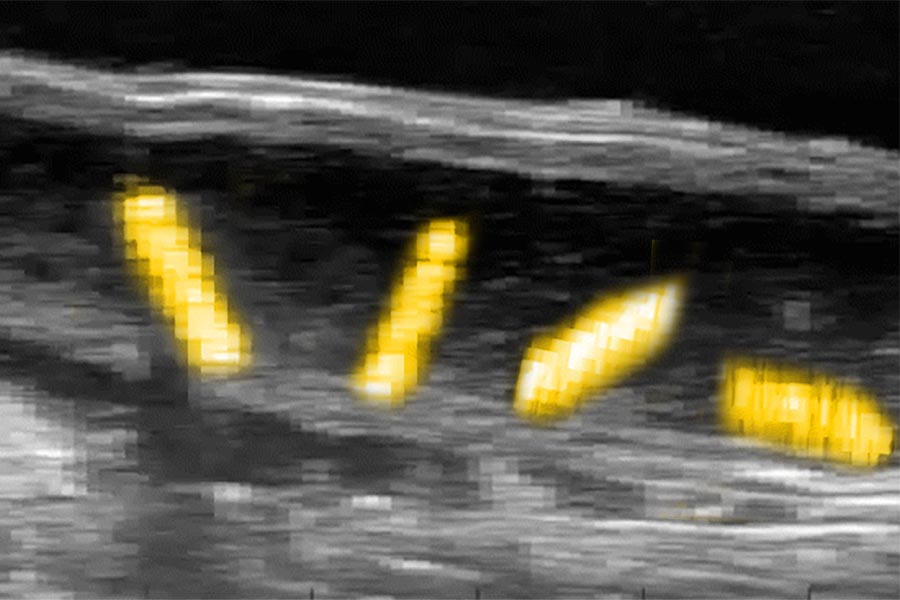
All-terrain microrobot flips through a live colon
Faculty in Robotics
Andres arrieta.
- Adaptive structures
- Mechanical metamaterials
- Robotic materials
- Programmable structures
- Multistable structures
- Structural nonlinearity
- Elastic instabilities
- Structural dynamics
- Nonlinear vibrations
Laura Blumenschein
- Growing robots
- Soft robotics
- Bioinspired systems
- Wearable robots
- Soft matter
David Cappelleri
- Multi-scale robotic manipulation and assembly
- Mobile micro/nano robotics
- Micro/nano aerial vehicles
- Micro-Bio robotics
- Mechatronics
- Automation for the life sciences
Alex Chortos
- Bio-inspired and mechanically adaptive electronics
- Multimaterial additive fabrication
- Soft actuators (artificial muscles)
- Wearable actuators (haptics)
- Polymer design and polymer physics
- Deformation sensors and transistors
Xinyan Deng
- Principles of aerial and aquatic locomotion in animals
- Experimental fluid mechanics
- Bio-inspired robotics
- Biologically inspired micro aerial vehicles and underwater robots
- Bio-sensing and sensor fusion algorithms
Shirley Dyke
- Structural Dynamics and Control
- Cyber-physical Systems
- Machine Vision
- Real-time Hybrid Simulation
- Damage Detection and Structural Condition Monitoring
- Cyberinfrastructure Development
- Legged locomotion
- Humanoid and quadrupedal robots
- Wearable robotics
- Hybrid dynamical systems
- State estimation
Nina Mahmoudian
- Marine Robotics
- Unmanned Systems
- Energy Autonomy
- Systems Design
- Coordination and Controls
Peter Meckl
- Motion and vibration control
- Adaptive control
- Intelligent control using fuzzy logic and neural networks
- Engine and emissions diagnostics
Gordon Pennock
- Kinematic synthesis and analysis
- Multi-degree-of-freedom mechanisms
Karthik Ramani
- Human Skill and Augmentation
- Collaborative and Hybridized Intelligence
- Deep Learning of Shapes and Computer Vision
- Human-Robot-Machine Interactions
- Making to Manufacturing (M2M)
- Factory of the Future and Robotics
- Manufacturing Productivity
Daniel Williams
- Vehicle Chassis Control Systems
- Vehicle Dynamics
- Autonomous Vehicles
- Human Driver Dynamics
- Adaptive and robust control
- Nonlinear control
- Precision control of mechanical systems
- Vehicle control
I want to research in these fundamental areas...
I want to have an impact in....
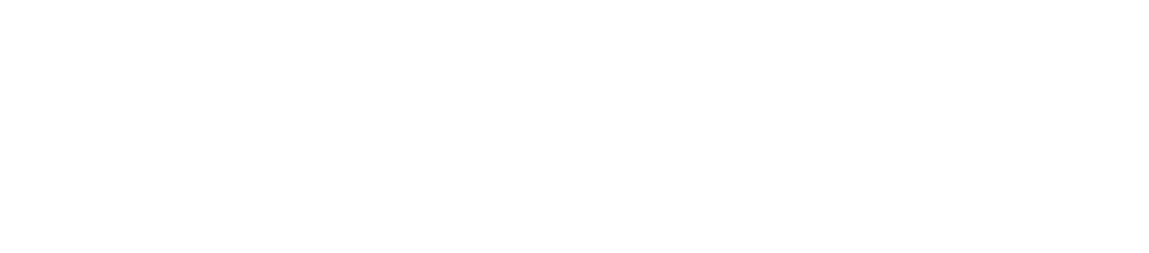
Departments
- Applied Physics
- Biomedical Engineering
- Center for Urban Science and Progress
- Chemical and Biomolecular Engineering
- Civil and Urban Engineering
- Computer Science and Engineering
- Electrical and Computer Engineering
- Finance and Risk Engineering
- Mathematics
- Mechanical and Aerospace Engineering
- Technology, Culture and Society
- Technology Management and Innovation
Degrees & Programs
- Bachelor of Science
- Master of Science
- Doctor of Philosophy
- Digital Learning
- Certificate Programs
- NYU Tandon Bridge
- Undergraduate
- Records & Registration
- Digital Learning Services
- Teaching Innovation
- Explore NYU Tandon
- Year in Review
- Strategic Plan
- Diversity & Inclusion
News & Events
- Social Media
Looking for News or Events ?
At NYU, we believe that robotics has a central role to play in future urban environments and the improvement of human life, from mobility to healthcare, from infrastructure management to the service industry.
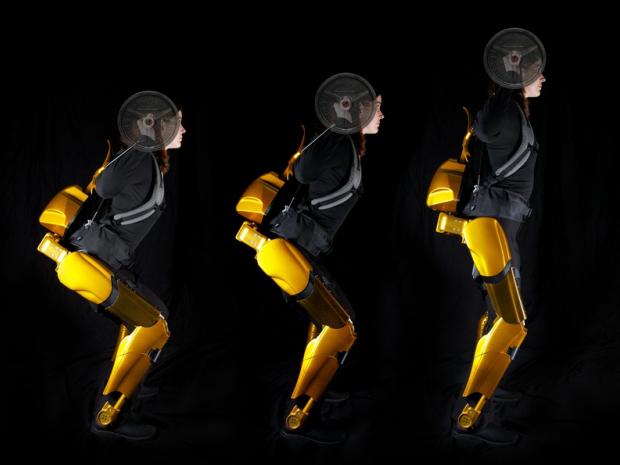
Robotics research and education at NYU focuses on developing and teaching the fundamental principles, theories, and algorithms for autonomous intelligent machines. Through our research and education, we aim to enhance mobility, service, infrastructure, and healthcare. Our mission is to carry out fundamental and multidisciplinary research to advance the science of robotics, to educate and mentor students in the theory and practice of robotics, and to make a positive impact on society.
Degree programs in robotics
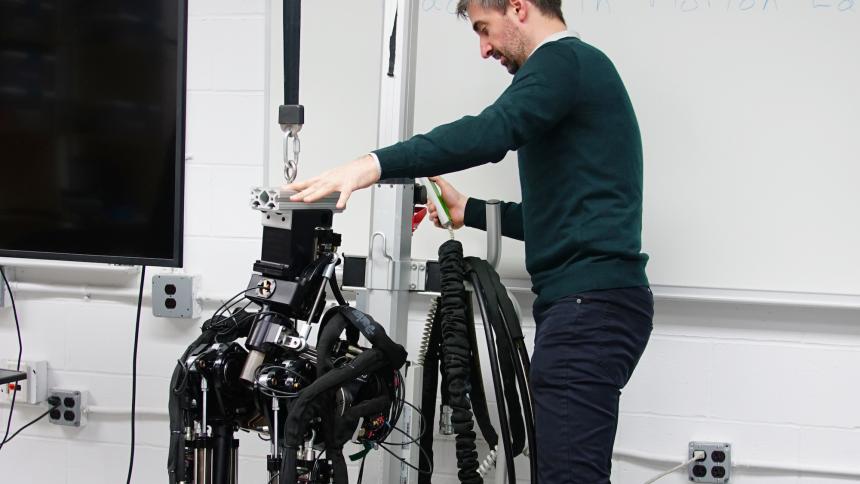
Mechatronics and Robotics, M.S.
Students in this program learn fundamental theory, modeling methods, hardware components, interfacing requirements, simulation and programming tools, and practical applications of mechatronics and robotics.
Learn more about the Mechatronics and Robotics, M.S.
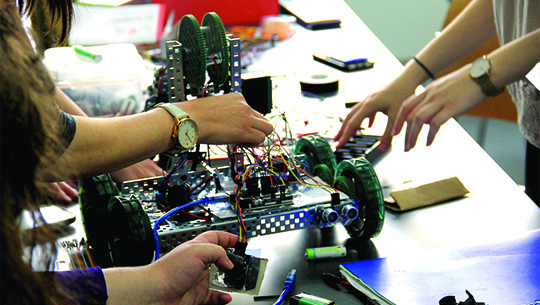
Undergraduate Minor in Robotics
The Robotics Minor consists of four undergraduate ROB courses. The minor teaches the fundamentals of robotics: kinematics, dynamics, manipulation, locomotion, planning, vision, and human-robot interaction. Students will have hands-on experience. Interested students should allow 4 semesters (two years) to complete the four courses for the minor in robotics.
More information about the robotics minor is available in the Bulletin .
Robotics Courses
Undergraduate Courses Graduate Courses
*Before taking undergraduate ROB courses, students should first take appropriate courses in math, physics, and computer science: Calculus (MA-UY 1124), Mechanics (PH-UY 1013), Programming (CS-UY 1114 or CS-UY 1133), Linear Algebra and Differential Equations (e.g., MA-UY 2034).
VIP Robotics Teams
Students* can earn credit by participating in Vertically Integrated Projects (VIPs) related to robotics.
*Projects marked with GY are open to graduate student participation
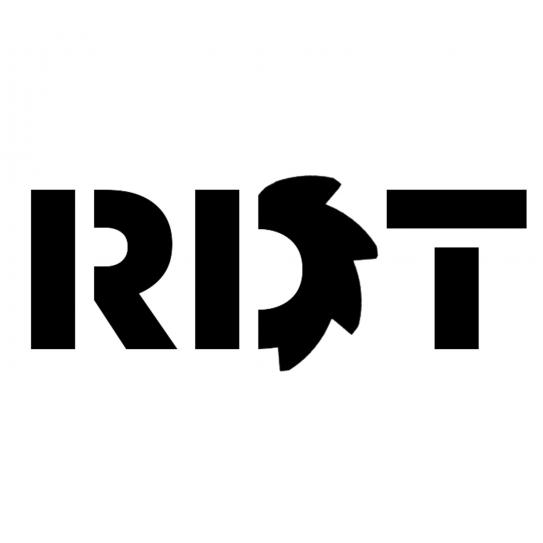
NYU Robotic Design Team
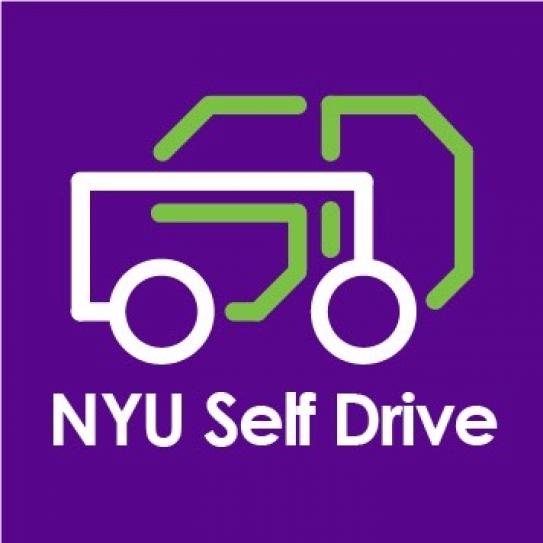
NYU Self Drive (GY)
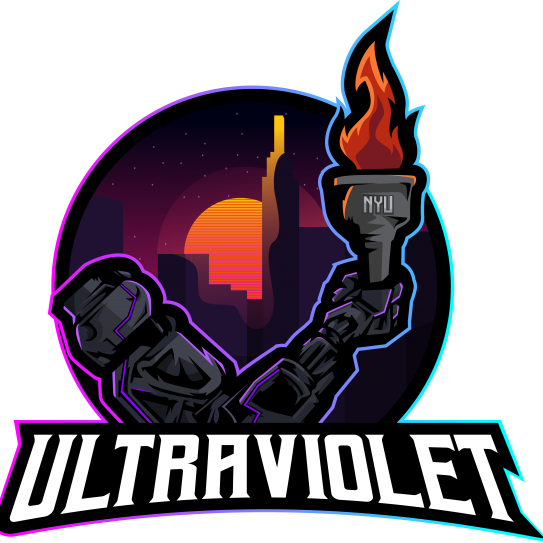
RoboMaster: Team UltraViolet (GY)
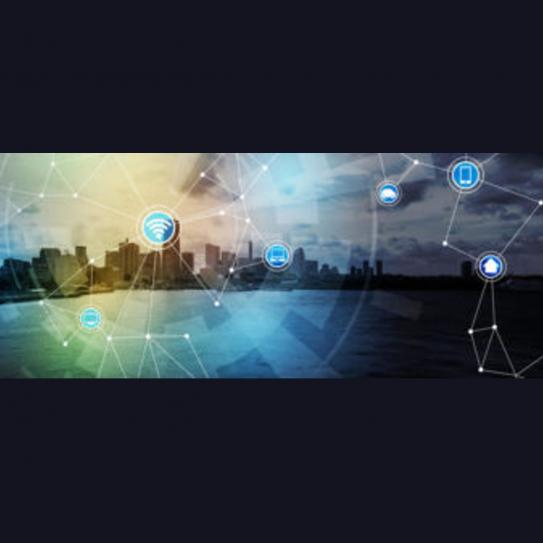
Smart Internet of Controlled Things
Research areas.
- Aerial robotics, autonomous drones
- Autonomous ground vehicles
- Control theory
- Computer vision for robotics
- Cyberphysical systems
- Dynamical systems
- Game theory and applications
- Learning-based control
- Legged locomotion
- Machine learning
- Mechatronics
- Medical, surgical, and rehabilitation robotics
- Resiliency and security
- Robotic manipulation
- Stability and energetics
Labs and Groups
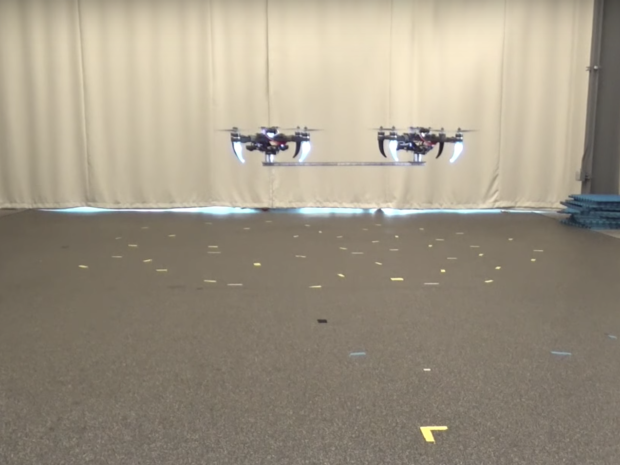
Agile Robotics and Perception Lab
ARPL performs fundamental and applied research in robot autonomy. The lab develops agile autonomous drones that can navigate on their own using only onboard sensors without relying on maps, GPS or motion capture systems.
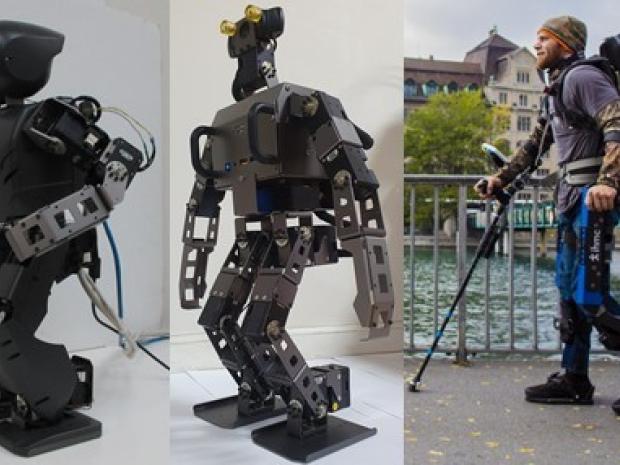
Applied Dynamics and Optimization Laboratory
We aim to establish mathematical models, quantitative criteria, and algorithmic/computational foundations toward their implementations in robotics (for design and control), biomechanical systems (for prediction and analysis), and their intersections such as lower-body wearable robots.
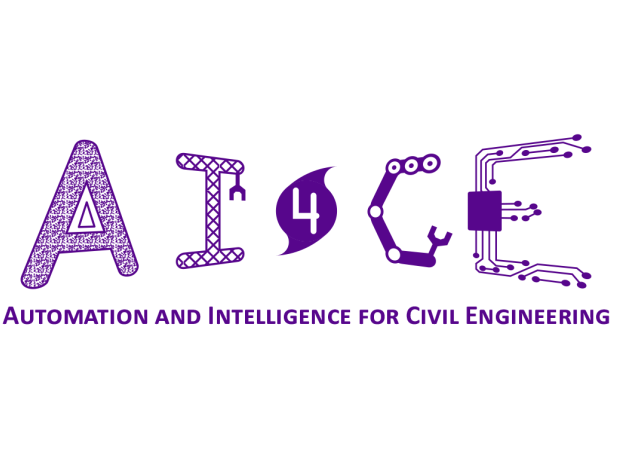
The AI4CE Lab works to advance fundamental automation and intelligence technologies, to enable their use in civil and mechanical engineering applications.
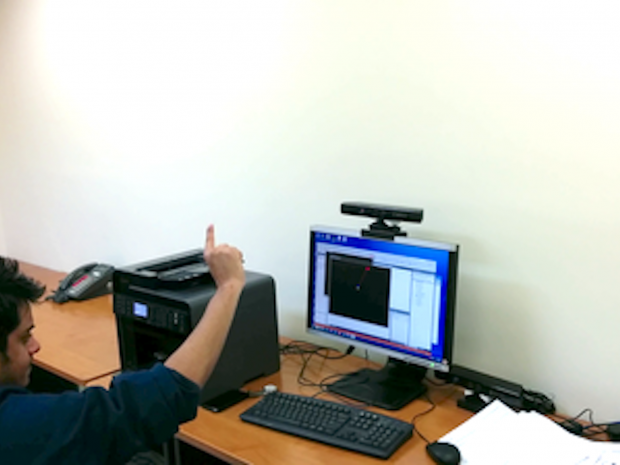
Control and Network (CAN) Lab
The CAN Lab, led by Professor Zhong-Ping Jiang, develops fundamental principles and tools for the stability analysis and control of nonlinear dynamical networks, with applications to information, mechanical, and biological systems.
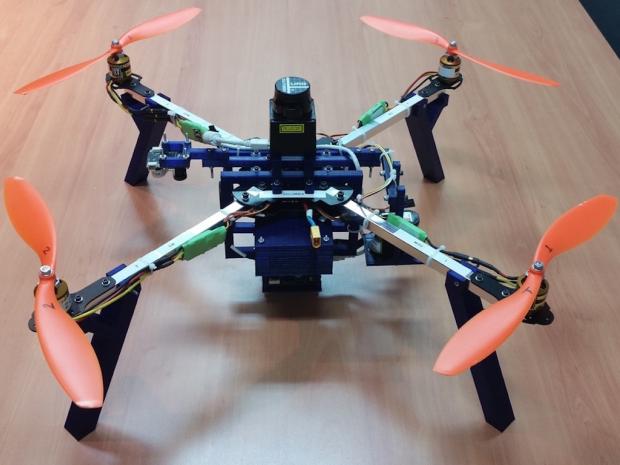
Control/Robotics Research Laboratory (CRRL)
CCRL conducts research projects on unmanned vehicles, autonomy and navigation, control systems, cyber-security, and machine learning.
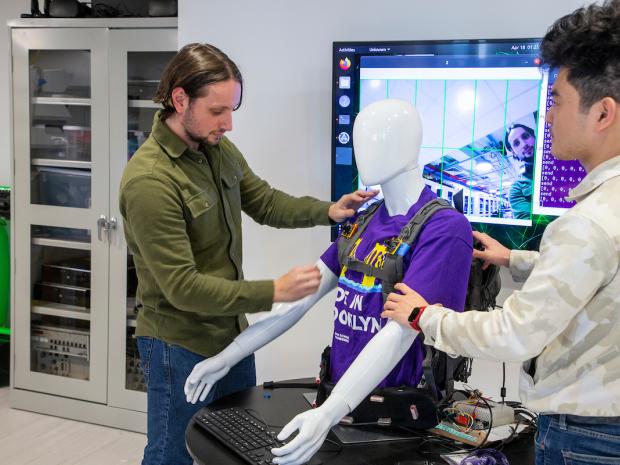
Dynamical Systems Laboratory (DSL)
Professor Maurizio Porfiri’s group conducts multidisciplinary research in the theory and application of dynamical systems, motivated by the objectives of advancing engineering science and improving society. Their theoretical expertise is in controls, networks, nonlinear dynamics, and time-series, while our application domain is in modeling and analysis of physical, social, and technical systems.

Laboratory for Agile and Resilient Complex Systems
Our goal is to develop new control and game-theoretic tools for designing agile and resilient control for smart energy systems, communication networks, secure cyber-physical systems, and human-in-the-loop systems.
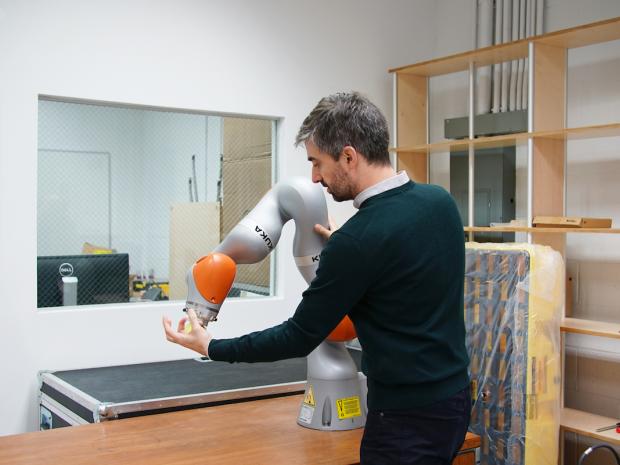
Machines in Motion
We try to understand the fundamental principles for robot locomotion and manipulation that will endow robots with the robustness and adaptability necessary to efficiently and autonomously act in an unknown and changing environment.
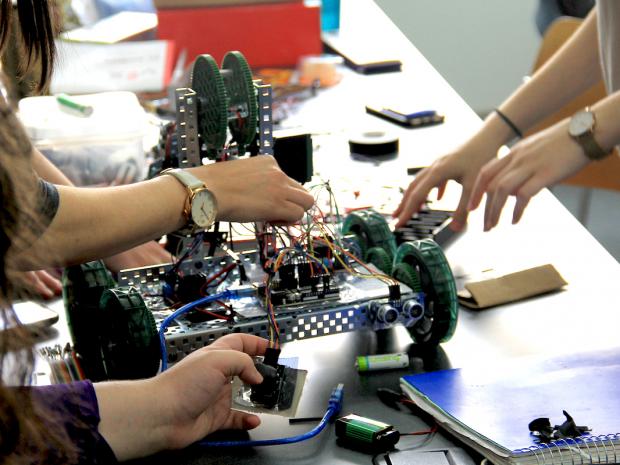
Mechatronics, Controls, and Robotics Lab
The lab provides undergraduate and graduate students a real-world, hands-on experience in modern DSP- and PC- based data acquisition and real-time control.
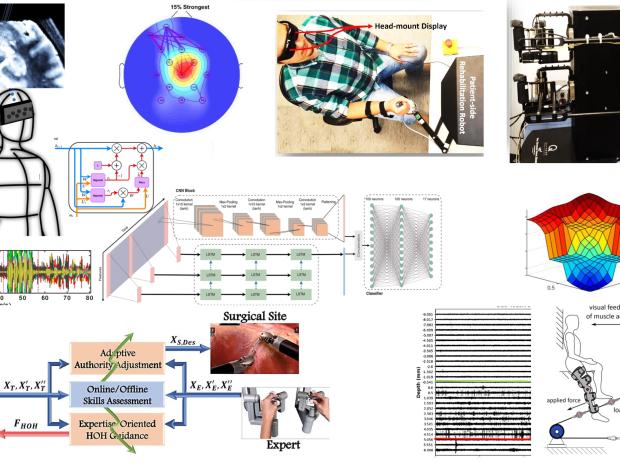
Medical Robotics and Interactive Intelligent Technologies (MERIIT)
Led by S. Farokh Atashzar, the MERIIT Lab develops and implements artificial intelligence algorithms, smart wearable hardware, advanced control systems, and signal processing modules systems to augment human capabilities using multimodal robotic technologies.
Farokh Atashzar Prof. Atashzar's research is in medical, surgical, and rehabilitation robotics. He also works on haptics, smart protheses, telerobotics, control theory, and AI. He organizes and chairs numerous workshops and symposia on these topics. Prof. Atashzar heads the MERIIT Lab.
Yi-Jen Chiang Prof. Chiang's research is in big data visualization and computation, including robot motion planning, I/O-efficient algorithms, information-theoretic data analysis and visualization, multiresolution techniques, graphics compression, computational geometry, and topology-driven visualization.
Anna Choromanska Prof. Choromanska's research is in machine learning (theory and applications), deep learning, optimization, and autonomous driving systems. Results of her work are in use by Facebook and Baidu. She has received an IBM faculty award and has been named an Alfred P. Sloan Fellow.
Chen Feng Prof. Feng's research is in computer vision and machine learning for robotics and automation. He has several patents on visual simultaneous localization and mapping. Prof. Feng's multidisciplinary research group, AI4CE, works on problems that originate from civil and mechanical engineering domains.
Zhong-Ping Jiang Prof. Jiang's current research is in learning-based control and distributed optimization/control for autonomous and nonlinear systems. He was named a Clarivate Analytics Highly Cited Researcher (2018) and is on numerous editorial boards. Prof. Jiang is the originator of robust adaptive dynamic programming which has applications to power systems, connected and autonomous vehicles, and human motor control. He is a Fellow of IEEE, IFAC, and CAA. He heads the CAN Lab.
Vikram Kapila Prof. Kapila's research is in mechatronics, robotics, smart sensors, and applications of control. He is a pioneer of mechatronics education and K-12 STEM education. Prof. Kapila has received numerous awards for teaching and innovation in education. He heads the Mechatronics Lab.
Farshad Khorrami Prof. Khorrami's research is in autonomous unmanned vehicles, smart structures, robotics, cyber-physical systems, high-speed positioning, large scale systems and decentralized control. He has multiple patents in micropositioning, vibration reduction, and actuator control. Prof. Khorrami heads the Control/Robotics Research Lab (CRRL).
Joo H. Kim Prof. Kim's research is in multibody system dynamics, optimization theory and algorithms, and control, with applications in robotics and biomechanical systems. His current interests include stability, energetics, and locomotion control of legged robots. Prof.Kim heads the Applied Dynamics and Optimization Lab.
Giuseppe Loianno Prof. Loianno's research is in aerial robotics, drones, and vision-based navigation. Much of his research has been highlighted in the media such as IEEE Spectrum (e.g., controlling a drone using eye-tracking glasses). Prof. Loianno heads the Agile Robotics and Perception Lab (ARPL).
Maurizio Porfiri In his research, Prof. Porfiri uses the theory and algorithms of dynamical systems and networks to model, analyze, and predict the behavior of environmental, social, and engineered systems. His research is frequently featured in the media. Prof. Porfiri is a Fellow of the IEEE and ASME. He heads the Dynamical Systems Lab.
Ludovic Righetti Prof. Righetti's research focuses on the control of movements for autonomous robots and he is more broadly interested in questions at the intersection of decision making, optimization, applied dynamical systems and machine learning, and their applications to physical systems. He heads the Machines in Motion Lab.
Nialah Wilson-Small Prof. Wilson-Small's research is in coordination algorithms for large collectives of simple robots, and human-drone interactions. Specifically, she is interested in how drones can use physical feedback to influence human motion, enhancing communication for novel applications.
Quanyan Zhu Prof. Zhu's research is in game theory for autonomous decision making, the design of resilient and secure cyber-physical systems, and resource allocation. His research has applications to smart and safe autonomous systems, power and transportation infrastructure security, health care economics, and public policy. Prof. Zhu heads the LARX Lab.
Selected Publications
- A Grasp-based Passivity Signature for Haptics-enabled Human-robot Interaction by S. F. Atashzar, M. Shahbazi, M. Tavakoli, R. V. Patel. The International Journal of Robotics Research (DOI) . More publications by Farokh Atashzar .
- Soft Subdivision Motion Planning for Complex Planar Robots by B. Zhou, Y.-J. Chiang, C. Yap. Proc. European Symposium on Algorithms. More publications by Yi-Jen Chiang .
- Reconfigurable Network for Efficient Inferencing in Autonomous Vehicles by S. Fang, A. Choromanska. IEEE International Conference on Robotics and Automation. More publications by Anna Choromanska .
- Real-time Soft Robot 3D Proprioception via Deep Vision-based Sensing by R. Wang, S. Wang, S. Du, E. Xiao, W. Yuan, C. Feng. More publications by Chen Feng .
- Reinforcement Learning for Vision-Based Lateral Control of a Self-Driving Car by M, Huang, M. Zhao, P. Parikh, Y. Wang, K. Ozbay, Z.-P. Jiang. International Conference on Control and Automation . More publications by Zhong-Ping Jiang .
- Augmented Reality as a Medium for Human-Robot Collaborative Tasks by S. M. Chacko and V. Kapila. IEEE/RSJ International Conference on Intelligent Robots and Systems . More publications by Vikram Kapila .
- Relative Pose Estimation of Unmanned Aerial Systems by A. Tsoukalas, A. Tzes, F. Khorrami. Mediterranean Conference on Control and Automation . More publications by Farshad Khorrami .
- Contact-dependent Balance Stability of Biped Robots by C. Mummolo, W. Z. Peng, C. Gonzalez, J. H. Kim. ASME Journal of Mechanisms and Robotics . More publications by Joo H. Kim .
- Human Gaze-driven Spatial Tasking of an Autonomous MAV by L. Yuan, C. Reardon, G. Warnell, G. Loianno. IEEE Robotics and Automation Letters . More publications by Giuseppe Loianno .
- Zebrafish Adjust Their Behavior in Response to an Interactive Robotic Predator by C. Spinello, Y. Yang, S. Macri, M. Porfiri. Frontiers in Robotics and AI . More publications by Maurizio Porfiri .
- On Time Optimization of Centroidal Momentum Dynamics by B. Ponton, A. Herzog, A. DelPrete, S. Schaal, and L. Righetti. IEEE International Conference on Robotics and Automation . More publications by Ludovic Righetti .
- Dynamic Games for Secure and Resilient Control System Design by Y. Huang, J. Chen, L. Huang, Q. Zhu. National Science Review . More publications by Quanyan Zhu .
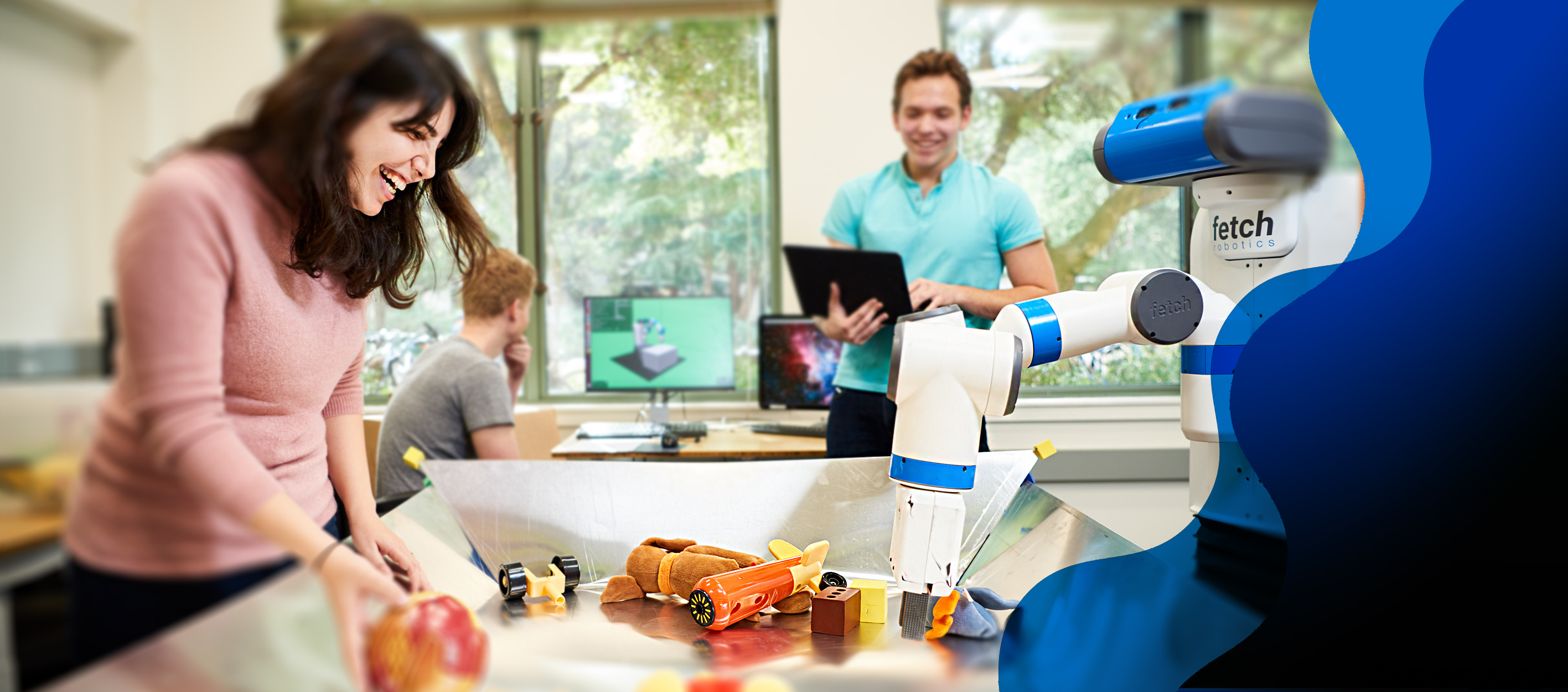
Enable robots and other agents to develop broadly intelligent behavior through learning and interaction. Exploring the intersection of machine learning and robotic control, including
- end-to-end learning of visual perception and robotic manipulation skills,
- deep reinforcement learning of general skills from autonomously collected experience,
- imitation learning,
- learning from various sources of human feedback,
- learning from interaction with other agents,
- meta-learning algorithms that can enable fast learning of new concepts and behaviors.
EE ACTIVE FACULTY | ||
ALL FACULTY | |
COURTESY FACULTY | ||
- Research & Faculty
- Offices & Services
- Information for:
- Faculty & Staff
- News & Events
- Contact & Visit
- A Message from the Chair
- Quick Facts
- Accreditation
- Undergraduate Study
- Prospective Undergraduates
- Degree Programs
- Frequently Asked Questions
- BS Curriculum
- Combined Degrees
- Honors, Electives, and Certificate Programs
- Advising and Forms
- Career Resources
- Student Organizations
- Graduate Study
- Prospective PhD Students
- How To Apply
- Prospective Master's Students
- Graduate Student Resources & Forms
- PhD Curriculum
- Master's Curriculum
- International Students
- Student Awards
- Graduate Student Society (MEGSS)
- Course Listing for Previous Years
- Courses in All Departments
- Core Disciplines
- Advanced Manufacturing
- AI and Design
- Biosystems and Health
- Computational Engineering
- Energy and Sustainability
- Micro/ Nanoengineering
- Robotics and Autonomy
- Affiliated Centers & Institutes
- Innovation & Entrepreneurship
- Core Faculty
- Administrative Faculty
- Faculty of Instruction
- Affiliated Faculty
- Advisory Board
- Faculty Awards & Honors
- Faculty Books
- News Archive
- Summer Short Course: Advanced Technologies and Tribology
- Colloquia & Seminars
- Department Email Groups
- ME Administrative Resources
- Filing Expense Reports
- Staff Resources
- Diversity, Equity, and Inclusion (DEI)
- Diversity, Equity and Inclusion Committee
- Student Groups
- Northwestern Engineering
Research / Areas of Research Robotics and Autonomy
Creating machines that interact with complex environments, make decisions, take action, and collaborate with humans and one another
View robotics and autonomy faculty
Research Area Subtopics
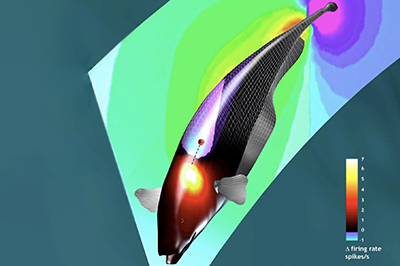

Bio-inspiration, Neuromechanics, and Neuroscience
We study sensorimotor integration in model animal systems to better understand neuromechanics and to derive inspiration for robots. We particularly seek to understand how animals move their bodies and sensory organs in order to execute behaviors, such as prey capture. Model systems include rat whisking and the electrosense of the black ghost knifefish.
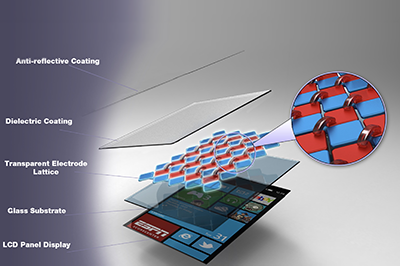
Human-machine Systems
We investigate a broad range of topics in human-machine systems including haptic interfaces to virtual environments, haptic feedback on touch surfaces, human-in-the-loop control systems to augment motor learning, human-robot co-adaptation, especially in the context of rehabilitation, and dynamic allocation of autonomy in the context of human-robot teams.
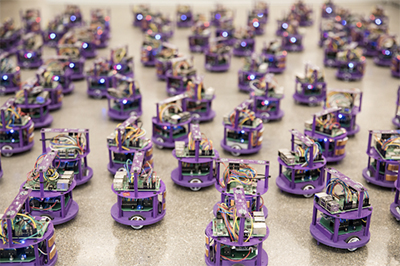
Swarm Robotics and Decentralized Computation
Networked systems often exhibit emergent behavior. In nature, for example, flocks of birds, schools of fish, and swarms of bees all develop cohesive global behavior from purely local interactions. We develop tools to design local control, communication, and estimation laws for individual agents that yield a desired group behavior such as self-assembly or locomotion.
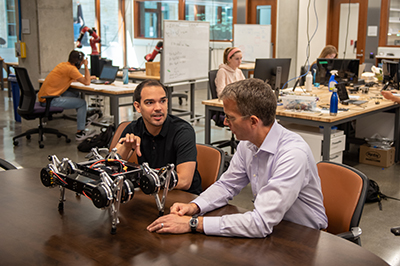
Autonomous Systems
Autonomous systems use sensory data, artificial intelligence, machine learning, and motion planning and control to make real-time decisions about how to act in changing environments. We explore new ways for robots, vehicles, and other powered devices to achieve sophisticated autonomous behaviors, including locomotion on complex terrain, multi-fingered or nonprehensile manipulation, and automation in manufacturing.
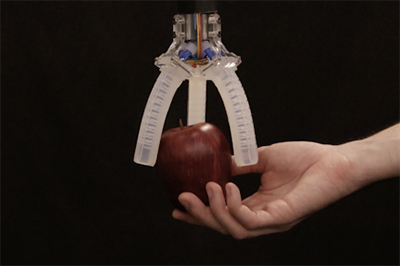
Soft Robotics
We develop soft devices and machines with novel bioinspired actuation, perception, control, and power capabilities. This work includes the synthesis and characterization of functional soft, polymeric, and nanoscale materials, the development of novel additive and digital manufacturing methods, and the design of soft robotic structures with embedded sensors, actuators, and control.
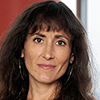
Brenna Argall
Professor of Computer Science
Professor of Mechanical Engineering
Professor of Physical Medicine and Rehabilitation
Email Brenna Argall
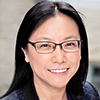
Jian Cao
Associate Vice President for Research
Cardiss Collins Professor of Mechanical Engineering and (by courtesy) Civil and Environmental Engineering and Materials Science and Engineering
Director, Northwestern Initiative for Manufacturing Science and Innovation (NIMSI)
Email Jian Cao
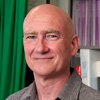
J. Edward Colgate
Walter P. Murphy Professor of Mechanical Engineering
Email J. Edward Colgate
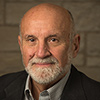
Kornel Ehmann
Professor Emeritus of Mechanical Engineering
Email Kornel Ehmann
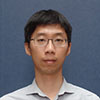
Ping Guo
Associate Professor of Mechanical Engineering
Email Ping Guo
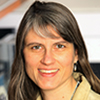
Mitra Hartmann
Professor of Biomedical Engineering
Professor of Computer Science (by courtesy)
Email Mitra Hartmann
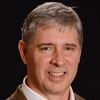
Kevin Lynch
Director, Center for Robotics and Biosystems
Email Kevin Lynch
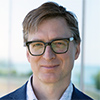
Malcolm MacIver
Email Malcolm MacIver
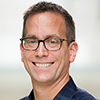
Todd Murphey
Director of the Master of Science in Robotics Program
Email Todd Murphey
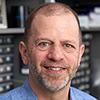
Michael Peshkin
Allen K. and Johnnie Cordell Breed Senior Professor in Design
Email Michael Peshkin
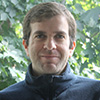
Michael Rubenstein
Associate Professor of Computer Science
Director of Graduate Admissions in Computer Science
Email Michael Rubenstein
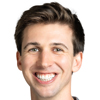
Ryan Truby
Assistant Professor of Materials Science and Engineering
Assistant Professor of Mechanical Engineering
June and Donald Brewer Junior Professor
Email Ryan Truby
Courtesy Faculty
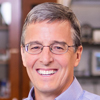
John Rogers
Louis Simpson and Kimberly Querrey Professor of Materials Science and Engineering, Biomedical Engineering and Neurological Surgery (and by courtesy Electrical and Computer Engineering, Mechanical Engineering, Chemistry and Dermatology)
Director, Querrey Simpson Institute for Bioelectronics
Email John Rogers
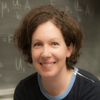
Petia Vlahovska
Professor of Engineering Sciences and Applied Mathematics (by courtesy) Mechanical Engineering
Email Petia Vlahovska
More in this section
Mechanical Engineering
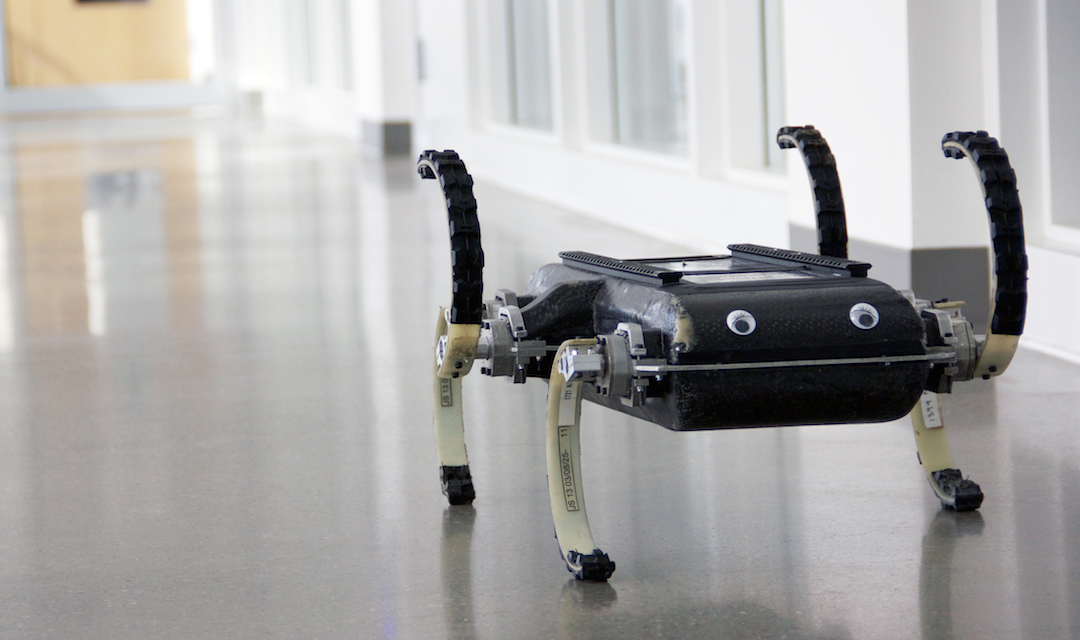
Our experts are creating the next generation of robotics for human interaction across disciplines.
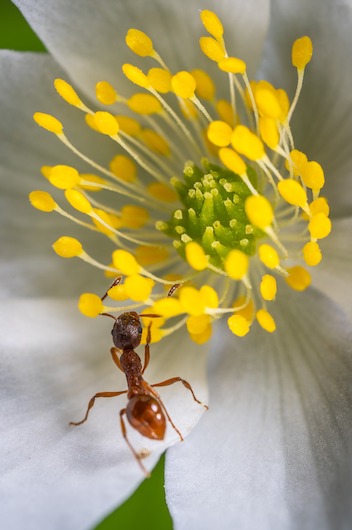
Bioinspired robotics
Natural organisms have amazing abilities that inspire the field of robotics. Our researchers are investigating how we can replicate a leafhopper’s jump in a robot, what we can learn from the way cockroaches move, and how we can create robots and biohybrid robotic systems that are as robust and adaptable as animals.
Faculty involved: Sarah Bergbreiter , Aaron Johnson , Victoria Webster-Wood , Inseung Kang , Ophelia Bolmin
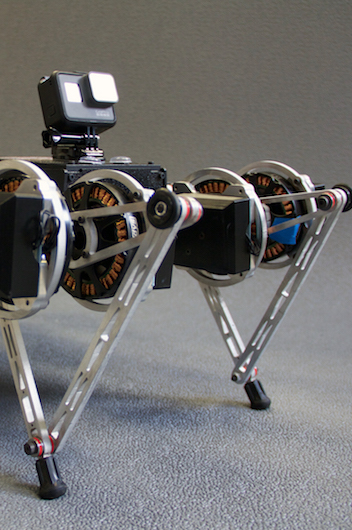
Robots that walk, run, jump, creep, roll, and fly
Researchers explore legged robotics to design better controllers for stable, energy efficiency, fast locomotion as well as the ability to reliably travel over unstructured terrain - like the moon.
Our faculty are creating technologies for infrastructure inspection, aerial load transportation, agricultural monitoring, and autonomous point-to-point flight.
Faculty involved: Sarah Bergbreiter , Aaron Johnson , Kenji Shimada , Inseung Kang
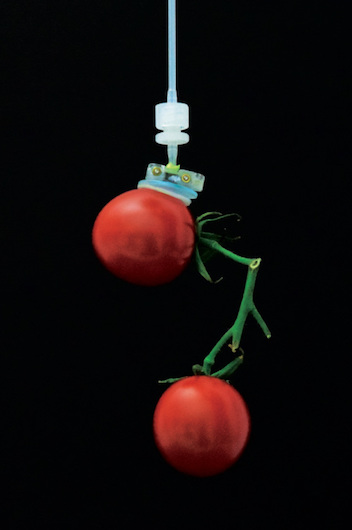
Micro robotics and biomedical applications
Through multidisciplinary collaboration, our faculty are fabricating magnetic micro swimming robots assembled from DNA nanostructures, controlling robots that walk on water and climb walls with nano-fiber adhesives, and designing devices for microsurgery.
Faculty involved: Sarah Bergbreiter , Burak Ozdoganlar , Rebecca Taylor , Victoria Webster-Wood , Inseung Kang , Ophelia Bolmin
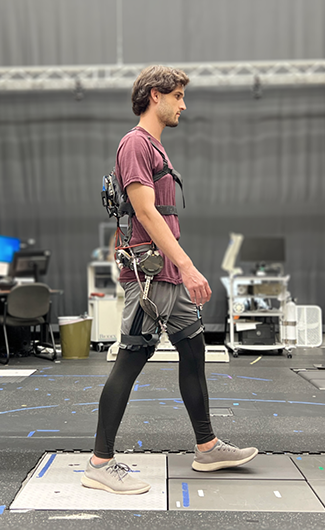
Assistive robotics and wearables
Wearable robotic systems can have a transformative impact on human mobility. Our researchers are developing robotic exoskeletons for clinical populations and building portable systems for precision rehab. By combining data from wearable sensors with artificial intelligence they are trying to understand what movement patterns are associated with disease progression.
Faculty involved: Eni Halilaj , Inseung Kang
Learn more.... "Precision rehabilitation to prevent osteoarthritis"
Special tasks: Human help
Whether it's maneuvering through debris after a hurricane, or helping a person with physical limitations chop vegetables, robots can perform tasks that are difficult and dangerous for people.
Faculty involved: Amir Barati Farimani , Aaron Johnson , Kenji Shimada , Ding Zhao , Inseung Kang
Learn who in Mechanical Engineering is working in advanced manufacturing .
Related News
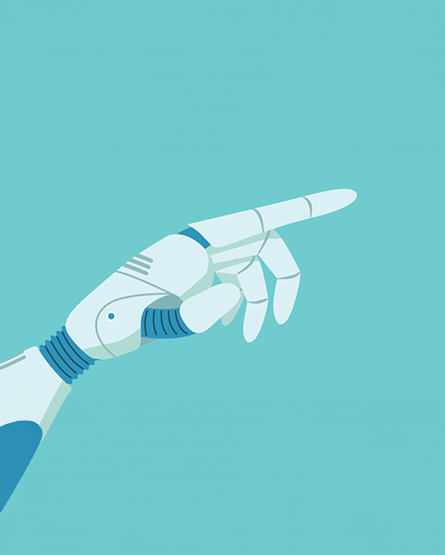
Carnegie Mellon core partner in new center to improve robot dexterity selected to receive up to $52 million Opens in new window
Carmel Majidi will lead a research thrust in a new multi-institutional collaboration to launch an NSF ERC dedicated to revolutionizing the ability of robots to amplify human labor.
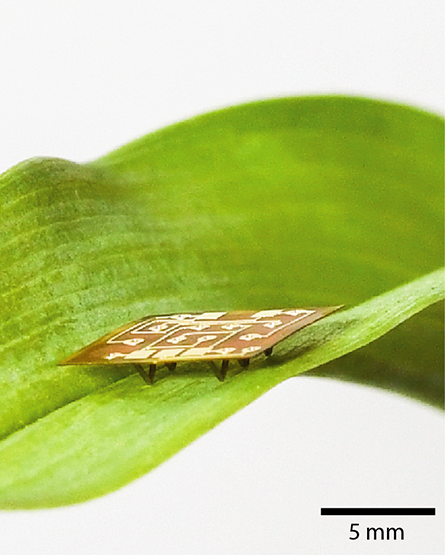
It’s an ant… it’s a robot… it’s Picotaur! The unrivaled micro robot Opens in new window
Researchers in the Department of Mechanical Engineering at Carnegie Mellon University have created the first legged robot of its size to run, turn, push loads and climb miniature stairs.
- Watch the Video Opens in new window
- Research paper Opens in new window
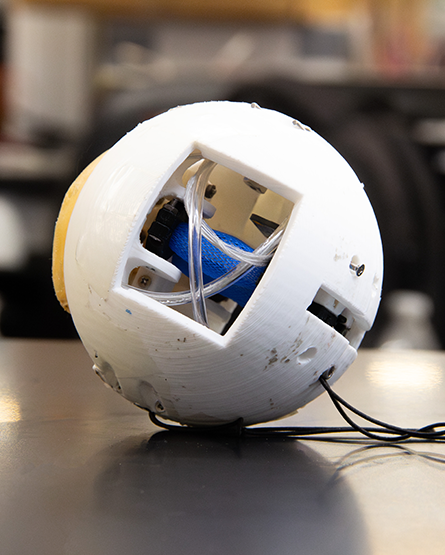
Sea slugs: what can we learn from them? Opens in new window
Studying Aplysia californica, or sea slugs, can tell us a lot about neuromuscular systems and open up avenues for more controlled animal experiments.
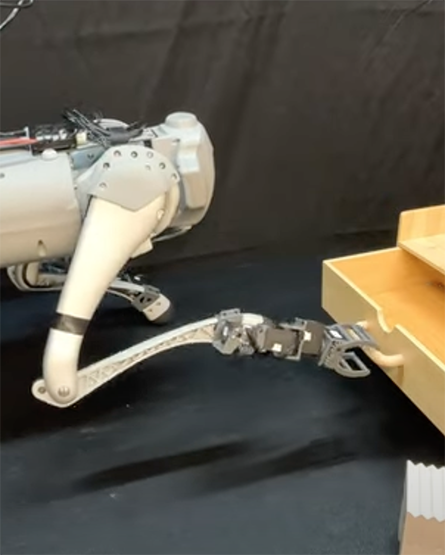
Manipulation technology makes home-helper robot possible Opens in new window
New locomotion and manipulation technology from Ding Zhao’s lab will enable four-legged robots to lend a hand in the not-so-distant future.
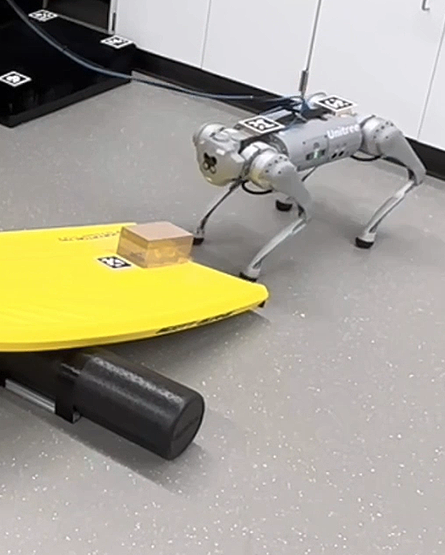
RoboTool enables creative tool use in—you guessed it—robots Opens in new window
Large language models enable robots to “brainstorm” creative tool use and perform seemingly impossible tasks.
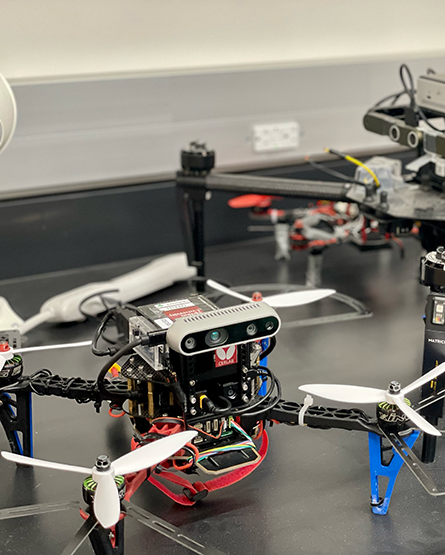
Drones CAN navigate dynamic environments Opens in new window
Novel drone navigation technology developed by Kenji Shimada was put to the test in an active Japanese tunnel construction site, enabling drones to approximate where a collision may occur and prevent it.

Big picture, small robot Opens in new window
A team of mechanical engineering researchers create “Mugatu,” the first steerable bipedal robot with only one motor.
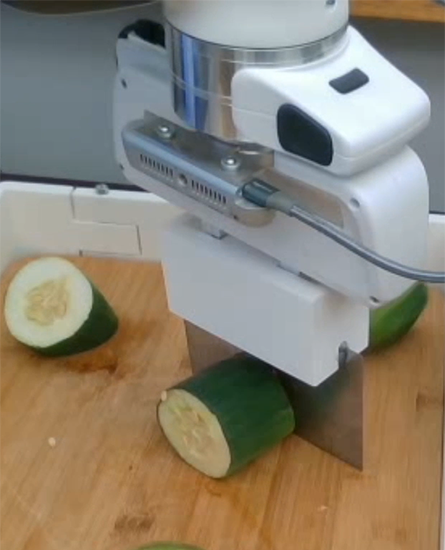
Chop, chop: Improving food prep with the power of AI Opens in new window
Researchers at CMU combined two vision foundational models—models trained on large visual data sets—to help a robot arm recognize the shape and the type of fruit and vegetable slices.
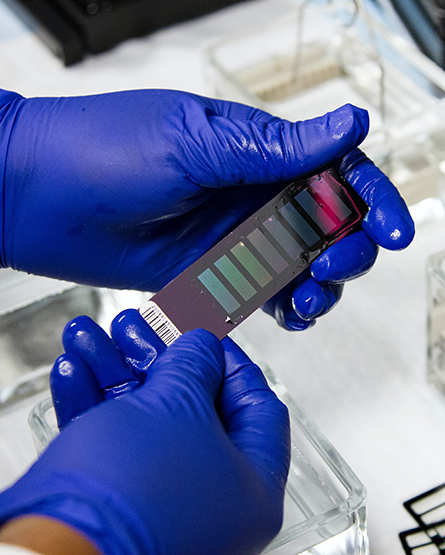
Dowd Fellowship encourages ambitious student research Opens in new window
Four Ph.D. students in the College of Engineering have received funding to pursue research on valuable, relatively unexplored topics.
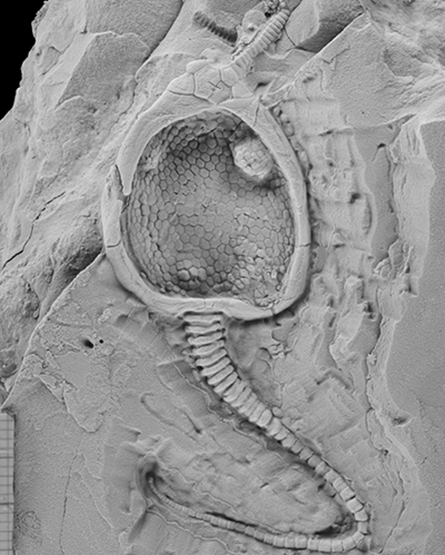
450-million-year-old organism finds new life in Softbotics Opens in new window
Researchers in the Department of Mechanical Engineering used fossil evidence to engineer a soft robotic replica of pleurocystitids, a marine organism that existed nearly 450 million years ago and is believed to be one of the first echinoderms capable of movement using a muscular stem.
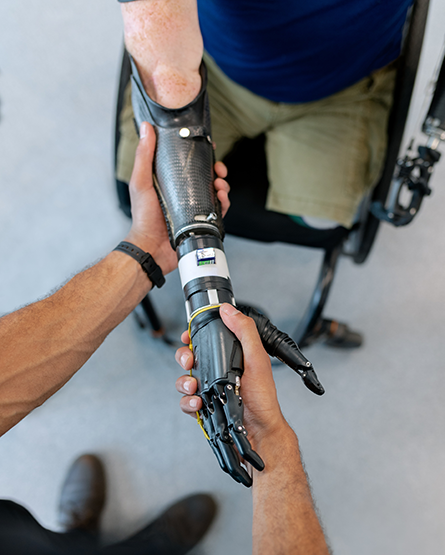
A new course for prosthetics care Opens in new window
Between his studies, a teaching opportunity, and nonprofit work, mechanical engineering Ph.D. student Jonathan Shulgach looks to reimagine the experience of receiving medical care by bringing patients closer to the process.
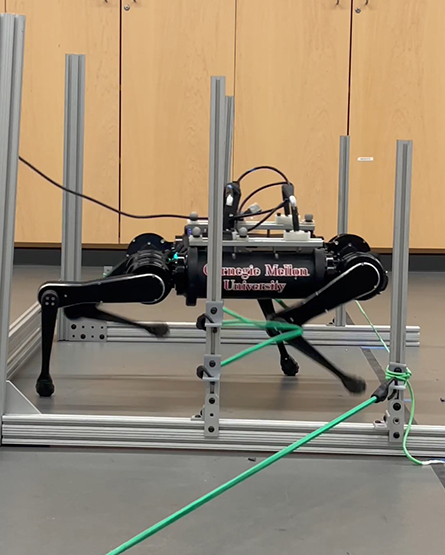
Tricky tangles: Robots learn to navigate vine-like vegetation Opens in new window
CMU researchers develop technology that enables four-legged robots to walk through vine-like vegetation.
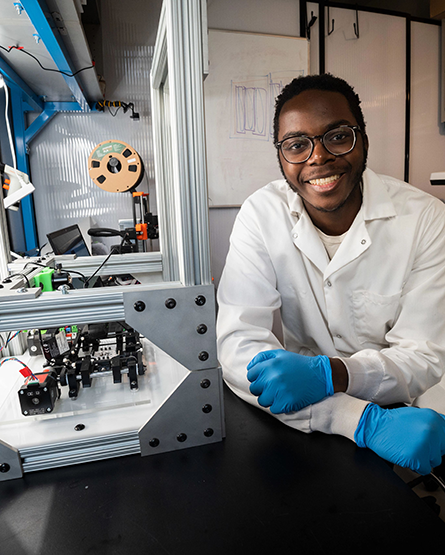
Student works on tiny bio robots Opens in new window
Lameck Beni, an undergraduate student in mechanical engineering and biomedical engineering, conducted research on small biodegradable robots that have important medical applications.
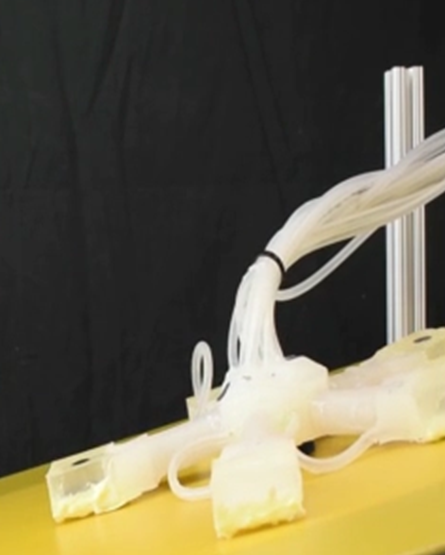
So tricky, a robot can do it Opens in new window
Carnegie Mellon Researchers have taken inspiration from geckos to create a material that adheres to wet and dry surfaces, even on an incline.
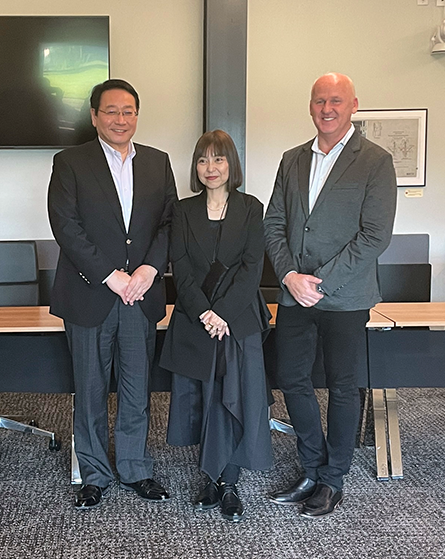
Virtual reality partners become real world neighbors Opens in new window
In July, the YKK AP Technologies Lab opened at Mill 19. Their focus on the development of a virtual factory is well aligned with the digital twin work underway at the Manufacturing Futures Institute.
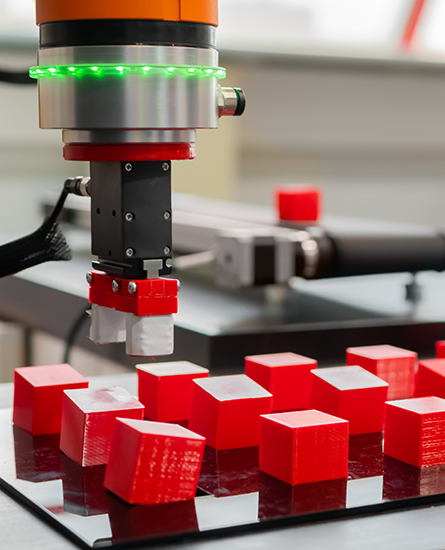
Training robotic arms with a hands-off approach Opens in new window
Researchers at Carnegie Mellon University recently trained a robotic arm with human movements generated by artificial intelligence.
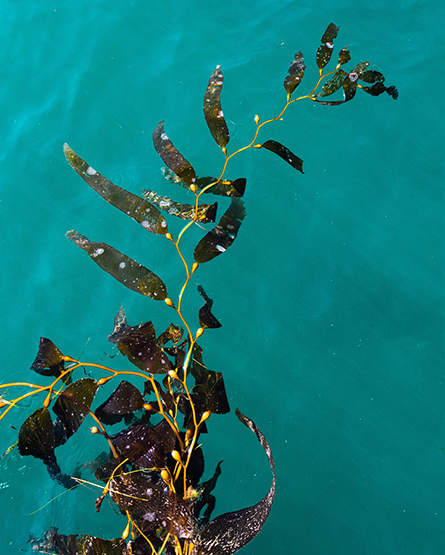
Now printing: seaweed-based, biodegradable actuators Opens in new window
We are one step closer to naturally compostable robots now that researchers at Carnegie Mellon can print actuators using a bio-ink made from seaweed.
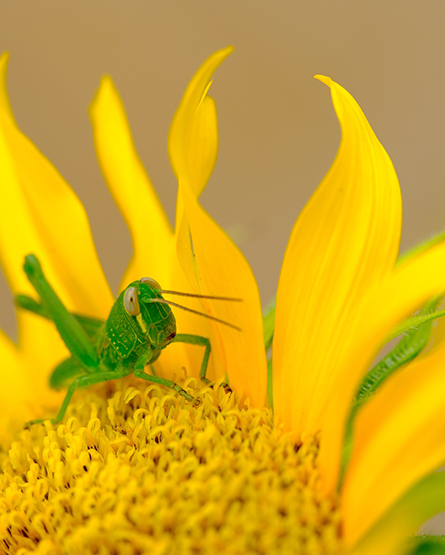
Grasshopping robots made possible with new, improved latch control Opens in new window
Researchers at Carnegie Mellon University have made grasshopping robots possible by uncovering that latches can mediate energy transfer between robotic jumpers and the environment that they are jumping from.
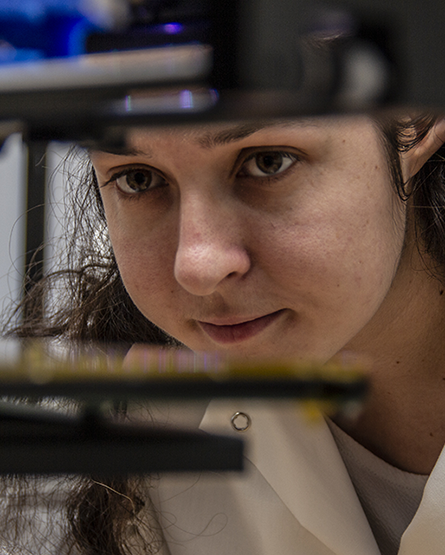
Multi-university team building actuators for next gen bio-bots Opens in new window
Multi-university team, led by Mechanical Engineering’s Vickie Webster-Wood, is building actuators for next generation sustainable bio-bots.
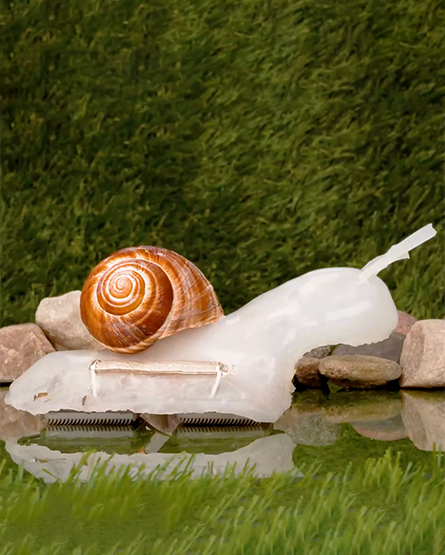
Engineering breakthrough in softbotics Opens in new window
Introducing the first soft material that can maintain a high enough electrical conductivity to support power hungry devices.
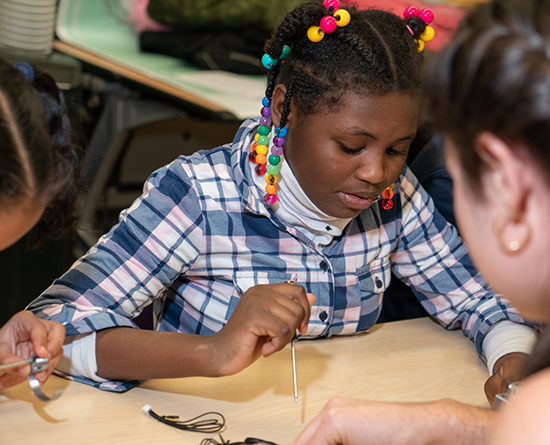
Gwen’s Girls partnership fosters children’s interest in robomechanics Opens in new window
A student-driven partnership between Aaron Johnson’s RoboMechanics lab and Gwen’s Girls has introduced nearly 100 grade school girls to engineering design and robotics.
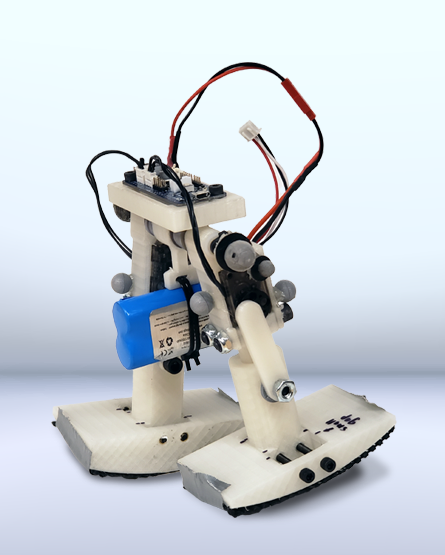
Big steps for mini robots Opens in new window
Aaron Johnson’s Robomechanics Lab tested spherical foot designs to find the best fit so their biped robot with 15-cm legs could walk steadily.
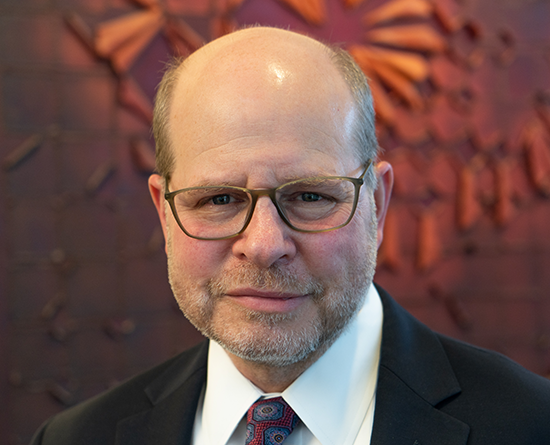
Jon Cagan named head of Mechanical Engineering Opens in new window
Jon Cagan will be the new head of the Department of Mechanical Engineering at Carnegie Mellon University, effective November 1, 2022.
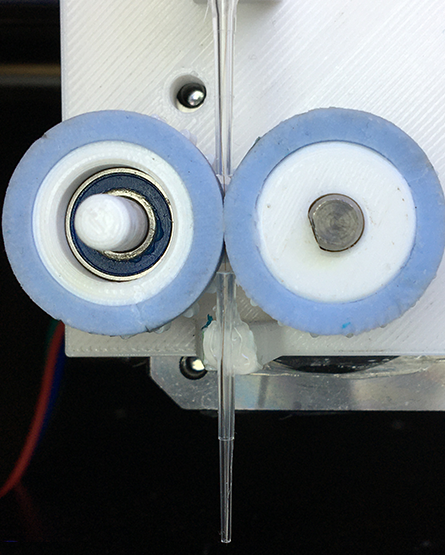
Hydrogels pave way for future of soft robotics Opens in new window
Wenhuan Sun, Victoria Webster-Wood, and Adam Feinberg have created an open-source, commercially available fiber extruder to benefit future research with hydrogels and soft robotics.
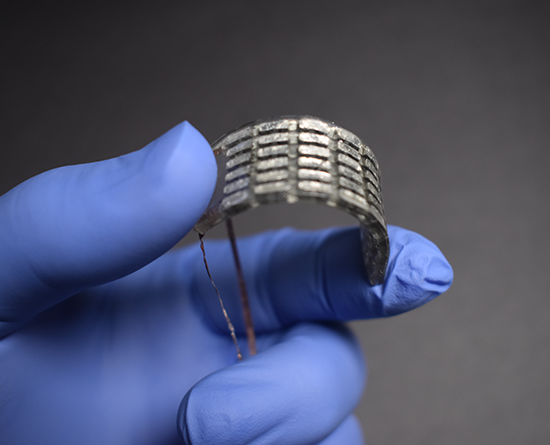
A cooler side to soft robotics Opens in new window
Researchers combined liquid crystal elastomers with a thermoelectric device to develop a stretchable transducer for soft robotics.
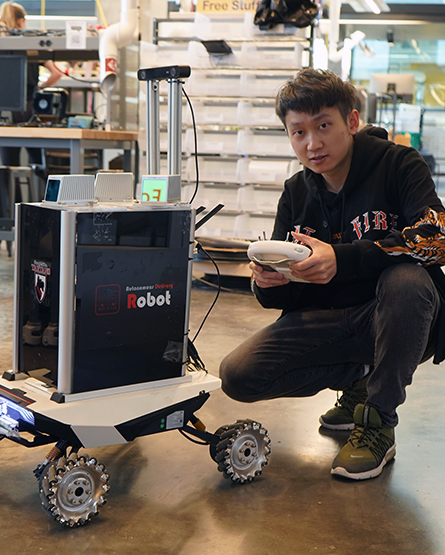
Robots that can learn to safely navigate warehouses Opens in new window
Advances in chips, sensors, and AI algorithms are enabling robots to continuously learn how to plan routes, avoid obstructions, and operate safely in large dynamic warehouse environments.
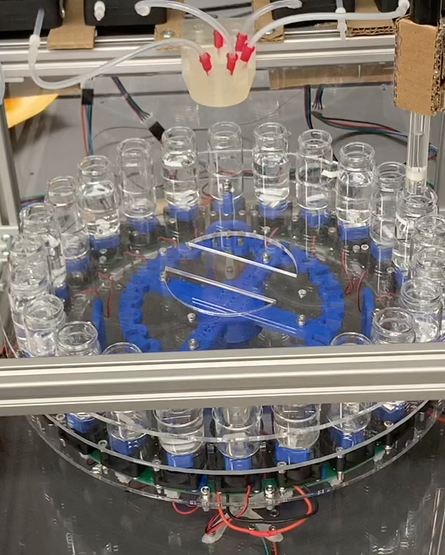
Students’ ingenuity automates scientific research Opens in new window
In an effort to modernize scientific research, CMU students used only commercial parts and the cardboard boxes they came in to build a modular robot able to autonomously conduct experiments.
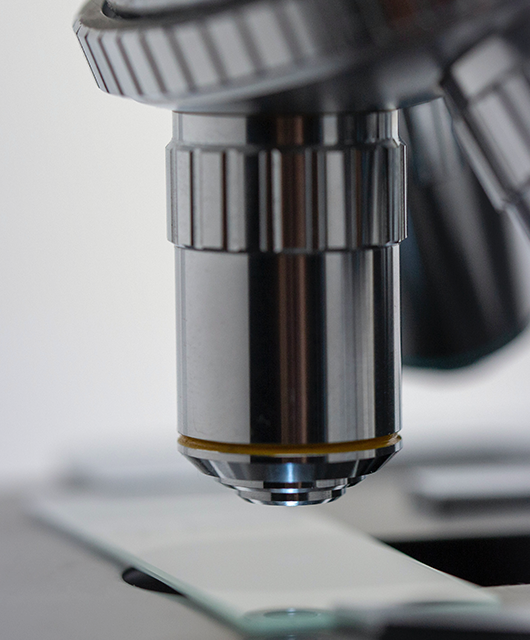
Tuning synthetic collagen threads for biohybrid robots Opens in new window
Researchers in Victoria Webster-Wood’s Biohybrid and Organic Robotics Group are using techniques from tissue engineering to refine tendon-like collagen threads for a new generation of robots.
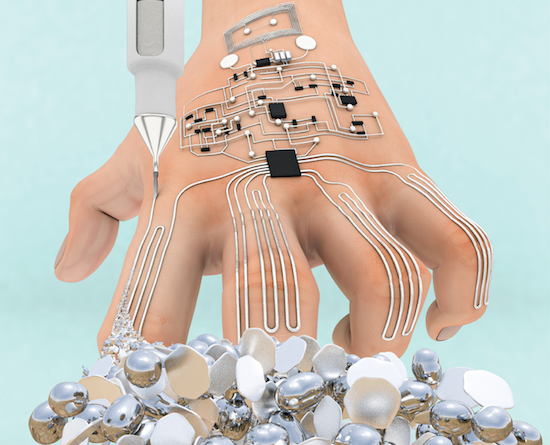
Innovative ink for stretchable circuits
A collaboration with CMU-Portugal introduces a unique printable ink that allowed, for the first time, digital printing of multi-layer stretchable circuits, e-skins, and adhesive medical patches for electrophysiological monitoring.
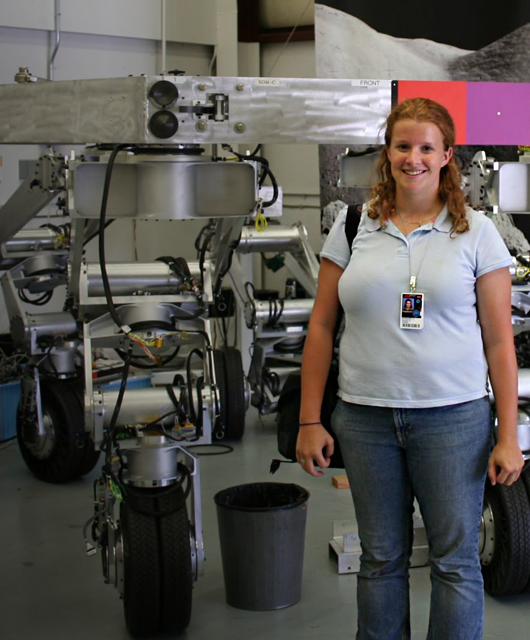
Rocket girl Opens in new window
Alumna Maggie Scholtz is co-founder and vice president of engineering at First Mode, a Seattle-based engineering firm focused on tackling challenging problems on Earth and throughout the solar system.
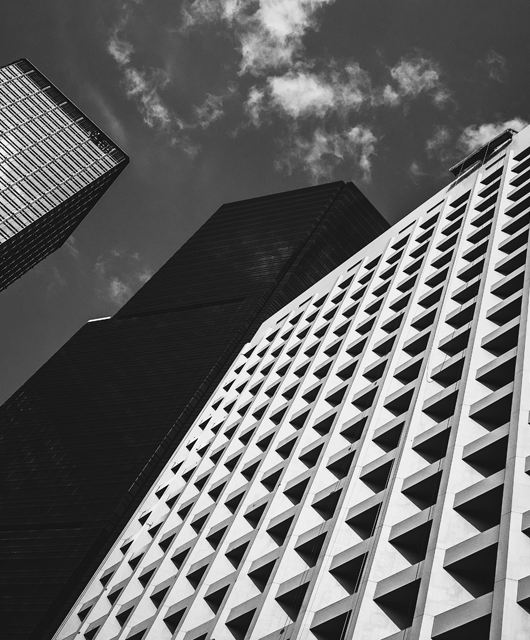
Bringing students into the next industrial revolution Opens in new window
Students taking a new course combine rapidly expanding technologies to create innovative solutions for real societal problems.
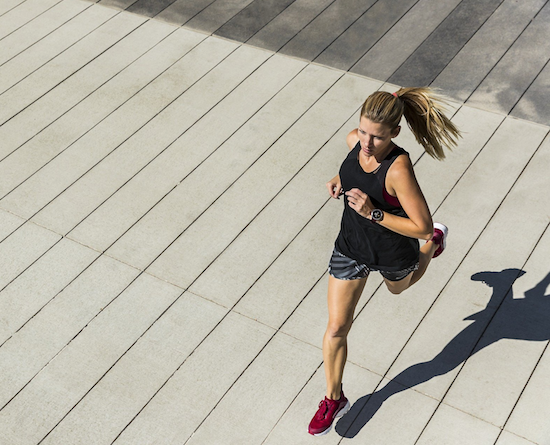
Biomechanics for teens
National Biomechanics Day is a worldwide celebration that strives to bring the complex world of biomechanics to high school students through hands-on activities, demonstrations, and Q&A sessions with real-world professionals.
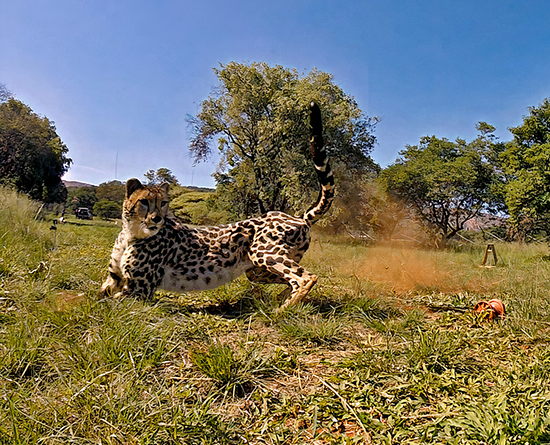
Tailing new ideas Opens in new window
Aaron Johnson’s Robomechanics Lab is looking to nature for robotic tail designs that make orientation tasks easier for moving robots.
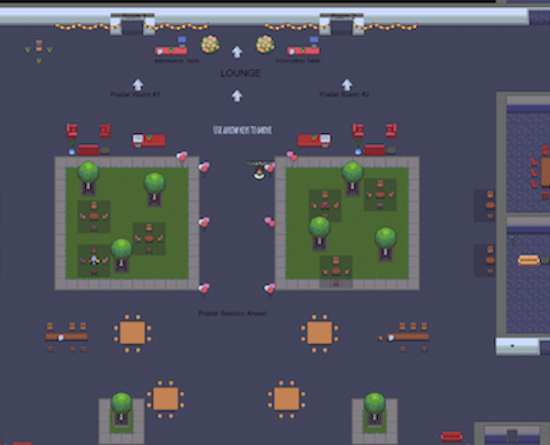
Virtually awarded
MechE graduate students earned accolades at the department’s annual research symposium poster sessions. This year's events were virtual through Gather Town, an interactive, video-chat world.
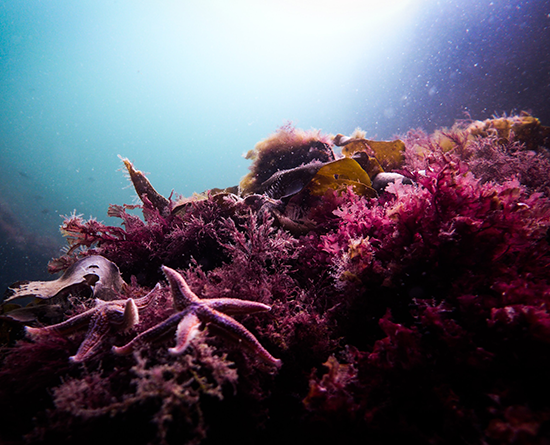
Under the sea Opens in new window
A team of researchers from Carnegie Mellon’s Soft Machines Lab has created a soft robot inspired by the quick and agile brittle star, the first mobile and untethered underwater crawling robot.
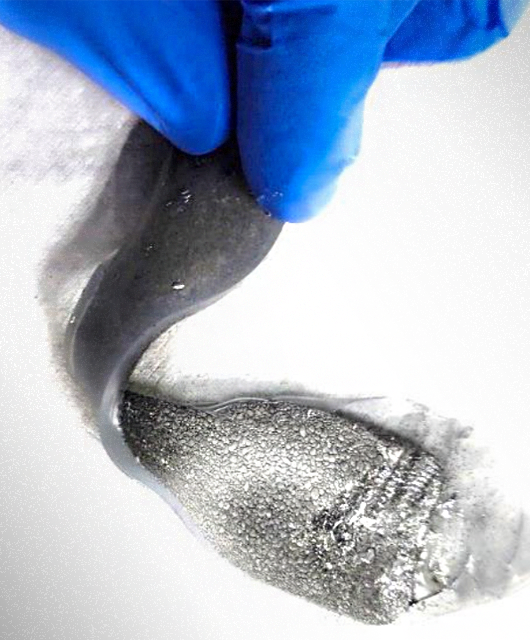
Making mechanical skin Opens in new window
These 3D printed circuits are self-healing, re-writable, and energy-harvesting, thanks to liquid metal.
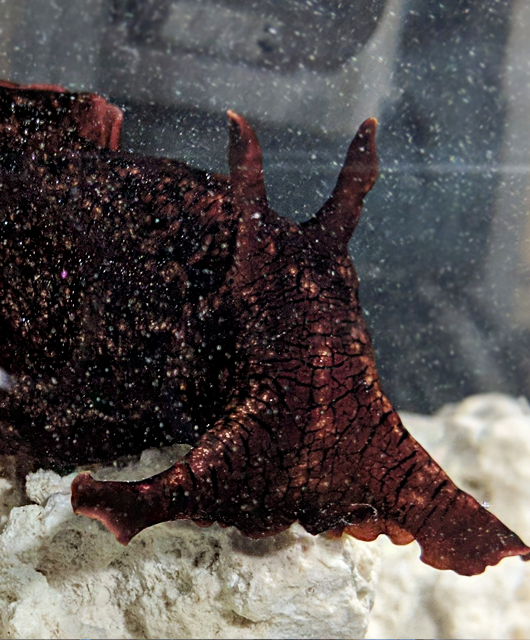
Sea slugs to provide clues in understanding the brain Opens in new window
As the co-principal investigator on an NSF NeuroNex project, Victoria Webster-Wood will investigate the impact of neuromodulators on muscle actuation and modeling biological motor control in engineering frameworks.
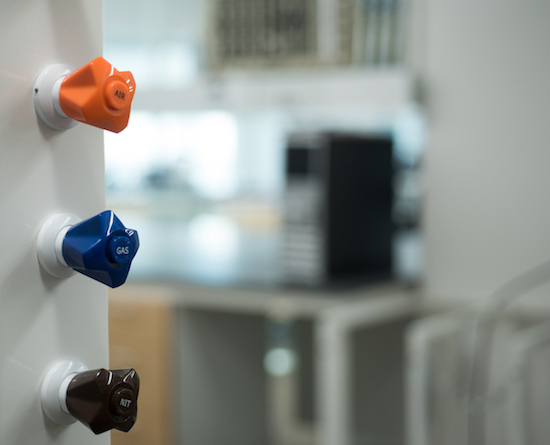
At home with research
Despite the coronavirus shutdown, researchers and students were creative about continuing their work and joining the fight against COVID-19.
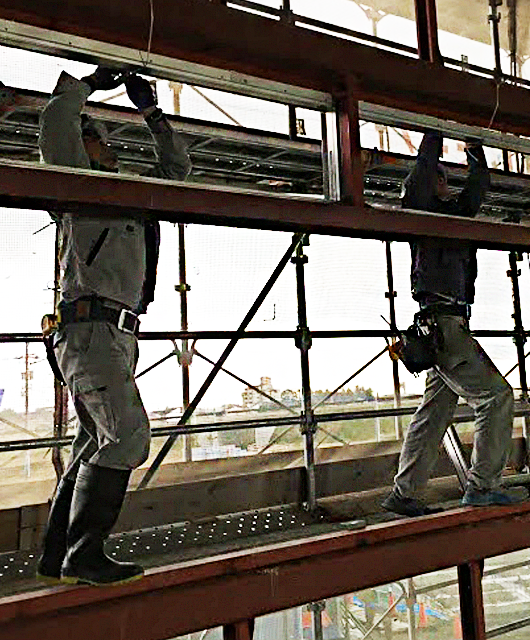
Installing windows with help from robots Opens in new window
Kenji Shimada is working on a collaboration with YKK AP to create high-tech window installation robots.
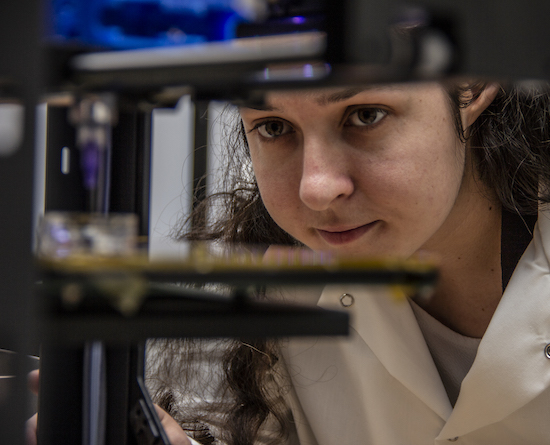
We have a Wimmer
As a 2020-2021 Wimmer Faculty Fellow, Victoria Webster-Wood plans to design interactive demonstrations and to build virtual labs for the course “Gadgetry: Sensors, Actuators, and Processors.”
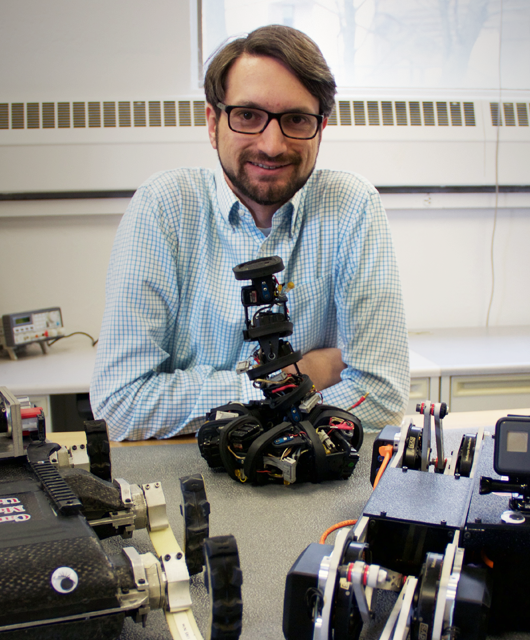
Johnson received NSF CAREER Award Opens in new window
Aaron Johnson has been awarded a CAREER award by the National Science Foundation (NSF).
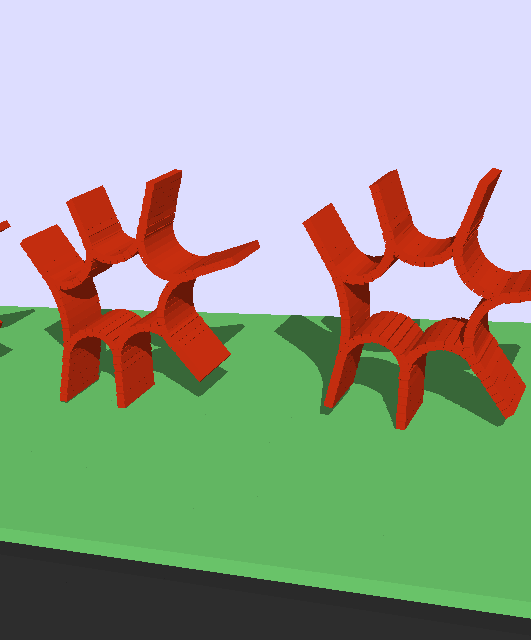
First real-time physics engine for soft robotics Opens in new window
Collaborators have adapted the sophisticated computer graphics technology used in blockbuster films and video games to simulate the movements of soft, limbed robots for the first time.
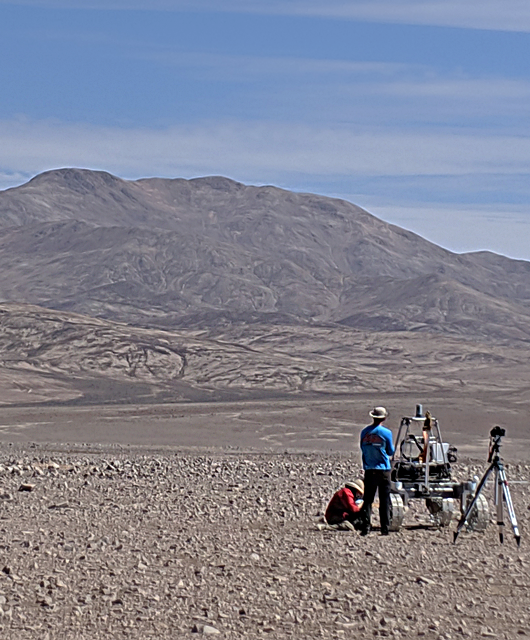
Making tracks in the desert Opens in new window
Catherine Pavlov, a Ph.D. candidate in mechanical engineering, traveled to the Atacama Desert to conduct experiments she modeled that aim to gain non-grasping functionality from space rovers.
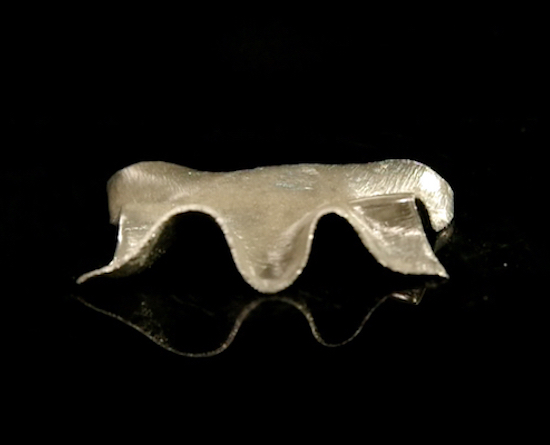
Almost natural
A step closer to integrated artificial muscle and nervous tissue, researchers develop an intelligent, shape-morphing, and self-healing material for soft robotics and wearable electronics.
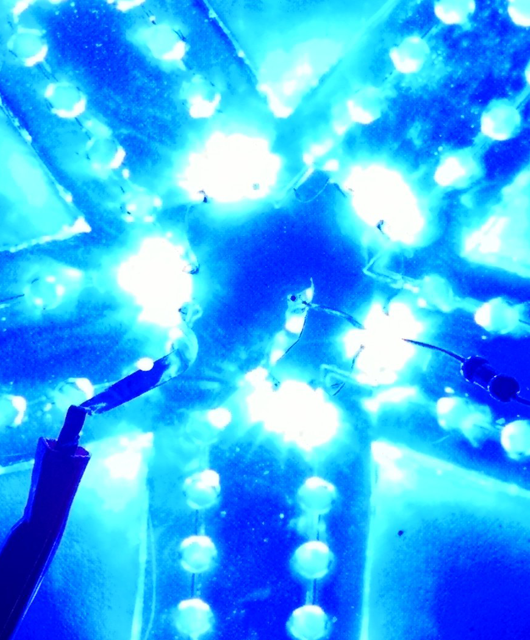
Giving robots a “nose” Opens in new window
A team of CMU researchers are developing soft robots that sense and respond to chemicals.
- Related Article Opens in new window
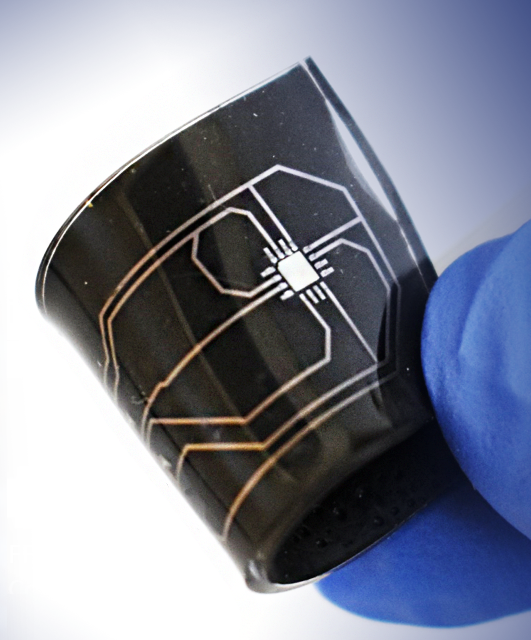
An elegant solution to the soft sensing challenge Opens in new window
Carmel Majidi’s team has developed a soft magnetic skin with a single sensing element that detects force and contact.
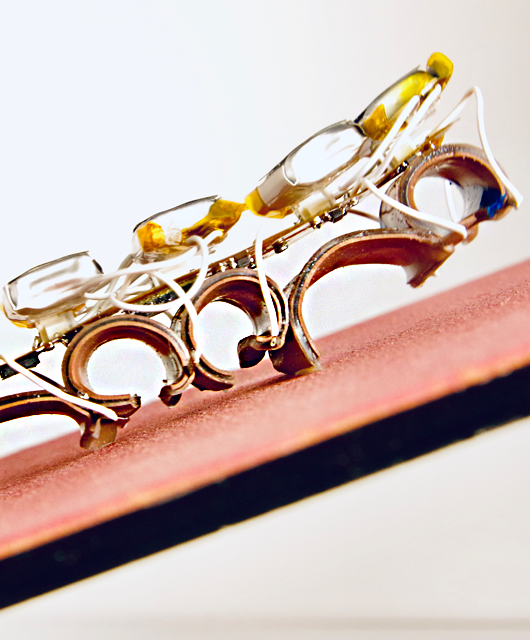
Run, robot, run! Opens in new window
Soft robots can mimic a critter’s scurry, thanks to shape memory alloy actuators.
- Read the paper Opens in new window
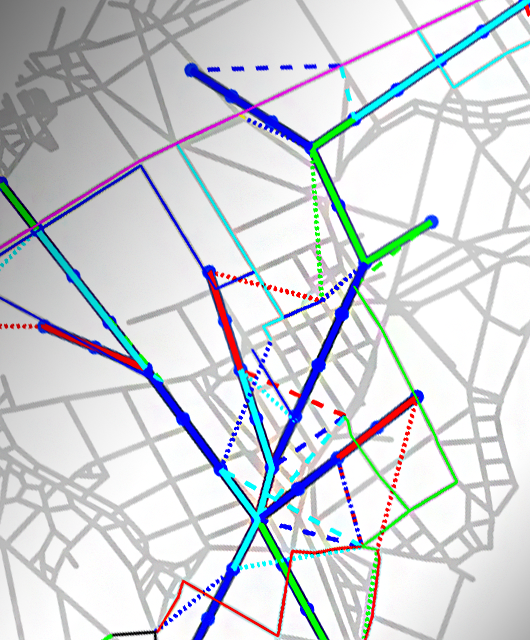
Using drones in agriculture and irrigation Opens in new window
Professor of Mechanical Engineering Kenji Shimada and his team of researchers are using drone technology to help detect and restore damaged water canals in Japan that are critical for the agricultural economy.
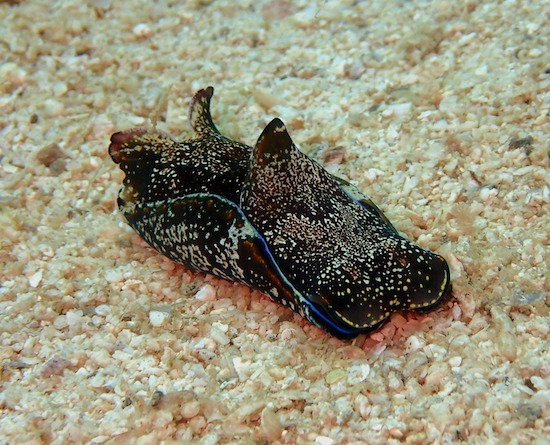
From bioinspired to biohybrid
Victoria Webster-Wood uses organic materials to build robotic devices for future applications in medicine and environmental science.
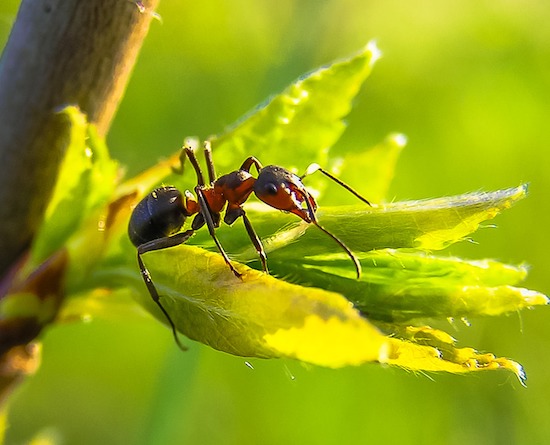
Merging microsystems and robotics
Sarah Bergbreiter develops ant-sized robots and microsystems in the Department Mechanical Engineering at Carnegie Mellon University.
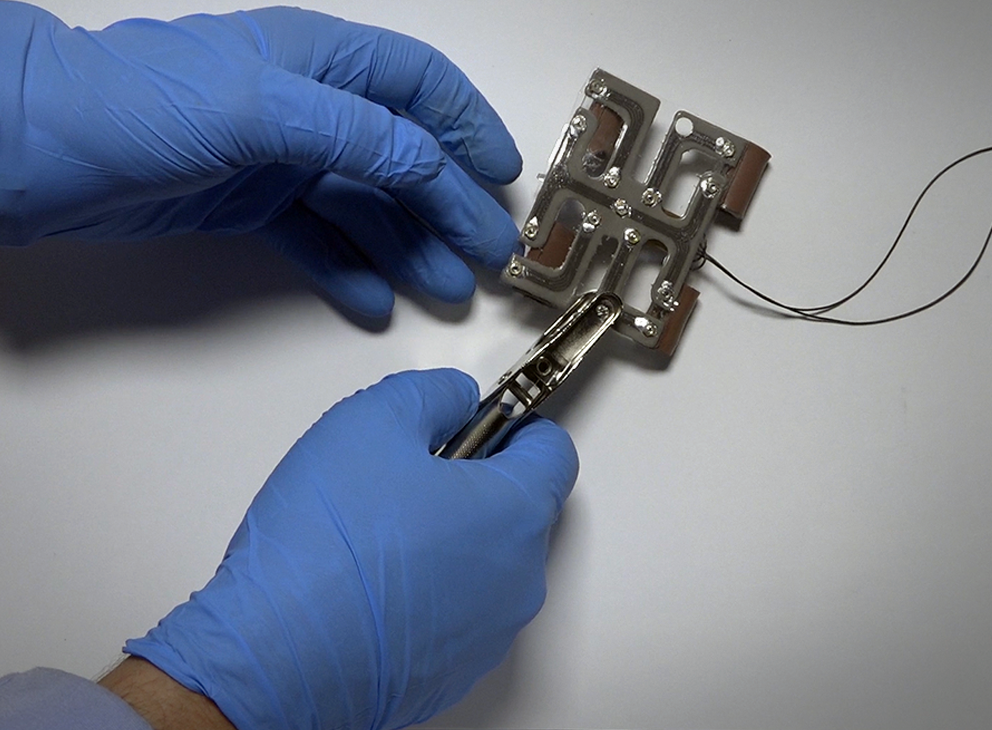
Circuit, heal thyself! Opens in new window
When punctured or torn, this material’s circuits can autonomously—and instantaneously—heal themselves to remain fully operational.
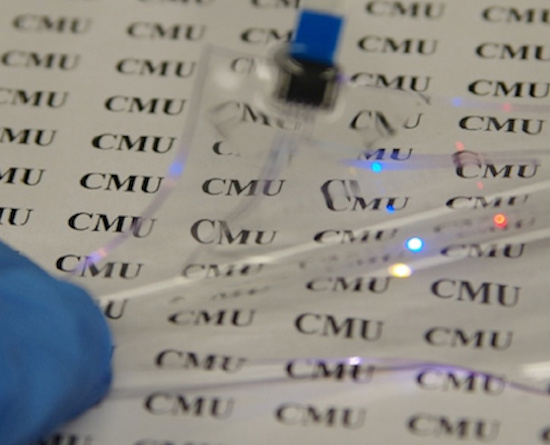
Invisible, stretchable circuits
Next-generation technologies for wearable computing, soft bioelectronics, and biologically-inspired robotics will require transparent conductors that are soft, elastic, and highly stretchable. Majidi's team is developing these invisible circuits.
Explore Other Research Topics
- Advanced manufacturing
- AI and Machine Learning
- Bioengineering
- Design methods and automation
- Energy and environment
- Micro/nanoengineering
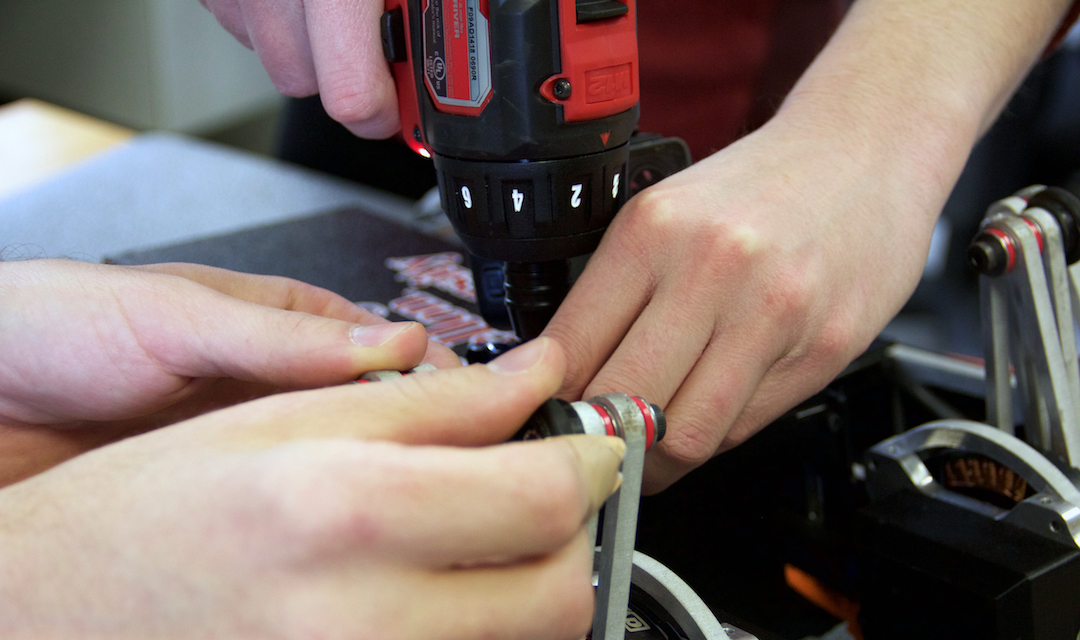
Robotics Institute
The Robotics Institute
featured research institute
Learn more about the Robotics Institute.
Robotics and Intelligent Systems Certificate Program
Topics for research in robotics and intelligent systems.
General areas for study and research:
Chemical and Biological Engineering
- Control of chemical and biological dynamic processes
- Optimal design of systems for material processing
- Manipulation of matter at atomic and molecular scale
Civil and Environmental Engineering
- Structural health monitoring and adaptive structures
- Water resources
- Earthquake detection and protective design
- Remote sensing of natural resources
- Urban planning and engineering
Computer Science
- Theory and practice of computation for physical systems
- Game playing, photo identification, and semantic identification
- Real-time algorithms for measurement, prediction, and control
- Artificial intelligence and machine learning
- Databases, Internet security, and privacy
Electrical Engineering
- Information theory
- Electricity, microelectronics, and electromagnetism
- Digital circuits and computation
- Image processing, face, and character recognition
- Video analysis and manipulation
- Telecommunications networks
- Autonomous vehicles
Mechanical and Aerospace Engineering
- Robotic devices and systems
- Autonomous air, sea, undersea, and land vehicles
- Space exploration and development
- Intelligent control systems
- Biomimetic modeling, dynamics, and control
- Cooperating robots for manufacturing and assembly
- Cooperative control of natural and engineered groups
- Identification of dynamic system models
- Optimal state estimation and control
Operations Research and Financial Engineering
- Intelligent transportation systems
- Financial management and risk analysis
- Dynamic resource management
- Decision science
- Optimal design
- Knowledge, reasoning, and language
- Logic and metaphysics
- Politics and art of robotics and intelligent systems
- Inference, reasoning, problem solving
- Human factors and human-machine interaction
- Human motor control
- Modeling perception
- Neural network (connectionist) modeling of cognitive functions
- Reinforcement learning
- Study of brain function using functional magnetic response imaging, electrical, and optical methods

- University of Southern California
- Viterbi School of Engineering
Robotics is an interdisciplinary field that involves mechatronic design, control, motion planning, computer vision, machine learning and among others. The goal of robotics is to design and develop machines that can help and assist humans in many situations and for many purposes including manufacturing processes, dangerous environments (including inspection of radioactive materials, bomb detection and deactivation), or where humans cannot survive (e.g. in space, underwater, in high heat, and clean up and containment of hazardous materials and radiation). Today, robotics is a rapidly growing field, as technological advances continue; researching, designing, and building new robots serve various practical purposes, whether domestically, commercially, or militarily.
Research in Robotics at the USC Viterbi Department of Aerospace and Mechanical Engineering spans many different areas including motion planning, robot learning and control, robot manipulation, bio-inspired robotics, and human-robot collaboration. Browsing through the websites of our faculty and research labs, you will find a wide range of applications where robotics research in our department is enabling scientific discovery and technological breakthroughs, such as: motion planning for high degrees of freedom robotic systems; physics aware decision making for multi-robot systems; motion planning and task planning for mobile manipulators; robotic additive manufacturing; robotic composite layup; human robot collaboration on assembly operations; design and control of different bio-inspired robots such as quadruped robots, bipedal robots, starfish robots, robotic birds, micro flying robots, etc.; motion planning and control of mobile robots such as unmanned surface vehicles (USVs), unmanned ground vehicles (UGVs), or self-driving cars; advanced learning and control development for dynamic robotics; and more.
Satyandra K. Gupta Eva Kanso Mitul Luhar Neda Maghsoodi Quan Nguyen Bingen Yang
Biodynamics Lab Center for Advanced Manufacturing Dynamic Robotics and Control Lab Dynamic Systems Lab Water Channel/Fluid-Structure Interactions Lab
- Diversity, Equity & Inclusion
- Announcements
- Media Coverage
- Events Calendar
- Research Areas
- Lab Directory
- Publications
- Faculty Directory
- Tenured/Tenure-track Faculty
- Teaching/Practice Faculty
- Research Faculty
- Adjunct Faculty
- Joint Faculty
- Emeritus Faculty
- Part-time Instructional Faculty
- Undergraduate Student Resources
- Graduate Student Resources
- Student Activities
- Industry and Career Resources
- Opportunities
- Make a Gift
Research Focus Areas
Robotics is a broad field encompassing many aspects of science and engineering. Students in Robotics Engineering have the opportunity to work with faculty on many topics and to make fundamental contributions to this evolving field. Below are our faculty members' current areas of research.
It Starts with Research—and Leads to Virtually Endless Opportunities
Scientific inquiry can end up in an unlimited number of destinations. But invariably, it all begins at the same starting point: research . Tireless research helped WPI identify the growth and proliferation of robotics in increasing areas of society. This led to the recognition of the burgeoning demand for qualified robotics engineers and, consequently, the need for top-notch education programs.
The result was WPI’s first-in-the-nation BS in Robotics Engineering, followed by MS, PhD, and our BS/MS combined program.
Likewise, ongoing research keeps WPI at the forefront of robotics engineering advances and breakthroughs. Students and faculty members take advantage of the almost endless opportunities to engage in cutting-edge research across multiple disciplines. Their results are rewarding, inspiring, and historic.
Find out about the research focus areas of our faculty members.
Human–Robot Interaction and Interfaces
- Scott Barton
- Zhi (Jane) Li
- Markus Nemitz
- Cagdas Onal
- Carlo Pinciroli
- Jeanine Skorinko
- Haichong (Kai) Zhang
Robot Motion Planning and Control
- Raghvendra Cowlagi
- Zhi ( Jane) Li
Medical Robotics and Assistive Robots
- Loris Fichera
- Greg Fischer
- Haichong (Kai) Zhang
Novel Sensors/Actuators and Robot Design
- Mahdi Agheli
- Siavash Farzan
Robotics and AI
Sensing and perception, human augmentation, robotic manipulation, soft robots, autonomous vehicles.
- Xinming Huang
- Alexander Wyglinski
Cyberphysical Systems
- James Duckworth
- William Michalson
Multi-Robot Systems
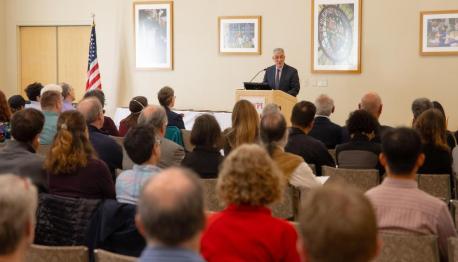
2024 Annual Awards Honor Faculty and Teaching Assistants
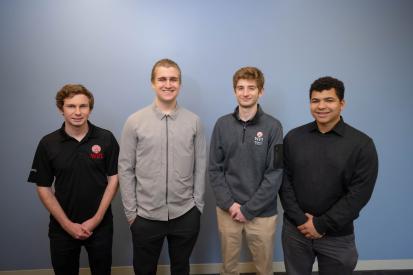
Student Work on Robotic Surgical Instrument Honored at International Conference
An official website of the United States government
Here's how you know
Official websites use .gov A .gov website belongs to an official government organization in the United States.
Secure .gov websites use HTTPS. A lock ( Lock Locked padlock ) or https:// means you've safely connected to the .gov website. Share sensitive information only on official, secure websites.
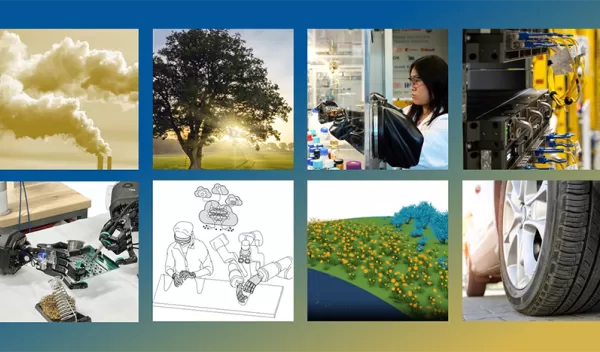
NSF announces 4 new Engineering Research Centers focused on biotechnology, manufacturing, robotics and sustainability
Engineering innovations transform our lives and energize the economy. The U.S. National Science Foundation announces a five-year investment of $104 million, with a potential 10-year investment of up to $208 million, in four new NSF Engineering Research Centers (ERCs) to create technology-powered solutions that benefit the nation for decades to come.
"NSF's Engineering Research Centers ask big questions in order to catalyze solutions with far-reaching impacts," said NSF Director Sethuraman Panchanathan. "NSF Engineering Research Centers are powerhouses of discovery and innovation, bringing America's great engineering minds to bear on our toughest challenges. By collaborating with industry and training the workforce of the future, ERCs create an innovation ecosystem that can accelerate engineering innovations, producing tremendous economic and societal benefits for the nation."
The new centers will develop technologies to tackle the carbon challenge, expand physical capabilities, make heating and cooling more sustainable and enable the U.S. supply and manufacturing of natural rubber.
The 2024 ERCs are:
- NSF ERC for Carbon Utilization Redesign through Biomanufacturing-Empowered Decarbonization (CURB) — Washington University in St. Louis in partnership with the University of Delaware, Prairie View A&M University and Texas A&M University. CURB will create manufacturing systems that convert CO2 to a broad range of products much more efficiently than current state-of-the-art engineered and natural systems.
- NSF ERC for Environmentally Applied Refrigerant Technology Hub (EARTH) — University of Kansas in partnership with Lehigh University, University of Hawaii, University of Maryland, University of Notre Dame and University of South Dakota. EARTH will create a transformative, sustainable refrigerant lifecycle to reduce global warming from refrigerants while increasing the energy efficiency of heating, ventilation and cooling.
- NSF ERC for Human AugmentatioN via Dexterity (HAND) — Northwestern University in partnership with Carnegie Mellon University, Florida A&M University, and Texas A&M University, and with engagement of MIT. HAND will revolutionize the ability of robots to augment human labor by transforming dexterous robot hands into versatile, easy-to-integrate tools.
- NSF ERC for Transformation of American Rubber through Domestic Innovation for Supply Security (TARDISS) — The Ohio State University in partnership with Caltech, North Carolina State University, Texas Tech University and the University of California, Merced. TARDISS will create bridges between engineering, biology, and agriculture to revolutionize and on-shore alternative natural rubber production from U.S. crops.
Since its founding in 1985, NSF's ERC program has funded 83 centers (including the four announced today) that receive support for up to 10 years. The centers build partnerships with educational institutions, government agencies and industry stakeholders to support innovation and inclusion in established and emerging engineering research.
Visit NSF's website and read about NSF Engineering Research Centers .
Research areas
- Open access
- Published: 10 February 2023
Trends and research foci of robotics-based STEM education: a systematic review from diverse angles based on the technology-based learning model
- Darmawansah Darmawansah ORCID: orcid.org/0000-0002-3464-4598 1 ,
- Gwo-Jen Hwang ORCID: orcid.org/0000-0001-5155-276X 1 , 3 ,
- Mei-Rong Alice Chen ORCID: orcid.org/0000-0003-2722-0401 2 &
- Jia-Cing Liang ORCID: orcid.org/0000-0002-1134-527X 1
International Journal of STEM Education volume 10 , Article number: 12 ( 2023 ) Cite this article
22k Accesses
29 Citations
5 Altmetric
Metrics details
Fostering students’ competence in applying interdisciplinary knowledge to solve problems has been recognized as an important and challenging issue globally. This is why STEM (Science, Technology, Engineering, Mathematics) education has been emphasized at all levels in schools. Meanwhile, the use of robotics has played an important role in STEM learning design. The purpose of this study was to fill a gap in the current review of research on Robotics-based STEM (R-STEM) education by systematically reviewing existing research in this area. This systematic review examined the role of robotics and research trends in STEM education. A total of 39 articles published between 2012 and 2021 were analyzed. The review indicated that R-STEM education studies were mostly conducted in the United States and mainly in K-12 schools. Learner and teacher perceptions were the most popular research focus in these studies which applied robots. LEGO was the most used tool to accomplish the learning objectives. In terms of application, Technology (programming) was the predominant robotics-based STEM discipline in the R-STEM studies. Moreover, project-based learning (PBL) was the most frequently employed learning strategy in robotics-related STEM research. In addition, STEM learning and transferable skills were the most popular educational goals when applying robotics. Based on the findings, several implications and recommendations to researchers and practitioners are proposed.
Introduction
Over the past few years, implementation of STEM (Science, Technology, Engineering, and Mathematics) education has received a positive response from researchers and practitioners alike. According to Chesloff ( 2013 ), the winning point of STEM education is its learning process, which validates that students can use their creativity, collaborative skills, and critical thinking skills. Consequently, STEM education promotes a bridge between learning in authentic real-life scenarios (Erdoğan et al., 2016 ; Kelley & Knowles, 2016 ). This is the greatest challenge facing STEM education. The learning experience and real-life situation might be intangible in some areas due to pre- and in-conditioning such as unfamiliarity with STEM content (Moomaw, 2012 ), unstructured learning activities (Sarama & Clements, 2009), and inadequate preparation of STEM curricula (Conde et al., 2021 ).
In response to these issues, the adoption of robotics in STEM education has been encouraged as part of an innovative and methodological approach to learning (Bargagna et al., 2019 ; Ferreira et al., 2018 ; Kennedy et al., 2015 ; Köse et al., 2015 ). Similarly, recent studies have reported that the use of robots in school settings has an impact on student curiosity (Adams et al., 2011 ), arts and craftwork (Sullivan & Bers, 2016 ), and logic (Bers, 2008 ). When robots and educational robotics are considered a core part of STEM education, it offers the possibility to promote STEM disciplines such as engineering concepts or even interdisciplinary practices (Okita, 2014 ). Anwar et. al. ( 2019 ) argued that integration between robots and STEM learning is important to support STEM learners who do not immediately show interest in STEM disciplines. Learner interest can elicit the development of various skills such as computational thinking, creativity and motivation, collaboration and cooperation, problem-solving, and other higher-order thinking skills (Evripidou et al., 2020 ). To some extent, artificial intelligence (AI) has driven the use of robotics and tools, such as their application to designing instructional activities (Hwang et al., 2020 ). The potential for research on robotics in STEM education can be traced by showing the rapid increase in the number of studies over the past few years. The emphasis is on critically reviewing existing research to determine what prior research already tells us about R-STEM education, what it means, and where it can influence future research. Thus, this study aimed to fill the gap by conducting a systematic review to grasp the potential of R-STEM education.
In terms of providing the core concepts of roles and research trends of R-STEM education, this study explored beyond the scope of previous reviews by conducting content analysis to see the whole picture. To address the following questions, this study analyzed published research in the Web of Science database regarding the technology-based learning model (Lin & Hwang, 2019 ):
In terms of research characteristic and features, what were the location, sample size, duration of intervention, research methods, and research foci of the R-STEM education research?
In terms of interaction between participants and robots, what were the participants, roles of the robot, and types of robot in the R-STEM education research?
In terms of application, what were the dominant STEM disciplines, contribution to STEM disciplines, integration of robots and STEM, pedagogical interventions, and educational objectives of the R-STEM research?
- Literature review
Previous studies have investigated the role of robotics in R-STEM education from several research foci such as the specific robot users (Atman Uslu et al., 2022 ; Benitti, 2012 ; Jung & Won, 2018 ; Spolaôr & Benitti, 2017 ; van den Berghe et al., 2019 ), the potential value of R-STEM education (Çetin & Demircan, 2020 ; Conde et al., 2021 ; Zhang et al., 2021 ), and the types of robots used in learning practices (Belpaeme et al., 2018 ; Çetin & Demircan, 2020 ; Tselegkaridis & Sapounidis, 2021 ). While their findings provided a dynamic perspective on robotics, they failed to contribute to the core concept of promoting R-STEM education. Those previous reviews did not summarize the exemplary practice of employing robots in STEM education. For instance, Spolaôr and Benitti ( 2017 ) concluded that robots could be an auxiliary tool for learning but did not convey whether the purpose of using robots is essential to enhance learning outcomes. At the same time, it is important to address the use and purpose of robotics in STEM learning, the connections between theoretical pedagogy and STEM practice, and the reasons for the lack of quantitative research in the literature to measure student learning outcomes.
First, Benitti ( 2012 ) reviewed research published between 2000 and 2009. This review study aimed to determine the educational potential of using robots in schools and found that it is feasible to use most robots to support the pedagogical process of learning knowledge and skills related to science and mathematics. Five years later, Spolaôr and Benitti ( 2017 ) investigated the use of robots in higher education by employing the adopted-learning theories that were not covered in their previous review in 2012. The study’s content analysis approach synthesized 15 papers from 2002 to 2015 that used robots to support instruction based on fundamental learning theory. The main finding was that project-based learning (PBL) and experiential learning, or so-called hands-on learning, were considered to be the most used theories. Both theories were found to increase learners’ motivation and foster their skills (Behrens et al., 2010 ; Jou et al., 2010 ). However, the vast majority of discussions of the selected reviews emphasized positive outcomes while overlooking negative or mixed outcomes. Along the same lines, Jung and Won ( 2018 ) also reviewed theoretical approaches to Robotics education in 47 studies from 2006 to 2017. Their focused review of studies suggested that the employment of robots in learning should be shifted from technology to pedagogy. This review paper argued to determine student engagement in robotics education, despite disagreements among pedagogical traits. Although Jung and Won ( 2018 ) provided information of teaching approaches applied in robotics education, they did not offer critical discussion on how those approaches were formed between robots and the teaching disciplines.
On the other hand, Conde et. al. ( 2021 ) identified PBL as the most common learning approach in their study by reviewing 54 papers from 2006 to 2019. Furthermore, the studies by Çetin and Demircan ( 2020 ) and Tselegkaridis and Sapounidis ( 2021 ) focused on the types of robots used in STEM education and reviewed 23 and 17 papers, respectively. Again, these studies touted learning engagement as a positive outcome, and disregarded the different perspectives of robot use in educational settings on students’ academic performance and cognition. More recently, a meta-analysis by Zhang et. al. ( 2021 ) focused on the effects of robotics on students’ computational thinking and their attitudes toward STEM learning. In addition, a systematic review by Atman Uslu et. al. ( 2022 ) examined the use of educational robotics and robots in learning.
So far, the review study conducted by Atman Uslu et. al. ( 2022 ) could be the only study that has attempted to clarify some of the criticisms of using educational robots by reviewing the studies published from 2006 to 2019 in terms of their research issues (e.g., interventions, interactions, and perceptions), theoretical models, and the roles of robots in educational settings. However, they failed to take into account several important features of robots in education research, such as thematic subjects and educational objectives, for instance, whether robot-based learning could enhance students’ competence of constructing new knowledge, or whether robots could bring either a motivational facet or creativity to pedagogy to foster students’ learning outcomes. These are essential in investigating the trends of technology-based learning research as well as the role of technology in education as a review study is aimed to offer a comprehensive discussion which derived from various angles and dimensions. Moreover, the role of robots in STEM education was generally ignored in the previous review studies. Hence, there is still a need for a comprehensive understanding of the role of robotics in STEM education and research trends (e.g., research issues, interaction issues, and application issues) so as to provide researchers and practitioners with valuable references. That is, our study can remedy the shortcomings of previous reviews (Additional file 1 ).
The above comments demonstrate how previous scholars have understood what they call “the effectiveness of robotics in STEM education” in terms of innovative educational tools. In other words, despite their useful findings and ongoing recommendations, there has not been a thorough investigation of how robots are widely used from all angles. Furthermore, the results of existing review studies have been less than comprehensive in terms of the potential role of robotics in R-STEM education after taking into account various potential dimensions based on the technology-based model that we propose in this study.
The studies in this review were selected from the literature on the Web of Science, our sole database due to its rigorous journal research and qualified studies (e.g., Huang et al., 2022 ), discussing the adoption of R-STEM education, and the data collection procedures for this study followed the Preferred Reporting Items for Systematic Reviews and Meta-Analyses (PRISMA) guidelines (Moher et al., 2009 ) as referred to by prior studies (e.g., Chen et al., 2021a , 2021b ; García-Martínez et al., 2020 ). Considering publication quality, previous studies (Fu & Hwang, 2018 ; Martín-Páez et al., 2019 ) suggested using Boolean expressions to search Web of Science databases. The search terms for “robot” are “robot” or “robotics” or “robotics” or “Lego” (Spolaôr & Benitti, 2017 ). According to Martín-Páez et. al. ( 2019 ), expressions for STEM education include “STEM” or “STEM education” or “STEM literacy” or “STEM learning” or “STEM teaching” or “STEM competencies”. These search terms were entered into the WOS database to search only for SSCI papers due to its wide recognition as being high-quality publications in the field of educational technology. As a result, 165 papers were found in the database. The search was then restricted to 2012–2021 as suggested by Hwang and Tsai ( 2011 ). In addition, the number of papers was reduced to 131 by selecting only publications of the “article” type and those written in “English”. Subsequently, we selected the category “education and educational research” which reduced the number to 60 papers. During the coding analysis, the two coders screened out 21 papers unrelated to R-STEM education. The coding result had a Kappa coefficient of 0.8 for both coders (Cohen, 1960 ). After the screening stage, a final total of 39 articles were included in this study, as shown in Fig. 1 . Also, the selected papers are marked with an asterisk in the reference list and are listed in Appendixes 1 and 2 .
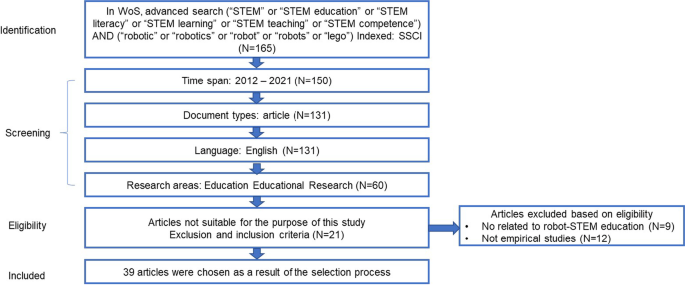
PRISMA procedure for the selection process
Theoretical model, data coding, and analysis
This study comprised content analysis using a coding scheme to provide insights into different aspects of the studies in question (Chen et al., 2021a , 2021b ; Martín-Páez et al., 2019 ). The coding scheme adopted the conceptual framework proposed by Lin and Hwang ( 2019 ), comprising “STEM environments”, “learners”, and “robots”, as shown in Fig. 2 . Three issues were identified:
In terms of research issues, five dimensions were included: “location”, “sample size”, “duration of intervention”, (Zhong & Xia, 2020 ) “research methods”, (Johnson & Christensen, 2000 ) and “research foci”. (Hynes et al., 2017 ; Spolaôr & Benitti, 2017 ).
In terms of interaction issues, three dimensions were included: “participants”, (Hwang & Tsai, 2011 ), “roles of the robot”, and “types of robot” (Taylor, 1980 ).
In terms of application, five dimensions were included, namely “dominant STEM disciplines”, “integration of robot and STEM” (Martín‐Páez et al., 2019 ), “contribution to STEM disciplines”, “pedagogical intervention”, (Spolaôr & Benitti, 2017 ) and “educational objectives” (Anwar et al., 2019 ). Table 1 shows the coding items in each dimension of the investigated issues.
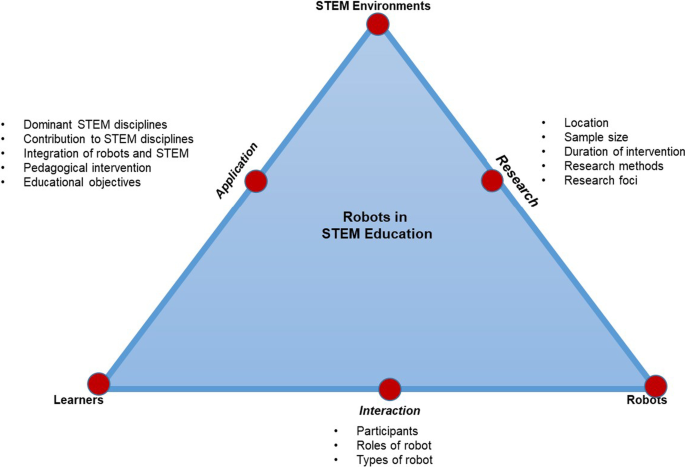
Model of R-STEM education theme framework
Figure 3 shows the distribution of the publications selected from 2012 to 2021. The first two publications were found in 2012. From 2014 to 2017, the number of publications steadily increased, with two, three, four, and four publications, respectively. Moreover, R-STEM education has been increasingly discussed within the last 3 years (2018–2020) with six, three, and ten publications, respectively. The global pandemic in the early 2020s could have affected the number of papers published, with only five papers in 2021. This could be due to the fact that most robot-STEM education research is conducted in physical classroom settings.
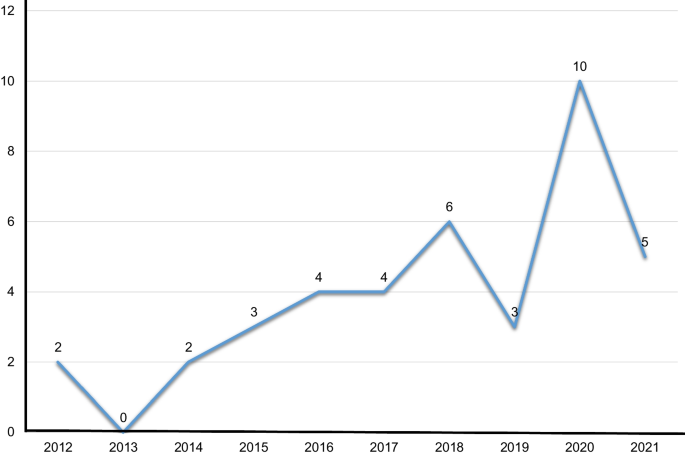
Number of publications on R-STEM education from 2012 to 2021
Table 2 displays the journals in which the selected papers were published, the number of papers published in each journal, and the journal’s impact factor. It can be concluded that most of the papers on R-STEM education research were published in the Journal of Science Education and Technology , and the International Journal of Technology and Design Education , with six papers, respectively.
Research issues
The geographic distribution of the reviewed studies indicated that more than half of the studies were conducted in the United States (53.8%), while Turkey and China were the location of five and three studies, respectively. Taiwan, Canada, and Italy were indicated to have two studies each. One study each was conducted in Australia, Mexico, and the Netherlands. Figure 4 shows the distribution of the countries where the R-STEM education was conducted.
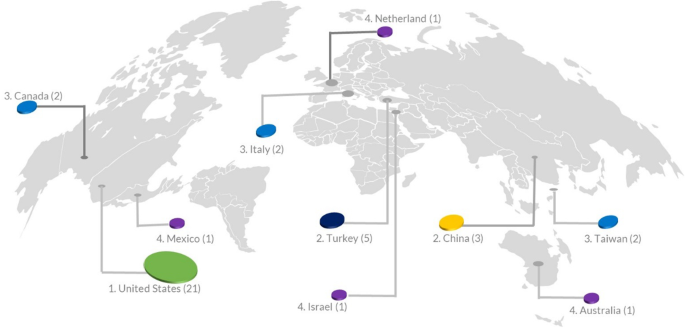
Locations where the studies were conducted ( N = 39)
Sample size
Regarding sample size, there were four most common sample sizes for the selected period (2012–2021): greater than 80 people (28.21% or 11 out of 39 studies), between 41 and 60 (25.64% or 10 out of 39 studies), 1 to 20 people (23.08% or 9 out of 39), and between 21 and 40 (20.51% or 8 out of 39 studies). The size of 61 to 80 people (2.56% or 1 out of 39 studies) was the least popular sample size (see Fig. 5 ).
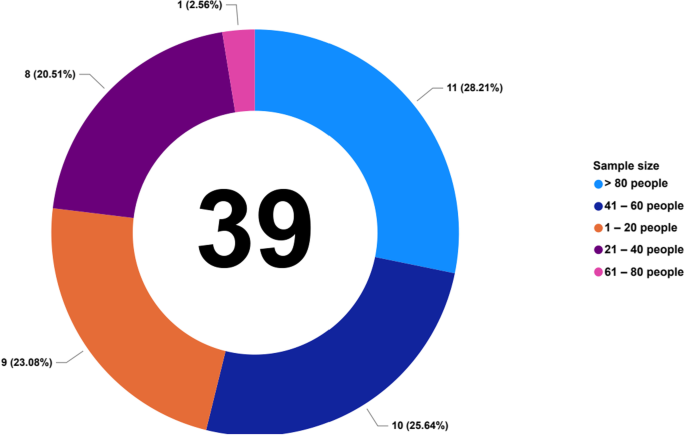
Sample size across the studies ( N = 39)
Duration of intervention
Regarding the duration of the study (see Fig. 6 ), experiments were mostly conducted for less than or equal to 4 weeks (35.9% or 14 out of 39 studies). This was followed by less than or equal to 8 weeks (25.64% or 10 out of 39 studies), less than or equal to 6 months (20.51% or 8 out 39 studies), less than or equal to 12 months (10.26% or 4 out of 39 studies), while less than or equal to 1 day (7.69% or 3 out of 39 studies) was the least chosen duration.
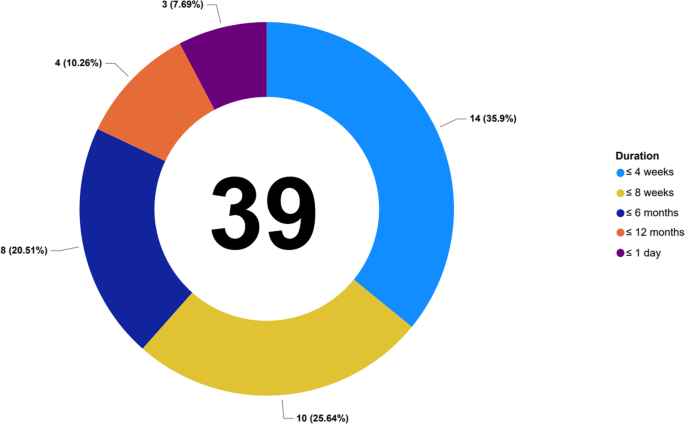
Duration of interventions across the studies ( N = 39)
Research methods
Figure 7 demonstrates the trends in research methods from 2012 to 2021. The use of questionnaires or surveys (35.9% or 14 out of 39 studies) and mixed methods research (35.9% or 14 out of 39 studies) outnumbered other methods such as experimental design (25.64% or 10 out of 39 studies) and system development (2.56% or 1 out of 39 studies).
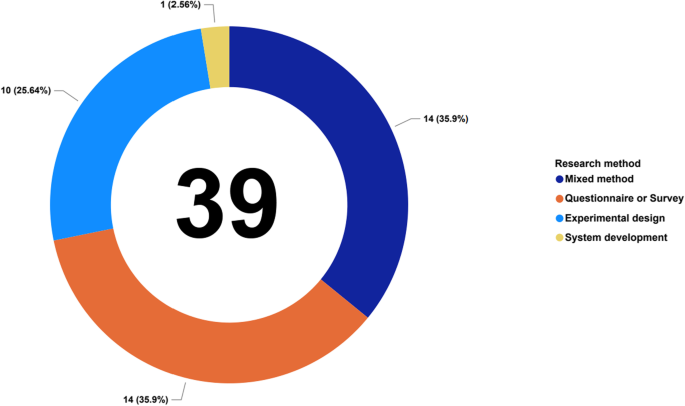
Frequency of each research method used in 2012–2021
Research foci
In these studies, research foci were divided into four aspects: cognition, affective, operational skill, and learning behavior. If the study involved more than one research focus, each issue was coded under each research focus.
In terms of cognitive skills, students’ learning performance was the most frequently measured (15 out of 39 studies). Six studies found that R-STEM education brought a positive result to learning performance. Two studies did not find any significant difference, while five studies showed mixed results or found that it depends. For example, Chang and Chen ( 2020 ) revealed that robots in STEM learning improved students’ cognition such as designing, electronic components, and computer programming.
In terms of affective skills, just over half of the reviewed studies (23 out of 39, 58.97%) addressed the students’ or teachers’ perceptions of employing robots in STEM education, of which 14 studies showed positive perceptions. In contrast, nine studies found mixed results. For instance, Casey et. al. ( 2018 ) determined students’ mixed perceptions of the use of robots in learning coding and programming.
Five studies were identified regarding operational skills by investigating students’ psychomotor aspects such as construction and mechanical elements (Pérez & López, 2019 ; Sullivan & Bers, 2016 ) and building and modeling robots (McDonald & Howell, 2012 ). Three studies found positive results, while two reported mixed results.
In terms of learning behavior, five out of 39 studies measured students’ learning behavior, such as students’ engagement with robots (Ma et al., 2020 ), students’ social behavior while interacting with robots (Konijn & Hoorn, 2020 ), and learner–parent interactions with interactive robots (Phamduy et al., 2017 ). Three studies showed positive results, while two found mixed results or found that it depends (see Table 3 ).
Interaction issues
Participants.
Regarding the educational level of the participants, elementary school students (33.33% or 13 studies) were the most preferred study participants, followed by high school students (15.38% or 6 studies). The data were similar for preschool, junior high school, in-service teachers, and non-designated personnel (10.26% or 4 studies). College students, including pre-service teachers, were the least preferred study participants. Interestingly, some studies involved study participants from more than one educational level. For example, Ucgul and Cagiltay ( 2014 ) conducted experiments with elementary and middle school students, while Chapman et. al. ( 2020 ) investigated the effectiveness of robots with elementary, middle, and high school students. One study exclusively investigated gifted and talented students without reporting their levels of education (Sen et al., 2021 ). Figure 8 shows the frequency of study participants between 2012 and 2021.
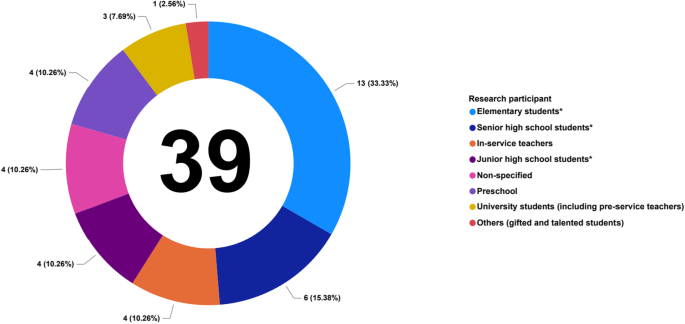
Frequency of research participants in the selected period
The roles of robot
For the function of robots in STEM education, as shown in Fig. 9 , more than half of the selected articles used robots as tools (31 out of 39 studies, 79.49%) for which the robots were designed to foster students’ programming ability. For instance, Barker et. al. ( 2014 ) investigated students’ building and programming of robots in hands-on STEM activities. Seven out of 39 studies used robots as tutees (17.95%), with the aim of students and teachers learning to program. For example, Phamduy et. al. ( 2017 ) investigated a robotic fish exhibit to analyze visitors’ experience of controlling and interacting with the robot. The least frequent role was tutor (2.56%), with only one study which programmed the robot to act as tutor or teacher for students (see Fig. 9 ).
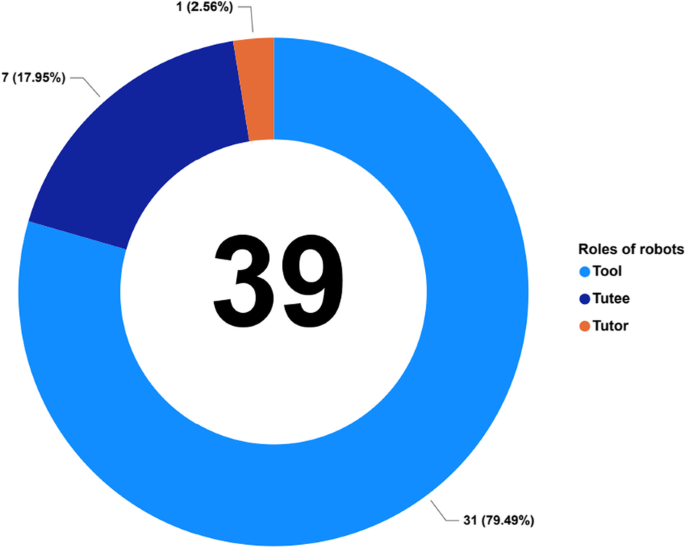
Frequency of roles of robots
Types of robot
Furthermore, in terms of the types of robots used in STEM education, the LEGO MINDSTORMS robot was the most used (35.89% or 14 out of 39 studies), while Arduino was the second most used (12.82% or 5 out of 39 studies), and iRobot Create (5.12% or 2 out of 39 studies), and NAO (5.12% or 2 out of 39 studies) ranked third equal, as shown in Fig. 10 . LEGO was used to solve STEM problem-solving tasks such as building bridges (Convertini, 2021 ), robots (Chiang et al., 2020 ), and challenge-specific game boards (Leonard et al., 2018 ). Furthermore, four out of 36 studies did not specify the robots used in their studies.
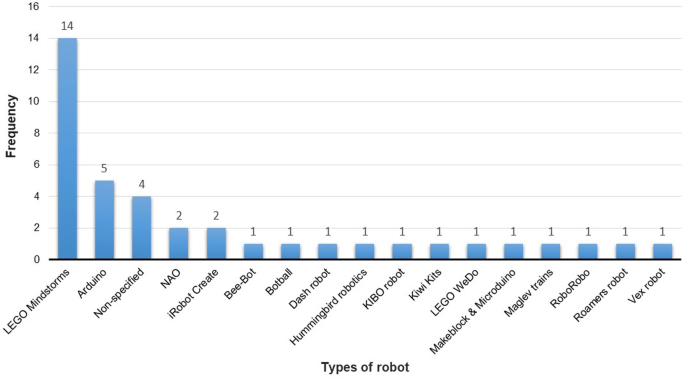
Frequency of types of robots used
Application issues
The dominant disciplines and the contribution to stem disciplines.
As shown in Table 4 , the most dominant discipline in R-STEM education research published from 2012 to 2021 was technology. Engineering, mathematics, and science were the least dominant disciplines. Programming was the most common subject for robotics contribution to the STEM disciplines (25 out of 36 studies, 64.1%), followed by engineering (12.82%), and mathematical method (12.82%). We found that interdisciplinary was discussed in the selected period, but in relatively small numbers. However, this finding is relevant to expose the use of robotics in STEM disciplines as a whole. For example, Barker et. al. ( 2014 ) studied how robotics instructional modules in geospatial and programming domains could be impacted by fidelity adherence and exposure to the modules. The dominance of STEM subjects based on robotics makes it necessary to study the way robotics and STEM are integrated into the learning process. Therefore, the forms of STEM integration are discussed in the following sub-section to report how teaching and learning of these disciplines can have learning goals in an integrated STEM environment.
Integration of robots and STEM
There are three general forms of STEM integration (see Fig. 11 ). Of these studies, robot-STEM content integration was commonly used (22 studies, 56.41%), in which robot activities had multiple STEM disciplinary learning objectives. For example, Chang and Chen ( 2020 ) employed Arduino in a robotics sailboat curriculum. This curriculum was a cross-disciplinary integration, the objectives of which were understanding sailboats and sensors (Science), the direction of motors and mechanical structures (Engineering), and control programming (Technology). The second most common form was supporting robot-STEM content integration (12 out of 39 studies, 30.76%). For instance, KIBO robots were used in the robotics activities where the mechanical elements content area was meaningfully covered in support of the main programming learning objectives (Sullivan & Bers, 2019 ). The least common form was robot-STEM context integration (5 out of 39 studies, 12.82%) which was implemented through the robot to situate the disciplinary content goals in another discipline’s practices. For example, Christensen et. al. ( 2015 ) analyzed the impact of an after-school program that offered robots as part of students’ challenges in a STEM competition environment (geoscience and programming).
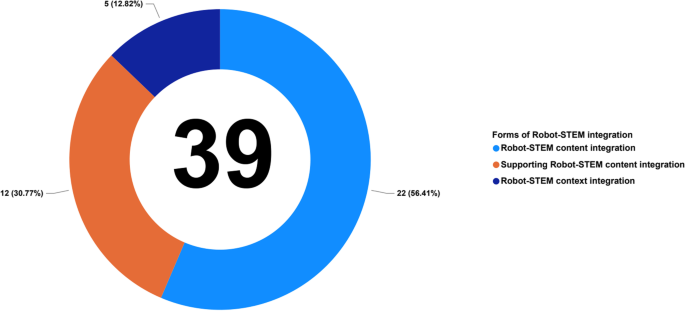
The forms of robot-STEM integration
Pedagogical interventions
In terms of instructional interventions, as shown in Fig. 12 , project-based learning (PBL) was the preferred instructional theory for using robots in R-STEM education (38.46% or 15 out 39 studies), with the aim of motivating students or robot users in the STEM learning activities. For example, Pérez and López ( 2019 ) argued that using low-cost robots in the teaching process increased students’ motivation and interest in STEM areas. Problem-based learning was the second most used intervention in this dimension (17.95% or 7 out of 39 studies). It aimed to improve students’ motivation by giving them an early insight into practical Engineering and Technology. For example, Gomoll et. al. ( 2017 ) employed robots to connect students from two different areas to work collaboratively. Their study showed the importance of robotic engagement in preliminary learning activities. Edutainment (12.82% or 5 out of 39 studies) was the third most used intervention. This intervention was used to bring together students and robots and to promote learning by doing. Christensen et. al. ( 2015 ) and Phamduy et. al. ( 2017 ) were the sample studies that found the benefits of hands-on and active learning engagement; for example, robotics competitions and robotics exhibitions could help retain a positive interest in STEM activities.
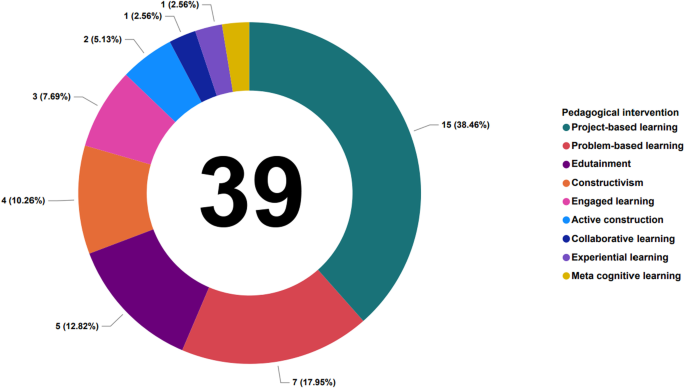
The pedagogical interventions in R-STEM education
Educational objectives
As far as the educational objectives of robots are concerned (see Fig. 13 ), the majority of robots are used for learning and transfer skills (58.97% or 23 out of 39 studies) to enhance students’ construction of new knowledge. It emphasized the process of learning through inquiry, exploration, and making cognitive associations with prior knowledge. Chang and Chen’s ( 2020 ) is a sample study on how learning objectives promote students’ ability to transfer science and engineering knowledge learned through science experiments to design a robotics sailboat that could navigate automatically as a novel setting. Moreover, it also explicitly aimed to examine the hands-on learning experience with robots. For example, McDonald and Howell ( 2012 ) described how robots engaged with early year students to better understand the concepts of literacy and numeracy.
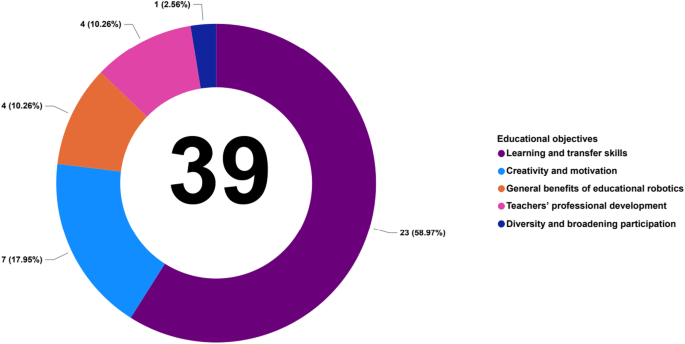
Educational objectives of R-STEM education
Creativity and motivation were found to be educational objectives in R-STEM education for seven out of 39 studies (17.94%). It was considered from either the motivational facet of social trend or creativity in pedagogy to improve students’ interest in STEM disciplines. For instance, these studies were driven by the idea that employing robots could develop students’ scientific creativity (Guven et al., 2020 ), confidence and presentation ability (Chiang et al., 2020 ), passion for college and STEM fields (Meyers et al., 2012 ), and career choice (Ayar, 2015 ).
The general benefits of educational robots and the professional development of teachers were equally found in four studies each. The first objective, the general benefits of educational robotics, was to address those studies that found a broad benefit of using robots in STEM education without highlighting the particular focus. The sample studies suggested that robotics in STEM could promote active learning and improve students’ learning experience through social interaction (Hennessy Elliott, 2020 ) and collaborative science projects (Li et al., 2016 ). The latter, teachers’ professional development, was addressed by four studies (10.25%) to utilize robots to enhance teachers’ efficacy. Studies in this category discussed how teachers could examine and identify distinctive instructional approaches with robotics work (Bernstein et al., 2022 ), design meaningful learning instruction (Ryan et al., 2017 ) and lesson materials (Kim et al., 2015 ), and develop more robust cultural responsive self-efficacy (Leonard et al., 2018 ).
This review study was conducted using content analysis from the WOS collection of research on robotics in STEM education from 2012 to 2021. The findings are discussed under the headings of each research question.
RQ 1: In terms of research, what were the location, sample size, duration of intervention, research methods, and research foci of the R-STEM education research?
About half of the studies were conducted in North America (the USA and Canada), while limited studies were found from other continents (Europe and the Asia Pacific). This trend was identified in the previous study on robotics for STEM activities (Conde et al., 2021 ). Among 39 studies, 28 (71.79%) had fewer than 80 participants, while 11 (28.21%) had more than 80 participants. The intervention’s duration across the studies was almost equally divided between less than or equal to a month (17 out of 39 studies, 43.59%) and more than a month (22 out of 39 studies, 56.41%). The rationale behind the most popular durations is that these studies were conducted in classroom experiments and as conditional learning. For example, Kim et. al. ( 2018 ) conducted their experiments in a course offered at a university where it took 3 weeks based on a robotics module.
A total of four different research methodologies were adopted in the studies, the two most popular being mixed methods (35.89%) and questionnaires or surveys (35.89%). Although mixed methods can be daunting and time-consuming to conduct (Kucuk et al., 2013 ), the analysis found that it was one of the most used methods in the published articles, regardless of year. Chang and Chen ( 2022 ) embedded a mixed-methods design in their study to qualitatively answer their second research question. The possible reason for this is that other researchers prefer to use mixed methods as their research design. Their main research question was answered quantitatively, while the second and remaining research questions were reported through qualitative analysis (Casey et al., 2018 ; Chapman et al., 2020 ; Ma et al., 2020 ; Newton et al., 2020 ; Sullivan & Bers, 2019 ). Thus, it was concluded that mixed methods could lead to the best understanding and integration of research questions (Creswell & Clark, 2013 ; Creswell et al., 2003 ).
In contrast, system development was the least used compared to other study designs, as most studies used existing robotic systems. It should be acknowledged that the most common outcome we found was to enable students to understand these concepts as they relate to STEM subjects. Despite the focus on system development, the help of robotics was identified as increasing the success of STEM learning (Benitti, 2012 ). Because limited studies focused on system development as their primary purpose (1 out of 39 studies, 2.56%), needs analyses may ask whether the mechanisms, types, and challenges of robotics are appropriate for learners. Future research will need further design and development of personalized robots to fill this part of the research gap.
About half of the studies (23 studies, 58.97%) were focused on investigating the effectiveness of robots in STEM learning, primarily by collecting students’ and teachers’ opinions. This result is more similar to Belpaeme et al. ( 2018 ) finding that users’ perceptions were common measures in studies on robotics learning. However, identifying perceptions of R-STEM education may not help us understand exactly how robots’ specific features afford STEM learning. Therefore, it is argued that researchers should move beyond such simple collective perceptions in future research. Instead, further studies may compare different robots and their features. For instance, whether robots with multiple sensors, a sensor, or without a sensor could affect students’ cognitive, metacognitive, emotional, and motivational in STEM areas (e.g., Castro et al., 2018 ). Also, there could be instructional strategies embedded in R-STEM education that can lead students to do high-order thinking, such as problem-solving with a decision (Özüorçun & Bicen, 2017 ), self-regulated and self-engagement learning (e.g., Li et al., 2016 ). Researchers may also compare the robotics-based approach with other technology-based approaches (e.g., Han et al., 2015 ; Hsiao et al., 2015 ) in supporting STEM learning.
RQ 2: In terms of interaction, what were the participants, roles of the robots, and types of robots of the R-STEM education research?
The majority of reviewed studies on R-STEM education were conducted with K-12 students (27 studies, 69.23%), including preschool, elementary school, junior, and high school students. There were limited studies that involved higher education students and teachers. This finding is similar to the previous review study (Atman Uslu et al., 2022 ), which found a wide gap among research participants between K-12 students and higher education students, including teachers. Although it is unclear why there were limited studies conducted involving teachers and higher education students, which include pre-service teachers, we are aware of the critical task of designing meaningful R-STEM learning experiences which is likely to require professional development. In this case, both pre- and in-service teachers could examine specific objectives, identify topics, test the application, and design potential instruction to align well with robots in STEM learning (Bernstein et al., 2022 ). Concurrently, these pedagogical content skills in R-STEM disciplines might not be taught in the traditional pre-service teacher education and particular teachers’ development program (Huang et al., 2022 ). Thus, it is recommended that future studies could be conducted to understand whether robots can improve STEM education for higher education students and teachers professionally.
Regarding the role of robots, most were used as learning tools (31 studies, 79.48%). These robots are designed to have the functional ability to command or program some analysis and processing (Taylor, 1980 ). For example, Leonard et. al. ( 2018 ) described how pre-service teachers are trained in robotics activities to facilitate students’ learning of computational thinking. Therefore, robots primarily provide opportunities for learners to construct knowledge and skills. Only one study (2.56%), however, was found to program robots to act as tutors or teachers for students. Designing a robot-assisted system has become common in other fields such as language learning (e.g., Hong et al., 2016 ; Iio et al., 2019 ) and special education (e.g., Özdemir & Karaman, 2017 ) where the robots instruct the learning activities for students. In contrast, R-STEM education has not looked at the robot as a tutor, but has instead focused on learning how to build robots (Konijn & Hoorn, 2020 ). It is argued that robots with features as human tutors, such as providing personalized guidance and feedback, could assist during problem-solving activities (Fournier-Viger et al., 2013 ). Thus, it is worth exploring in what teaching roles the robot will work best as a tutor in STEM education.
When it comes to types of robots, the review found that LEGO dominated robots’ employment in STEM education (15 studies, 38.46%), while the other types were limited in their use. It is considered that LEGO tasks are more often associated with STEM because learners can be more involved in the engineering or technical tasks. Most researchers prefer to use LEGO in their studies (Convertini, 2021 ). Another interesting finding is about the cost of the robots. Although robots are generally inexpensive, some products are particularly low-cost and are commonly available in some regions (Conde et al., 2021 ). Most preferred robots are still considered exclusive learning tools in developing countries and regions. In this case, only one study offered a low-cost robot (Pérez & López, 2019 ). This might be a reason why the selected studies were primarily conducted in the countries and continents where the use of advanced technologies, such as robots, is growing rapidly (see Fig. 4 ). Based on this finding, there is a need for more research on the use of low-cost robots in R-STEM instruction in the least developed areas or regions of the world. For example, Nel et. al. ( 2017 ) designed a STEM program to build and design a robot which exclusively enabling students from low-income household to participate in the R-STEM activities.
RQ 3: In terms of application, what were the dominant STEM disciplines, contribution to STEM disciplines, integration of robots and STEM, pedagogical interventions, and educational objectives of the R-STEM research?
While Technology and Engineering are the dominant disciplines, this review found several studies that directed their research to interdisciplinary issues. The essence of STEM lies in interdisciplinary issues that integrate one discipline into another to create authentic learning (Hansen, 2014 ). This means that some researchers are keen to develop students’ integrated knowledge of Science, Technology, Engineering, and Mathematics (Chang & Chen, 2022 ; Luo et al., 2019 ). However, Science and Mathematics were given less weight in STEM learning activities compared to Technology and Engineering. This issue has been frequently reported as a barrier to implementing R-STEM in the interdisciplinary subject. Some reasons include difficulties in pedagogy and classroom roles, lack of curriculum integration, and a limited opportunity to embody one learning subject into others (Margot & Kettler, 2019 ). Therefore, further research is encouraged to treat these disciplines equally, so is the way of STEM learning integration.
The subject-matter results revealed that “programming” was the most common research focus in R-STEM research (25 studies). Researchers considered programming because this particular topic was frequently emphasized in their studies (Chang & Chen, 2020 , 2022 ; Newton et al., 2020 ). Similarly, programming concepts were taught through support robots for kindergarteners (Sullivan & Bers, 2019 ), girls attending summer camps (Chapman et al., 2020 ), and young learners with disabilities (Lamptey et al., 2021 ). Because programming simultaneously accompanies students’ STEM learning, we believe future research can incorporate a more dynamic and comprehensive learning focus. Robotics-based STEM education research is expected to encounter many interdisciplinary learning issues.
Researchers in the reviewed studies agreed that the robot could be integrated with STEM learning with various integration forms. Bryan et. al. ( 2015 ) argued that robots were designed to develop multiple learning goals from STEM knowledge, beginning with an initial learning context. It is parallel with our finding that robot-STEM content integration was the most common integration form (22 studies, 56.41%). In this form, studies mainly defined their primary learning goals with one or more anchor STEM disciplines (e.g., Castro et al., 2018 ; Chang & Chen, 2020 ; Luo et al., 2019 ). The learning goals provided coherence between instructional activities and assessments that explicitly focused on the connection among STEM disciplines. As a result, students can develop a deep and transferable understanding of interdisciplinary phenomena and problems through emphasizing the content across disciplines (Bryan et al., 2015 ). However, the findings on learning instruction and evaluation in this integration are inconclusive. A better understanding of the embodiment of learning contexts is needed, for instance, whether instructions are inclusive, socially relevant, and authentic in the situated context. Thus, future research is needed to identify the quality of instruction and evaluation and the specific characteristics of robot-STEM integration. This may place better provision of opportunities for understanding the form of pedagogical content knowledge to enhance practitioners’ self-efficacy and pedagogical beliefs (Chen et al., 2021a , 2021b ).
Project-based learning (PBL) was the most used instructional intervention with robots in R-STEM education (15 studies, 38.46%). Blumenfeld et al. ( 1991 ) credited PBL with the main purpose of engaging students in investigating learning models. In the case of robotics, students can create robotic artifacts (Spolaôr & Benitti, 2017 ). McDonald and Howell ( 2012 ) used robotics to develop technological skills in lower grades. Leonard et. al. ( 2016 ) used robots to engage and develop students’ computational thinking strategies in another example. In the aforementioned study, robots were used to support learning content in informal education, and both teachers and students designed robotics experiences aligned with the curriculum (Bernstein et al., 2022 ). As previously mentioned, this study is an example of how robots can cover STEM content from the learning domain to support educational goals.
The educational goal of R-STEM education was the last finding of our study. Most of the reviewed studies focused on learning and transferable skills as their goals (23 studies, 58.97%). They targeted learning because the authors investigated the effectiveness of R-STEM learning activities (Castro et al., 2018 ; Convertini, 2021 ; Konijn & Hoorn, 2020 ; Ma et al., 2020 ) and conceptual knowledge of STEM disciplines (Barak & Assal, 2018 ; Gomoll et al., 2017 ; Jaipal-Jamani & Angeli 2017 ). They targeted transferable skills because they require learners to develop individual competencies in STEM skills (Kim et al., 2018 ; McDonald & Howell, 2012 ; Sullivan & Bers, 2016 ) and to master STEM in actual competition-related skills (Chiang et al., 2020 ; Hennessy Elliott, 2020 ).
Conclusions and implications
The majority of the articles examined in this study referred to theoretical frameworks or certain applications of pedagogical theories. This finding contradicts Atman Uslu et. al. ( 2022 ), who concluded that most of the studies in this domain did not refer to pedagogical approaches. Although we claim the employment pedagogical frameworks in the examined articles exist, those articles primarily did not consider a strict instructional design when employing robots in STEM learning. Consequently, the discussions in the studies did not include how the learning–teaching process affords students’ positive perceptions. Therefore, both practitioners and researchers should consider designing learning instruction using robots in STEM education. To put an example, the practitioners may regard students’ zone of proximal development (ZPD) when employing robot in STEM tasks. Giving an appropriate scaffolding and learning contents are necessary for them to enhance their operational skills, application knowledge and emotional development. Although the integration between robots and STEM education was founded in the reviewed studies, it is worth further investigating the disciplines in which STEM activities have been conducted. This current review found that technology and engineering were the subject areas of most concern to researchers, while science and mathematics did not attract as much attention. This situation can be interpreted as an inadequate evaluation of R-STEM education. In other words, although those studies aimed at the interdisciplinary subject, most assessments and evaluations were monodisciplinary and targeted only knowledge. Therefore, it is necessary to carry out further studies in these insufficient subject areas to measure and answer the potential of robots in every STEM field and its integration. Moreover, the broadly consistent reporting of robotics generally supporting STEM content could impact practitioners only to employ robots in the mainstream STEM educational environment. Until that point, very few studies had investigated the prominence use of robots in various and large-scale multidiscipline studies (e.g., Christensen et al., 2015 ).
Another finding of the reviewed studies was the characteristic of robot-STEM integration. Researchers and practitioners must first answer why and how integrated R-STEM could be embodied in the teaching–learning process. For example, when robots are used as a learning tool to achieve STEM learning objectives, practitioners are suggested to have application knowledge. At the same time, researchers are advised to understand the pedagogical theories so that R-STEM integration can be flexibly merged into learning content. This means that the learning design should offer students’ existing knowledge of the immersive experience in dealing with robots and STEM activities that assist them in being aware of their ideas, then building their knowledge. In such a learning experience, students will understand the concept of STEM more deeply by engaging with robots. Moreover, demonstration of R-STEM learning is not only about the coherent understanding of the content knowledge. Practitioners need to apply both flexible subject-matter knowledge (e.g., central facts, concepts and procedures in the core concept of knowledge), and pedagogical content knowledge, which specific knowledge of approaches that are suitable for organizing and delivering topic-specific content, to the discipline of R-STEM education. Consequently, practitioners are required to understand the nature of robots and STEM through the content and practices, for example, taking the lead in implementing innovation through subject area instruction, developing collaboration that enriches R-STEM learning experiences for students, and being reflective practitioners by using students’ learning artifacts to inform and revise practices.
Limitations and recommendations for future research
Overall, future research could explore the great potential of using robots in education to build students’ knowledge and skills when pursuing learning objectives. It is believed that the findings from this study will provide insightful information for future research.
The articles reviewed in this study were limited to journals indexed in the WOS database and R-STEM education-related SSCI articles. However, other databases and indexes (e.g., SCOPUS, and SCI) could be considered. In addition, the number of studies analyzed was relatively small. Further research is recommended to extend the review duration to cover the publications in the coming years. The results of this review study have provided directions for the research area of STEM education and robotics. Specifically, robotics combined with STEM education activities should aim to foster the development of creativity. Future research may aim to develop skills in specific areas such as robotics STEM education combined with the humanities, but also skills in other humanities disciplines across learning activities, social/interactive skills, and general guidelines for learners at different educational levels. Educators can design career readiness activities to help learners build self-directed learning plans.
Availability of data and materials
Data sharing not applicable to this article as no datasets were generated or analyzed during the current study.
Abbreviations
Science, technology, engineering, and mathematics
Robotics-based STEM
Project-based learning
References marked with an asterisk indicate studies included in the systematic review
Adams, R., Evangelou, D., English, L., De Figueiredo, A. D., Mousoulides, N., Pawley, A. L., Schiefellite, C., Stevens, R., Svinicki, M., Trenor, J. M., & Wilson, D. M. (2011). Multiple perspectives on engaging future engineers. Journal of Engineering Education, 100 (1), 48–88. https://doi.org/10.1002/j.2168-9830.2011.tb00004.x
Article Google Scholar
Anwar, S., Bascou, N. A., Menekse, M., & Kardgar, A. (2019). A systematic review of studies on educational robotics. Journal of Pre-College Engineering Education Research (j-PEER), 9 (2), 19–24. https://doi.org/10.7771/2157-9288.1223
Atman Uslu, N., Yavuz, G. Ö., & KoçakUsluel, Y. (2022). A systematic review study on educational robotics and robots. Interactive Learning Environments . https://doi.org/10.1080/10494820.2021.2023890
*Ayar, M. C. (2015). First-hand experience with engineering design and career interest in engineering: An informal STEM education case study. Educational Sciences Theory & Practice, 15 (6), 1655–1675. https://doi.org/10.12738/estp.2015.6.0134
*Barak, M., & Assal, M. (2018). Robotics and STEM learning: Students’ achievements in assignments according to the P3 Task Taxonomy—Practice, problem solving, and projects. International Journal of Technology and Design Education, 28 (1), 121–144. https://doi.org/10.1007/s10798-016-9385-9
Bargagna, S., Castro, E., Cecchi, F., Cioni, G., Dario, P., Dell’Omo, M., Di Lieto, M. C., Inguaggiato, E., Martinelli, A., Pecini, C., & Sgandurra, G. (2019). Educational robotics in down syndrome: A feasibility study. Technology, Knowledge and Learning, 24 (2), 315–323. https://doi.org/10.1007/s10758-018-9366-z
*Barker, B. S., Nugent, G., & Grandgenett, N. F. (2014). Examining fidelity of program implementation in a STEM-oriented out-of-school setting. International Journal of Technology and Design Education, 24 (1), 39–52. https://doi.org/10.1007/s10798-013-9245-9
Behrens, A., Atorf, L., Schwann, R., Neumann, B., Schnitzler, R., Balle, J., Herold, T., Telle, A., Noll, T. G., Hameyer, K., & Aach, T. (2010). MATLAB meets LEGO Mindstorms—A freshman introduction course into practical engineering. IEEE Transactions on Education, 53 (2), 306–317. https://doi.org/10.1109/TE.2009.2017272
Belpaeme, T., Kennedy, J., Ramachandran, A., Scassellati, B., Tanaka, F. (2018). Social robots for education: A review. Science Robotics, 3 (21), eaat5954. https://doi.org/10.1126/scirobotics.aat5954
Benitti, F. B. V. (2012). Exploring the educational potential of robotics in schools: A systematic review. Computers & Education, 58 (3), 978–988. https://doi.org/10.1016/j.compedu.2011.10.006
*Bernstein, D., Mutch-Jones, K., Cassidy, M., & Hamner, E. (2022). Teaching with robotics: Creating and implementing integrated units in middle school subjects. Journal of Research on Technology in Education . https://doi.org/10.1080/15391523.2020.1816864
Bers, M. U. (2008). Blocks to robots learning with technology in the early childhood classroom . Teachers College Press.
Google Scholar
Blumenfeld, P. C., Soloway, E., Marx, R. W., Krajcik, J. S., Guzdial, M., & Palincsar, A. (1991). Motivating project-based learning: sustaining the doing, supporting the learning. Educational Psychologist, 26 (3–4), 369–398. https://doi.org/10.1080/00461520.1991.9653139
Bryan, L. A., Moore, T. J., Johnson, C. C., & Roehrig, G. H. (2015). Integrated STEM education. In C. C. Johnson, E. E. Peters-Burton, & T. J. Moore (Eds.), STEM road map: A framework for integrated STEM education (pp. 23–37). Routledge.
Chapter Google Scholar
*Casey, J. E., Gill, P., Pennington, L., & Mireles, S. V. (2018). Lines, roamers, and squares: Oh my! using floor robots to enhance Hispanic students’ understanding of programming. Education and Information Technologies, 23 (4), 1531–1546. https://doi.org/10.1007/s10639-017-9677-z
*Castro, E., Cecchi, F., Valente, M., Buselli, E., Salvini, P., & Dario, P. (2018). Can educational robotics introduce young children to robotics and how can we measure it?. Journal of Computer Assisted Learning, 34 (6), 970–977. https://doi.org/10.1111/jcal.12304
Çetin, M., & Demircan, H. Ö. (2020). Empowering technology and engineering for STEM education through programming robots: A systematic literature review. Early Child Development and Care, 190 (9), 1323–1335. https://doi.org/10.1080/03004430.2018.1534844
*Chang, C. C., & Chen, Y. (2020). Cognition, attitude, and interest in cross-disciplinary i-STEM robotics curriculum developed by thematic integration approaches of webbed and threaded models: A concurrent embedded mixed methods study. Journal of Science Education and Technology, 29 , 622–634. https://doi.org/10.1007/s10956-020-09841-9
*Chang, C. C., & Chen, Y. (2022). Using mastery learning theory to develop task-centered hands-on STEM learning of Arduino-based educational robotics: Psychomotor performance and perception by a convergent parallel mixed method. Interactive Learning Environments . https://doi.org/10.1080/10494820.2020.1741400
*Chapman, A., Rodriguez, F. D., Pena, C., Hinojosa, E., Morales, L., Del Bosque, V., Tijerina, Y., & Tarawneh, C. (2020). “Nothing is impossible”: Characteristics of Hispanic females participating in an informal STEM setting. Cultural Studies of Science Education, 15 , 723–737. https://doi.org/10.1007/s11422-019-09947-6
Chen, M. R. A., Hwang, G. J., Majumdar, R., Toyokawa, Y., & Ogata, H. (2021a). Research trends in the use of E-books in English as a foreign language (EFL) education from 2011 to 2020: A bibliometric and content analysis. Interactive Learning Environments . https://doi.org/10.1080/10494820.2021.1888755
Chen, Y. L., Huang, L. F., & Wu, P. C. (2021b). Preservice preschool teachers’ self-efficacy in and need for STEM education professional development: STEM pedagogical belief as a mediator. Early Childhood Education Journal, 49 (2), 137–147.
Chesloff, J. D. (2013). STEM education must start in early childhood. Education Week, 32 (23), 27–32.
*Chiang, F. K., Liu, Y. Q., Feng, X., Zhuang, Y., & Sun, Y. (2020). Effects of the world robot Olympiad on the students who participate: A qualitative study. Interactive Learning Environments . https://doi.org/10.1080/10494820.2020.1775097
*Christensen, R., Knezek, G., & Tyler-Wood, T. (2015). Alignment of hands-on STEM engagement activities with positive STEM dispositions in secondary school students. Journal of Science Education and Technology, 24 (6), 898–909. https://doi.org/10.1007/s10956-015-9572-6
Cohen, J. (1960). A coefficient of agreement for nominal scales. Educational and Psychological Measurement, 20 , 37–46. https://doi.org/10.1177/001316446002000104
Conde, M. Á., Rodríguez-Sedano, F. J., Fernández-Llamas, C., Gonçalves, J., Lima, J., & García-Peñalvo, F. J. (2021). Fostering STEAM through challenge-based learning, robotics, and physical devices: A systematic mapping literature review. Computer Applications in Engineering Education, 29 (1), 46–65. https://doi.org/10.1002/cae.22354
*Convertini, J. (2021). An interdisciplinary approach to investigate preschool children’s implicit inferential reasoning in scientific activities. Research in Science Education, 51 (1), 171–186. https://doi.org/10.1007/s11165-020-09957-3
Creswell, J. W., & Clark, V. L. P. (2013). Designing and conducting mixed methods research (3rd ed.). Thousand Oaks: Sage Publications Inc.
Creswell, J. W., Plano-Clark, V. L., Gutmann, M. L., & Hanson, W. E. (2003). Advanced mixed methods research designs. Handbook of mixed methods in social and behavioral research. Sage.
Erdoğan, N., Navruz, B., Younes, R., & Capraro, R. M. (2016). Viewing how STEM project-based learning influences students’ science achievement through the implementation lens: A latent growth modeling. EURASIA Journal of Mathematics, Science & Technology Education, 12 (8), 2139–2154. https://doi.org/10.12973/eurasia.2016.1294a
Evripidou, S., Georgiou, K., Doitsidis, L., Amanatiadis, A. A., Zinonos, Z., & Chatzichristofis, S. A. (2020). Educational robotics: Platforms, competitions and expected learning outcomes. IEEE Access, 8 , 219534–219562. https://doi.org/10.1109/ACCESS.2020.3042555
Ferreira, N. F., Araujo, A., Couceiro, M. S., & Portugal, D. (2018). Intensive summer course in robotics–Robotcraft. Applied Computing and Informatics, 16 (1/2), 155–179. https://doi.org/10.1016/j.aci.2018.04.005
Fournier-Viger, P., Nkambou, R., Nguifo, E. M., Mayers, A., & Faghihi, U. (2013). A multiparadigm intelligent tutoring system for robotic arm training. IEEE Transactions on Learning Technologies, 6 (4), 364–377. https://doi.org/10.1109/TLT.2013.27
Fu, Q. K., & Hwang, G. J. (2018). Trends in mobile technology-supported collaborative learning: A systematic review of journal publications from 2007 to 2016. Computers & Education, 119 , 129–143. https://doi.org/10.1016/j.compedu.2018.01.004
García-Martínez, I., Tadeu, P., Montenegro-Rueda, M., & Fernández-Batanero, J. M. (2020). Networking for online teacher collaboration. Interactive Learning Environments . https://doi.org/10.1080/10494820.2020.1764057
*Gomoll, A., Hmelo-Silver, C. E., Šabanović, S., & Francisco, M. (2016). Dragons, ladybugs, and softballs: Girls’ STEM engagement with human-centered robotics. Journal of Science Education and Technology, 25 (6), 899–914. https://doi.org/10.1007/s10956-016-9647-z
*Gomoll, A. S., Hmelo-Silver, C. E., Tolar, E., Šabanovic, S., & Francisco, M. (2017). Moving apart and coming together: Discourse, engagement, and deep learning. Educational Technology and Society, 20 (4), 219–232.
*Guven, G., KozcuCakir, N., Sulun, Y., Cetin, G., & Guven, E. (2020). Arduino-assisted robotics coding applications integrated into the 5E learning model in science teaching. Journal of Research on Technology in Education . https://doi.org/10.1080/15391523.2020.1812136
Han, J., Jo, M., Hyun, E., & So, H. J. (2015). Examining young children’s perception toward augmented reality-infused dramatic play. Educational Technology Research and Development, 63 (3), 455–474. https://doi.org/10.1007/s11423-015-9374-9
Hansen, M. (2014). Characteristics of schools successful in STEM: Evidence from two states’ longitudinal data. Journal of Educational Research, 107 (5), 374–391. https://doi.org/10.1080/00220671.2013.823364
*Hennessy Elliott, C. (2020). “Run it through me:” Positioning, power, and learning on a high school robotics team. Journal of the Learning Sciences, 29 (4–5), 598–641. https://doi.org/10.1080/10508406.2020.1770763
Hong, Z. W., Huang, Y. M., Hsu, M., & Shen, W. W. (2016). Authoring robot-assisted instructional materials for improving learning performance and motivation in EFL classrooms. Journal of Educational Technology & Society, 19 (1), 337–349.
Hsiao, H. S., Chang, C. S., Lin, C. Y., & Hsu, H. L. (2015). “iRobiQ”: The influence of bidirectional interaction on kindergarteners’ reading motivation, literacy, and behavior. Interactive Learning Environments, 23 (3), 269–292. https://doi.org/10.1080/10494820.2012.745435
Huang, B., Jong, M. S. Y., Tu, Y. F., Hwang, G. J., Chai, C. S., & Jiang, M. Y. C. (2022). Trends and exemplary practices of STEM teacher professional development programs in K-12 contexts: A systematic review of empirical studies. Computers & Education . https://doi.org/10.1016/j.compedu.2022.104577
Hwang, G. J., & Tsai, C. C. (2011). Research trends in mobile and ubiquitous learning: A review of publications in selected journals from 2001 to 2010. British Journal of Educational Technology, 42 (4), E65–E70.
Hwang, G. J., Xie, H., Wah, B. W., & Gašević, D. (2020). Vision, challenges, roles and research issues of artificial intelligence in education. Computers and Education: Artificial Intelligence, 1 , 100001. https://doi.org/10.1016/j.caeai.2020.100001
Hynes, M. M., Mathis, C., Purzer, S., Rynearson, A., & Siverling, E. (2017). Systematic review of research in P-12 engineering education from 2000–2015. International Journal of Engineering Education, 33 (1), 453–462.
Iio, T., Maeda, R., Ogawa, K., Yoshikawa, Y., Ishiguro, H., Suzuki, K., Aoki, T., Maesaki, M., & Hama, M. (2019). Improvement of Japanese adults’ English speaking skills via experiences speaking to a robot. Journal of Computer Assisted Learning, 35 (2), 228–245. https://doi.org/10.1111/jcal.12325
*Jaipal-Jamani, K., & Angeli, C. (2017). Effect of robotics on elementary preservice teachers’ self-efficacy, science learning, and computational thinking. Journal of Science Education and Technology, 26 (2), 175–192. https://doi.org/10.1007/s10956-016-9663-z
Johnson, B., & Christensen, L. (2000). Educational research: Quantitative and qualitative approaches . Allyn & Bacon.
Jou, M., Chuang, C. P., & Wu, Y. S. (2010). Creating interactive web-based environments to scaffold creative reasoning and meaningful learning: From physics to products. Turkish Online Journal of Educational Technology-TOJET, 9 (4), 49–57.
Jung, S., & Won, E. (2018). Systematic review of research trends in robotics education for young children. Sustainability, 10 (4), 905. https://doi.org/10.3390/su10040905
Kelley, T. R., & Knowles, J. G. (2016). A conceptual framework for integrated STEM education. International Journal of STEM Education, 3 , 11. https://doi.org/10.1186/s40594-016-0046-z
Kennedy, J., Baxter, P., & Belpaeme, T. (2015). Comparing robot embodiments in a guided discovery learning interaction with children. International Journal of Social Robotics, 7 (2), 293–308. https://doi.org/10.1007/s12369-014-0277-4
*Kim, C., Kim, D., Yuan, J., Hill, R. B., Doshi, P., & Thai, C. N. (2015). Robotics to promote elementary education pre-service teachers’ STEM engagement, learning, and teaching. Computers and Education., 91 , 14–31. https://doi.org/10.1016/j.compedu.2015.08.005
*Kim, C. M., Yuan, J., Vasconcelos, L., Shin, M., & Hill, R. B. (2018). Debugging during block-based programming. Instructional Science, 46 (5), 767–787. https://doi.org/10.1007/s11251-018-9453-5
*Konijn, E. A., & Hoorn, J. F. (2020). Robot tutor and pupils’ educational ability: Teaching the times tables. Computers and Education, 157 , 103970. https://doi.org/10.1016/j.compedu.2020.103970
Köse, H., Uluer, P., Akalın, N., Yorgancı, R., Özkul, A., & Ince, G. (2015). The effect of embodiment in sign language tutoring with assistive humanoid robots. International Journal of Social Robotics, 7 (4), 537–548. https://doi.org/10.1007/s12369-015-0311-1
Kucuk, S., Aydemir, M., Yildirim, G., Arpacik, O., & Goktas, Y. (2013). Educational technology research trends in Turkey from 1990 to 2011. Computers & Education, 68 , 42–50. https://doi.org/10.1016/j.compedu.2013.04.016
Lamptey, D. L., Cagliostro, E., Srikanthan, D., Hong, S., Dief, S., & Lindsay, S. (2021). Assessing the impact of an adapted robotics programme on interest in science, technology, engineering and mathematics (STEM) among children with disabilities. International Journal of Disability, Development and Education, 68 (1), 62–77. https://doi.org/10.1080/1034912X.2019.1650902
*Leonard, J., Buss, A., Gamboa, R., Mitchell, M., Fashola, O. S., Hubert, T., & Almughyirah, S. (2016). Using robotics and game design to enhance children’s self-efficacy, STEM attitudes, and computational thinking skills. Journal of Science Education and Technology, 25 (6), 860–876. https://doi.org/10.1007/s10956-016-9628-2
*Leonard, J., Mitchell, M., Barnes-Johnson, J., Unertl, A., Outka-Hill, J., Robinson, R., & Hester-Croff, C. (2018). Preparing teachers to engage rural students in computational thinking through robotics, game design, and culturally responsive teaching. Journal of Teacher Education, 69 (4), 386–407. https://doi.org/10.1177/0022487117732317
*Li, Y., Huang, Z., Jiang, M., & Chang, T. W. (2016). The effect on pupils’ science performance and problem-solving ability through Lego: An engineering design-based modeling approach. Educational Technology and Society, 19 (3), 143–156. https://doi.org/10.2307/jeductechsoci.19.3.14
Lin, H. C., & Hwang, G. J. (2019). Research trends of flipped classroom studies for medical courses: A review of journal publications from 2008 to 2017 based on the technology-enhanced learning model. Interactive Learning Environments, 27 (8), 1011–1027. https://doi.org/10.1080/10494820.2018.1467462
*Luo, W., Wei, H. R., Ritzhaupt, A. D., Huggins-Manley, A. C., & Gardner-McCune, C. (2019). Using the S-STEM survey to evaluate a middle school robotics learning environment: Validity evidence in a different context. Journal of Science Education and Technology, 28 (4), 429–443. https://doi.org/10.1007/s10956-019-09773-z
*Ma, H. L., Wang, X. H., Zhao, M., Wang, L., Wang, M. R., & Li, X. J. (2020). Impact of robotic instruction with a novel inquiry framework on primary schools students. International Journal of Engineering Education, 36 (5), 1472–1479.
Margot, K. C., & Kettler, T. (2019). Teachers’ perception of STEM integration and education: A systematic literature review. International Journal of STEM Education, 6 (1), 1–16. https://doi.org/10.1186/s40594-018-0151-2
Martín-Páez, T., Aguilera, D., Perales-Palacios, F. J., & Vílchez-González, J. M. (2019). What are we talking about when we talk about STEM education? A review of literature. Science Education, 103 (4), 799–822. https://doi.org/10.1002/sce.21522
*McDonald, S., & Howell, J. (2012). Watching, creating and achieving: Creative technologies as a conduit for learning in the early years. British Journal of Educational Technology, 43 (4), 641–651. https://doi.org/10.1111/j.1467-8535.2011.01231.x
*Meyers, K., Goodrich, V. E., Brockman, J. B., & Caponigro, J. (2012). I2D2: Imagination, innovation, discovery, and design. In 2012 ASEE annual conference & exposition (pp. 25–707). https://doi.org/10.18260/1-2--21464
Moher, D., Liberati, A., Tetzlaff, J., Altman, D. G., Prisma Group. (2009). Preferred reporting items for systematic reviews and meta-analyses: The PRISMA statement. PLoS Medicine . https://doi.org/10.1371/journal.pmed.1000097
Moomaw, S. (2012). STEM Begins in the Early Years. School Science and Mathematics, 112 (2), 57–58. https://doi.org/10.1111/j.1949-8594.2011.00119.x
Nel, H., Ettershank, M., & Venter, J. (2017). AfrikaBot: Design of a robotics challenge to promote STEM in Africa. In M. Auer, D. Guralnick, & J. Uhomoibhi (Eds.), Interactive collaborative learning. ICL 2016. Advances in intelligent systems and computing. Springer. https://doi.org/10.1007/978-3-319-50340-0_44
*Newton, K. J., Leonard, J., Buss, A., Wright, C. G., & Barnes-Johnson, J. (2020). Informal STEM: Learning with robotics and game design in an urban context. Journal of Research on Technology in Education, 52 (2), 129–147. https://doi.org/10.1080/15391523.2020.1713263
Okita, S. Y. (2014). The relative merits of transparency: Investigating situations that support the use of robotics in developing student learning adaptability across virtual and physical computing platforms. British Journal of Educational Technology, 45 (5), 844–862. https://doi.org/10.1111/bjet.12101
Özdemir, D., & Karaman, S. (2017). Investigating interactions between students with mild mental retardation and humanoid robot in terms of feedback types. Education and Science, 42 (191), 109–138. https://doi.org/10.15390/EB.2017.6948
Özüorçun, N. Ç., & Bicen, H. (2017). Does the inclusion of robots affect engineering students’ achievement in computer programming courses? Eurasia Journal of Mathematics, Science and Technology Education, 13 (8), 4779–4787. https://doi.org/10.12973/eurasia.2017.00964a
*Pérez, S. E., & López, J. F. (2019). An ultra-low cost line follower robot as educational tool for teaching programming and circuit’s foundations. Computer Applications in Engineering Education, 27 (2), 288–302. https://doi.org/10.1002/cae.22074
*Phamduy, P., Leou, M., Milne, C., & Porfiri, M. (2017). An interactive robotic fish exhibit for designed settings in informal science learning. IEEE Transactions on Education, 60 (4), 273–280. https://doi.org/10.1109/TE.2017.2695173
*Ryan, M., Gale, J., & Usselman, M. (2017). Integrating engineering into core science instruction: Translating NGSS principles into practice through iterative curriculum design. International Journal of Engineering Education., 33 (1), 321–331.
*Sen, C., Ay, Z. S., & Kiray, S. A. (2021). Computational thinking skills of gifted and talented students in integrated STEM activities based on the engineering design process: The case of robotics and 3D robot modeling. Thinking Skills and Creativity, 42 , 100931. https://doi.org/10.1016/j.tsc.2021.100931
Spolaôr, N., & Benitti, F. B. V. (2017). Robotics applications grounded in learning theories on tertiary education: A systematic review. Computers & Education, 112 , 97–107. https://doi.org/10.1016/j.compedu.2017.05.001
*Stewart, W. H., Baek, Y., Kwid, G., & Taylor, K. (2021). Exploring factors that influence computational thinking skills in elementary students’ collaborative robotics. Journal of Educational Computing Research, 59 (6), 1208–1239. https://doi.org/10.1177/0735633121992479
*Sullivan, A., & Bers, M. U. (2016). Robotics in the early childhood classroom: Learning outcomes from an 8-week robotics curriculum in pre-kindergarten through second grade. International Journal of Technology and Design Education, 26 (1), 3–20. https://doi.org/10.1007/s10798-015-9304-5
*Sullivan, A., & Bers, M. U. (2019). Investigating the use of robotics to increase girls’ interest in engineering during early elementary school. International Journal of Technology and Design Education, 29 , 1033–1051. https://doi.org/10.1007/s10798-018-9483-y
*Taylor, M. S. (2018). Computer programming with pre-K through first-grade students with intellectual disabilities. Journal of Special Education, 52 (2), 78–88. https://doi.org/10.1177/0022466918761120
Taylor, R. P. (1980). Introduction. In R. P. Taylor (Ed.), The computer in school: Tutor, tool, tutee (pp. 1–10). Teachers College Press.
Tselegkaridis, S., & Sapounidis, T. (2021). Simulators in educational robotics: A review. Education Sciences, 11 (1), 11. https://doi.org/10.3390/educsci11010011
*Üçgül, M., & Altıok, S. (2022). You are an astroneer: The effects of robotics camps on secondary school students’ perceptions and attitudes towards STEM. International Journal of Technology and Design Education, 32 (3), 1679–1699. https://doi.org/10.1007/s10798-021-09673-7
*Ucgul, M., & Cagiltay, K. (2014). Design and development issues for educational robotics training camps. International Journal of Technology and Design Education, 24 (2), 203–222. https://doi.org/10.1007/s10798-013-9253-9
van den Berghe, R., Verhagen, J., Oudgenoeg-Paz, O., Van der Ven, S., & Leseman, P. (2019). Social robots for language learning: A review. Review of Educational Research, 89 (2), 259–295. https://doi.org/10.3102/0034654318821286
Zhang, Y., Luo, R., Zhu, Y., & Yin, Y. (2021). Educational robots improve K-12 students’ computational thinking and STEM attitudes: Systematic review. Journal of Educational Computing Research, 59 (7), 1450–1481. https://doi.org/10.1177/0735633121994070
Zhong, B., & Xia, L. (2020). A systematic review on exploring the potential of educational robotics in mathematics education. International Journal of Science and Mathematics Education, 18 (1), 79–101. https://doi.org/10.1007/s10763-018-09939-y
Download references
Acknowledgements
The authors would like to express their gratefulness to the three anonymous reviewers for providing their precious comments to refine this manuscript.
This study was supported by the Ministry of Science and Technology of Taiwan under contract numbers MOST-109-2511-H-011-002-MY3 and MOST-108-2511-H-011-005-MY3; National Science and Technology Council (TW) (NSTC 111-2410-H-031-092-MY2); Soochow University (TW) (111160605-0014). Any opinions, findings, conclusions, and/or recommendations expressed in this paper are those of the authors and do not necessarily reflect the views of Ministry of Science and Technology of Taiwan.
Author information
Authors and affiliations.
Graduate Institute of Digital Learning and Education, National Taiwan University of Science and Technology, 43, Sec. 4, Keelung Rd., Taipei, 106, Taiwan
Darmawansah Darmawansah, Gwo-Jen Hwang & Jia-Cing Liang
Department of English Language and Literature, Soochow University, Q114, No. 70, Linhsi Road, Shihlin District, Taipei, 111, Taiwan
Mei-Rong Alice Chen
Yuan Ze University, 135, Yuandong Road, Zhongli District, Taipei, Taiwan
Gwo-Jen Hwang
You can also search for this author in PubMed Google Scholar
Contributions
DD, MR and GJ conceptualized the study. MR wrote the outline and DD wrote draft. DD, MR and GJ contributed to the manuscript through critical reviews. DD, MR and GJH revised the manuscript. DD, MR and GJ finalized the manuscript. DD edited the manuscript. MR and GJ monitored the project and provided adequate supervision. DD, MR and JC contributed with data collection, coding, analyses and interpretation. All authors read and approved the final manuscript.
Corresponding author
Correspondence to Mei-Rong Alice Chen .
Ethics declarations
Competing interests.
The authors declare that they have no competing interests.
Additional information
Publisher's note.
Springer Nature remains neutral with regard to jurisdictional claims in published maps and institutional affiliations.
Supplementary Information
Additional file 1..
Coded papers.
Appendix 1. Summary of selected studies from the angle of research issue
# | Authors | Dimension | ||||
---|---|---|---|---|---|---|
Location | Sample size | Duration of intervention | Research methods | Research foci | ||
1 | Convertini ( ) | Italy | 21–40 | ≤ 1 day | Experimental design | Problem solving, collaboration or teamwork, and communication |
2 | Lamptey et. al. ( ) | Canada | 41–60 | ≤ 8 weeks | Mixed method | Satisfaction or interest, and learning perceptions |
3 | Üçgül and Altıok ( ) | Turkey | 41–60 | ≤ 1 day | Questionnaire or survey | Attitude and motivation, learning perceptions |
4 | Sen et. al. ( ) | Turkey | 1–20 | ≤ 4 weeks | Experimental design | Problem solving, critical thinking, logical thinking, creativity, collaboration or teamwork, and communication |
5 | Stewart et. al. ( ) | USA | > 80 | ≤ 6 months | Mixed method | Higher order thinking skills, problem-solving, technology acceptance, attitude and motivation, and learning perceptions |
6 | Bernstein et. al. ( ) | USA | 1–20 | ≤ 1 day | Questionnaire or survey | Attitude and motivation, and learning perceptions |
7 | Chang and Chen ( ) | Taiwan | 41–60 | ≤ 8 weeks | Mixed method | Learning performance, problem-solving, satisfaction or interest, and operational skill |
8 | Chang and Chen ( ) | Taiwan | 41–60 | ≤ 8 weeks | Experimental design | Learning perceptions, and operational skill |
9 | Chapman et al. ( ) | USA | > 80 | ≤ 8 weeks | Mixed method | Learning performance, and learning perceptions |
10 | Chiang et. al. ( ) | China | 41–60 | ≤ 4 weeks | Questionnaire or survey | Creativity, and self-efficacy and confidence |
11 | Guven et. al. ( ) | Turkey | 1–20 | ≤ 6 months | Mixed method | Creativity, technology acceptance, attitude and motivation, self-efficacy or confidence, satisfaction or interest, and learning perception |
12 | Hennessy Elliott ( ) | USA | 1–20 | ≤ 12 months | Experimental design | Collaboration, communication, and preview situation |
13 | Konijn and Hoorn ( ) | Netherlands | 41–60 | ≤ 4 weeks | Experimental design | Learning performance, and learning behavior |
14 | Ma et. al. ( ) | China | 41–60 | ≤ 6 months | Mixed method | Learning performance, learning perceptions, and learning behavior |
15 | Newton et. al. ( ) | USA | > 80 | ≤ 6 months | Mixed method | Attitude and motivation, and self-efficacy and confidence |
16 | Luo et. al. ( ) | USA | 41–60 | ≤ 4 weeks | Questionnaire or survey | Technology acceptance, attitude and motivation, and self-efficacy |
17 | Pérez and López ( ) | Mexico | 21–40 | ≤ 6 months | System development | Operational skill |
18 | Sullivan and Bers ( ) | USA | > 80 | ≤ 8 weeks | Mixed method | Attitude and motivation, satisfaction or interest, and learning behavior |
19 | Barak and Assal ( ) | Israel | 21–40 | ≤ 6 months | Mixed method | Learning performance, technology acceptance, self-efficacy, and satisfaction or interest |
20 | Castro et. al. ( ) | Italy | > 80 | ≤ 8 weeks | Questionnaire or survey | Learning performance, and self-efficacy |
21 | Casey et. al. ( ) | USA | > 80 | ≤ 12 months | Questionnaire or survey | Learning satisfaction |
22 | Kim et. al. ( ) | USA | 1–20 | ≤ 4 weeks | Questionnaire or survey | Problem solving, and preview situation |
23 | Leonard et. al. ( ) | USA | 41–60 | ≤ 12 months | Questionnaire or survey | Learning performance, self-efficacy, and learning perceptions |
24 | Taylor ( ) | USA | 1–20 | ≤ 1 day | Experimental design | Learning performance, and preview situation |
25 | Gomoll et. al. ( ) | USA | 21–40 | ≤ 8 weeks | Experimental design | Problem solving, collaboration, communication |
26 | Jaipal-Jamani and Angeli ( ) | Canada | 21–40 | ≤ 4 weeks | Mixed method | Learning performance, self-efficacy, and satisfaction or interest |
27 | Phamduy et. al. ( ) | USA | > 80 | ≤ 4 weeks | Mixed method | Satisfaction or interest, and learning behavior |
28 | Ryan et. al. ( ) | USA | 1–20 | ≤ 12 months | Questionnaire or survey | Learning perceptions |
29 | Gomoll et. al. ( ) | USA | 21–40 | ≤ 6 months | Experimental design | Satisfaction or interest, and learning perceptions |
30 | Leonard et. al. ( ) | USA | 61–80 | ≤ 4 weeks | Mixed method | Attitude and motivation, and self-efficacy |
31 | Li et. al. ( ) | China | 21–40 | ≤ 8 weeks | Experimental design | Learning performance, and problem-solving, |
32 | Sullivan and Bers ( ) | USA | 41–60 | ≤ 8 weeks | Experimental design | Learning performance, and operational skill |
33 | Ayar ( ) | Turkey | > 80 | ≤ 4 weeks | Questionnaire or survey | Attitude and motivation, satisfaction or interest, and learning perceptions |
34 | Christensen et. al. ( ) | USA | > 80 | ≤ 6 months | Questionnaire or survey | Technology acceptance, satisfaction or interest, and learning perceptions |
35 | Kim et al. ( ) | USA | 1–20 | ≤ 4 weeks | Mixed method | Learning performance, satisfaction or interest, and learning perceptions |
36 | Barker et. al. ( ) | USA | 21–40 | ≤ 4 weeks | Questionnaire or survey | Technology acceptance, attitude and motivation, and learning perceptions |
37 | Ucgul and Cagiltay ( ) | Turkey | 41–60 | ≤ 4 weeks | Questionnaire or survey | Learning performance, satisfaction or interest, and learning perceptions |
38 | McDonald and Howell ( ) | Australia | 1–20 | ≤ 8 weeks | Mixed method | Learning performance, operational skills, and learning behavior |
39 | Meyers et. al. ( ) | USA | > 80 | ≤ 4 weeks | Questionnaire or survey | Learning perceptions |
Appendix 2. Summary of selected studies from the angles of interaction and application
# | Authors | Interaction | Application | ||||||
---|---|---|---|---|---|---|---|---|---|
Participants | Role of robot | Types of robot | Dominant STEM discipline | Contribution to STEM | Integration of robot and STEM | Pedagogical intervention | Educational objectives | ||
1 | Convertini ( ) | Preschool or Kindergarten | Tutee | LEGO (Mindstorms) | Engineering | Structure and construction | Context integration | Active construction | Learning and transfer skills |
2 | Lamptey et. al. ( ) | Non-specified | Tool | LEGO (Mindstorms) | Technology | Programming | Supporting content integration | Problem-based learning | Learning and transfer skills |
3 | Üçgül and Altıok ( ) | Junior high school students | Tool | LEGO (Mindstorms) | Technology | Programming | Content integration | Project-based learning | Creativity and motivation |
4 | Sen et. al. ( ) | Others (gifted and talented students) | Tutee | LEGO (Mindstorms) | Technology | Programming, and Mathematical methods | Supporting content integration | Problem-based learning | Learning and transfer skills |
5 | Stewart et. al. ( ) | Elementary school students | Tool | Botball robot | Technology | Programming, and power and dynamical system | Content integration | Project-based learning | Learning and transfer skills |
6 | Bernstein et. al. ( ) | In-service teachers | Tool | Non-specified | Science | Biomechanics | Content integration | Project-based learning | Teachers’ professional development |
7 | Chang and Chen ( ) | High school students | Tool | Arduino | Interdisciplinary | Basic Physics, Programming, Component design, and mathematical methods | Content integration | Project-based learning | Learning transfer and skills |
8 | Chang and Chen ( ) | High school students | Tool | Arduino | Interdisciplinary | Basic Physics, Programming, Component design, and mathematical methods | Content integration | Project-based learning | Learning transfer and skills |
9 | Chapman et. al. ( ) | Elementary, middle, and high school students | Tool | LEGO (Mindstorms) and Maglev trains | Engineering | Engineering | Content integration | Engaged learning | Learning transfer and skills |
10 | Chiang et. al. ( ) | Non-specified | Tool | LEGO (Mindstorms) | Technology | Non-specified | Context integration | Edutainment | Creativity and motivation |
11 | Guven et. al. ( ) | Elementary school students | Tutee | Arduino | Technology | Programming | Content integration | Constructivism | Creativity and motivation |
12 | Hennessy Elliott ( ) | Students and teachers | Tool | Non-specified | Technology | Non-specified | Supporting content integration | Collaborative learning | General benefits of educational robotics |
13 | Konijn and Hoorn ( ) | Elementary school students | Tutor | Nao robot | Mathematics | Mathematical methods | Supporting content integration | Engaged learning | Learning and transfer skills |
14 | Ma et. al. ( ) | Elementary school students | Tool | Microduino and Makeblock | Engineering | Non-specified | Content integration | Experiential learning | Learning and transfer skills |
15 | Newton et. al. ( ) | Elementary school students | Tool | LEGO (Mindstorms) | Technology | Programming | Supporting content integration | Active construction | Learning and transfer skills |
16 | Luo et. al. ( ) | Junior high or middle school | Tool | Vex robots | Interdisciplinary | Programming, Engineering, and Mathematics | Content integration | Constructivism | General benefits of educational robots |
17 | Pérez and López ( ) | High school students | Tutee | Arduino | Engineering | Programming, and mechanics | Content integration | Project-based learning | Learning and transfer skills |
18 | Sullivan and Bers ( ) | Kindergarten and Elementary school students | Tool | KIBO robots | Technology | Programming | Context integration | Project-based learning | Learning and transfer skills |
19 | Barak and Assal ( ) | High school students | Tool | Non-specified | Technology | Programming, mathematical methods | Content integration | Problem-based learning | Learning and transfer skills |
20 | Castro et. al. ( ) | Lower secondary | Tool | Bee-bot | Technology | Programming | Content integration | Problem-based learning | Learning and transfer skills |
21 | Casey et. al. ( ) | Elementary school students | Tool | Roamers robot | Technology | Programming | Content integration | Metacognitive learning | Learning and transfer skills |
22 | Kim et. al. ( ) | Pre-service teachers | Tool | Non-specified | Technology | Programming | Supporting content integration | Problem-based learning | Learning and transfer skills |
23 | Leonard et. al. ( ) | In-service teachers | Tool | LEGO (Mindstorms) | Technology | Programming | Supporting content integration | Project-based learning | Teachers’ professional development |
24 | Taylor ( ) | Kindergarten and elementary school students | Tool | Dash robot | Technology | Programming, | Content integration | Problem-based learning | Learning and transfer skills |
25 | Gomoll et. al. ( ) | Middle school students | Tool | iRobot create | Technology | Programming, and structure and construction | Content integration | Problem-based learning | Learning and transfer skills |
26 | Jaipal-Jamani and Angeli ( ) | Pre-service teachers | Tool | LEGO WeDo | Technology | Programming | Supporting content integration | Project-based learning | Learning and transfer skills |
27 | Phamduy et. al. ( ) | Non-specified | Tutee | Arduino | Science | Biology | Context integration | Edutainment | Diversity and broadening participation |
28 | Ryan et. al. ( ) | In-service teachers | Tool | LEGO (Mindstorms) | Engineering | Engineering | Content integration | Constructivism | Teacher’s professional development |
29 | Gomoll et. al. ( ) | Non-specified | Tool | iRobot create | Technology | Programming | Content integration | Project-based learning | Learning and transfer skill |
30 | Leonard et. al. ( ) | Middle school students | Tool | LEGO (Mindstorms) | Technology | Programming | Content integration | Project-based learning | Learning and transfer skill |
31 | Li et. al. ( ) | Elementary school students | Tool | LEGO Bricks | Engineering | Structure and construction | Supporting content integration | Project-based learning | General benefits of educational robotics |
32 | Sullivan and Bers ( ) | Kindergarten and Elementary school students | Tool | Kiwi Kits | Engineering | Digital signal process | Content integration | Project-based learning | Learning and transfer skill |
33 | Ayar ( ) | High school students | Tool | Nao robot | Engineering | Component design | Content integration | Edutainment | Creativity and 34motivation |
34 | Christensen et. al. ( ) | Middle and high school students | Tutee | Non-specified | Engineering | Engineering | Context integration | Edutainment | Creativity and motivation |
35 | Kim et. al. ( ) | Pre-service teachers | Tool | RoboRobo | Technology | Programming | Supporting content integration | Engaged learning | Teachers’ professional development |
36 | Barker et. al. ( ) | In-service teachers | Tool | LEGO (Mindstorms) | Technology | Geography information system, and programming | Supporting content integration | Constructivism | Creativity and motivation |
37 | Ucgul and Cagiltay ( ) | Elementary and Middle school students | Tool | LEGO (Mindstorms) | Technology | Programming, mechanics, and mathematics | Content integration | Project-based learning | General benefits of educational robots |
38 | McDonald and Howell ( ) | Elementary school students | Tool | LEGO WeDo | Technology | Programming, and students and construction | Content integration | Project-based learning | Learning and transfer skills |
39 | Meyers et. al. ( ) | Elementary school students | Tool | LEGO (Mindstorms) | Engineering | Engineering | Supporting content integration | Edutainment | Creativity and motivation |
Rights and permissions
Open Access This article is licensed under a Creative Commons Attribution 4.0 International License, which permits use, sharing, adaptation, distribution and reproduction in any medium or format, as long as you give appropriate credit to the original author(s) and the source, provide a link to the Creative Commons licence, and indicate if changes were made. The images or other third party material in this article are included in the article's Creative Commons licence, unless indicated otherwise in a credit line to the material. If material is not included in the article's Creative Commons licence and your intended use is not permitted by statutory regulation or exceeds the permitted use, you will need to obtain permission directly from the copyright holder. To view a copy of this licence, visit http://creativecommons.org/licenses/by/4.0/ .
Reprints and permissions
About this article
Cite this article.
Darmawansah, D., Hwang, GJ., Chen, MR.A. et al. Trends and research foci of robotics-based STEM education: a systematic review from diverse angles based on the technology-based learning model. IJ STEM Ed 10 , 12 (2023). https://doi.org/10.1186/s40594-023-00400-3
Download citation
Received : 11 May 2022
Accepted : 13 January 2023
Published : 10 February 2023
DOI : https://doi.org/10.1186/s40594-023-00400-3
Share this article
Anyone you share the following link with will be able to read this content:
Sorry, a shareable link is not currently available for this article.
Provided by the Springer Nature SharedIt content-sharing initiative
- STEM education
- Interdisciplinary projects
- Twenty-first century skills
What does a robotics engineer do?
Would you make a good robotics engineer? Take our career test and find your match with over 800 careers.
What is a Robotics Engineer?
A robotics engineer specializes in the design, development, and implementation of robotic systems and technologies. These engineers work at the intersection of mechanical, electrical, and computer engineering to create machines capable of performing tasks autonomously or semi-autonomously.
Robotics engineers are involved in the entire lifecycle of robotic systems, from conceptualization and design to programming, testing, and deployment. They may work on a wide range of applications, including industrial automation, medical robotics, autonomous vehicles, and consumer electronics. As the field of robotics continues to advance, robotics engineers contribute to innovations that have the potential to transform industries, improve daily life, and drive the future of automation and intelligent systems.
What does a Robotics Engineer do?
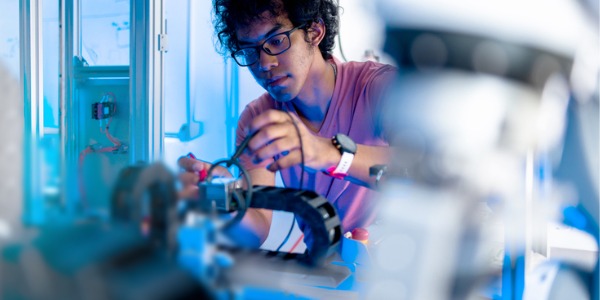
Duties and Responsibilities The duties and responsibilities of a robotic engineer can vary depending on the specific job and industry, but generally include the following:
- Design and Development: Collaborate with multidisciplinary teams to conceptualize robotic systems, creating detailed mechanical designs and electrical systems for components and end-effectors.
- Programming and Control: Write and implement sophisticated software code for robots, including motion control, path planning, and task execution; integrate and calibrate various sensors for environment perception.
- Testing and Validation: Build and rigorously test prototypes to validate design concepts, ensuring feasibility, and using simulation tools for virtual testing before physical implementation.
- Collaboration and Project Management: Work closely with mechanical, electrical, and software engineers, managing project timelines, budgets, and resources to ensure the successful and timely completion of robotic development projects.
- Research and Innovation: Stay abreast of advancements in robotics, AI, and automation, contributing to or leading research initiatives to push technological boundaries and enhance existing systems.
- Implementation and Deployment: Oversee the installation and integration of robotic systems into real-world environments, addressing specific challenges of deployment contexts.
- Compliance and Standards: Ensure robotic systems adhere to industry standards, safety regulations, and ethical considerations, maintaining comprehensive documentation of design specifications and testing procedures.
Types of Robotic Engineers Robotics engineering encompasses various specializations, each focusing on specific aspects of designing, building, and implementing robotic systems. Here are some types of robotic engineers:
- Mechanical Robotics Engineer: Specializes in designing the mechanical components of robotic systems, including the physical structures, joints, actuators, and end-effectors.
- Electrical Robotics Engineer: Focuses on the electrical systems of robots, designing circuits, sensors, and actuators to enable communication and control within the robotic framework.
- Software Robotics Engineer (Robotics Programmer): Specializes in writing and implementing the software code that controls the behavior, motion, and functionality of robotic systems.
- Robotic Controls Engineer: Concentrates on developing control algorithms to govern the movements and responses of robotic systems, ensuring precision and efficiency.
- Computer Vision Engineer: Specializes in creating algorithms and systems that enable robots to interpret visual information from cameras, lidar, and other sensors for perception and decision-making.
- Machine Learning Engineer for Robotics: Applies machine learning techniques to enhance the capabilities of robots, enabling them to adapt, learn from experience, and improve their performance over time.
- Autonomous Robotics Engineer: Focuses on developing robotic systems capable of autonomous operation, decision-making, and navigation without continuous human intervention.
- Human-Robot Interaction Engineer: Specializes in designing interfaces and systems that facilitate effective communication and collaboration between robots and humans.
- Robotics Research Scientist: Engages in scientific research to advance the field of robotics, contributing to the development of new technologies and methodologies.
- Bio-Inspired Robotics Engineer: Draws inspiration from biological systems to design robots that mimic or are inspired by natural organisms, exploring biomimicry in robotics.
- Swarm Robotics Engineer: Focuses on the coordination and cooperation of multiple robots working together in a swarm, studying collective behavior and decentralized control.
- Robotics System Integration Engineer: Specializes in integrating different components and subsystems of robotic systems to ensure seamless functionality and interoperability.
- Robotics Test Engineer: Focuses on developing and conducting tests to evaluate the performance, reliability, and safety of robotic systems.
- Robotics Project Manager: Manages the overall planning, execution, and delivery of robotic projects, coordinating efforts among various engineering disciplines.
- Robotics Ethicist: Examines the ethical implications of robotic technologies, ensuring responsible and ethical use of robots in various applications.
Are you suited to be a robotics engineer?
Robotics engineers have distinct personalities . They tend to be investigative individuals, which means they’re intellectual, introspective, and inquisitive. They are curious, methodical, rational, analytical, and logical. Some of them are also realistic, meaning they’re independent, stable, persistent, genuine, practical, and thrifty.
Does this sound like you? Take our free career test to find out if robotics engineer is one of your top career matches.
What is the workplace of a Robotics Engineer like?
The workplace of a robotics engineer is dynamic and can vary based on the specific industry, project requirements, and the engineer's role within a multidisciplinary team. Generally, robotics engineers find employment in diverse settings that leverage their skills in designing, developing, and implementing robotic systems.
One common workplace for robotics engineers is research and development laboratories or innovation centers. In these environments, engineers engage in cutting-edge research, pushing the boundaries of robotic technology. They collaborate with fellow researchers, scientists, and technologists to explore new concepts, experiment with prototypes, and contribute to advancements in the field.
Robotics engineers are also integral to manufacturing industries, particularly those employing automation. Here, engineers work in production facilities and assembly lines, designing and implementing robotic systems to optimize manufacturing processes. Their role includes ensuring that robots operate efficiently, safely, and with high precision, contributing to increased productivity and quality in manufacturing.
In technology companies specializing in robotics, engineers may find themselves in modern offices or engineering labs. These workplaces foster collaboration and innovation, providing engineers with access to state-of-the-art equipment, simulation tools, and testing facilities. They work on projects ranging from industrial automation to the development of robotic companions and autonomous systems.
For engineers focused on autonomous vehicles, drones, or other mobile robotic systems, the workplace extends to outdoor testing environments. These engineers may spend time in testing facilities, open fields, or specialized tracks, overseeing the deployment and performance evaluation of robotic vehicles.
In academia, robotics engineers may work within university research departments, engaging in both theoretical and practical aspects of robotics. They often mentor students, lead research projects, and contribute to the academic community's understanding of robotics technologies.
Regardless of the specific workplace, robotics engineers commonly collaborate with professionals from diverse backgrounds, including mechanical engineers, electrical engineers, software developers, and experts in various specialized fields. This interdisciplinary collaboration fosters a dynamic work environment where ideas are shared, problems are solved collectively, and innovative solutions are developed to address the challenges of creating advanced robotic systems.
The work of a robotics engineer may involve a combination of computer modeling, simulation, hands-on prototyping, and real-world testing. The field's evolving nature requires engineers to stay updated on the latest technologies and methodologies, making continuous learning a key aspect of their professional development.
Frequently Asked Questions
Engineering specializations and degrees.
- Aerospace Engineer
- Agricultural Engineer
- Architectural Engineer
- Artificial Intelligence Engineer
- Audio Engineer
- Automation Engineer
- Automotive Engineer
- Automotive Engineering Technician
- Big Data Engineer
- Biochemical Engineer
- Biofuel Engineer
- Biomedical Engineer
- Broadcast Engineer
- Chemical Engineer
- Civil Engineer
- Civil Engineering Technician
- Cloud Engineer
- Coastal Engineer
- Computer Engineer
- Computer Hardware Engineer
- Computer Vision Engineer
- Construction Engineer
- Control Engineer
- Data Engineer
- Digital Remastering Engineer
- Electrical Engineer
- Electromechanical Engineer
- Electronics Engineer
- Environmental Engineer
- Flight Engineer
- Fuel Cell Engineer
- Fuel Cell Technician
- Game Audio Engineer
- Geotechnical Engineer
- Geothermal Engineer
- Industrial Engineer
- Industrial Engineering Technician
- Laser Engineer
- Live Sound Engineer
- Locomotive Engineer
- Machine Learning Engineer
- Manufacturing Engineer
- Marine Engineer
- Mastering Engineer
- Mechanical Engineer
- Mechanical Engineering Technician
- Mechatronics Engineer
- Mining and Geological Engineer
- Mixing Engineer
- Nanosystems Engineer
- Nanotechnology Engineer
- Natural Language Processing Engineer
- Naval Engineer
- Nuclear Engineer
- Ocean Engineer
- Optical Engineer
- Paper Science Engineer
- Petroleum Engineer
- Photonics Engineer
- Plastics Engineer
- Power Engineer
- Product Safety Engineer
- Pulp and Paper Engineer
- Recording Engineer
- Robotics Engineer
- Sales Engineer
- Security Engineer
- Ship Engineer
- Software Engineer
- Software Quality Assurance Engineer
- Solar Engineer
- Stationary Engineer
- Structural Engineer
- Systems Engineer
- Transportation Engineer
- Urban Planning Engineer
- Water Engineer
- Water Resources Engineer
- Wind Energy Engineer
- Engineering
- Aerospace Engineering
- Agricultural Engineering
- Architectural Engineering
- Biochemical Engineering
- Biological Systems Engineering
- Biomedical Engineering
- Chemical Engineering
- Civil Engineering
- Computer Engineering
- Computer Hardware Engineering
- Computer Software Engineering
- Construction Engineering
- Electrical Engineering
- Electromechanical Engineering
- Engineering Mechanics
- Engineering Physics
- Engineering Science
- Environmental Engineering
- Geological Engineering
- Industrial Engineering
- Manufacturing Engineering
- Materials Science and Engineering
- Mechanical Engineering
- Naval Engineering
- Nuclear Engineering
- Ocean Engineering
- Optical Engineering
- Paper Science and Engineering
- Petroleum Engineering
- Plastics Engineering
- Pulp and Paper Engineering
- Robotics Engineering
- Sound Engineering
- Structural Engineering
- Surveying Engineering
- Systems Engineering
- Telecommunications Engineering
Continue reading

Virtual Tour
Experience University of Idaho with a virtual tour. Explore now
- Discover a Career
- Find a Major
- Experience U of I Life
More Resources
- Admitted Students
- International Students
Take Action
- Find Financial Aid
- View Deadlines
- Find Your Rep
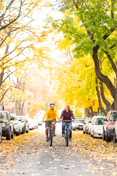
Helping to ensure U of I is a safe and engaging place for students to learn and be successful. Read about Title IX.
Get Involved
- Clubs & Volunteer Opportunities
- Recreation and Wellbeing
- Student Government
- Student Sustainability Cooperative
- Academic Assistance
- Safety & Security
- Career Services
- Health & Wellness Services
- Register for Classes
- Dates & Deadlines
- Financial Aid
- Sustainable Solutions
- U of I Library
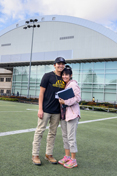
- Upcoming Events
Review the events calendar.
Stay Connected
- Vandal Family Newsletter
- Here We Have Idaho Magazine
- Living on Campus
- Campus Safety
- About Moscow
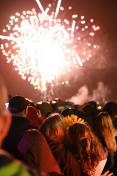
The largest Vandal Family reunion of the year. Check dates.
Benefits and Services
- Vandal Voyagers Program
- Vandal License Plate
- Submit Class Notes
- Make a Gift
- View Events
- Alumni Chapters
- University Magazine
- Alumni Newsletter
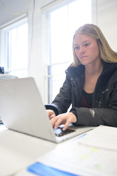
SlateConnect
U of I's web-based retention and advising tool provides an efficient way to guide and support students on their road to graduation. Login to SlateConnect.
Common Tools
- Administrative Procedures Manual (APM)
- Class Schedule
- OIT Tech Support
- Academic Dates & Deadlines
- U of I Retirees Association
- Faculty Senate
- Staff Council
College of Engineering
Campus locations.
Physical Address: Bruce M. Pitman Center 875 Perimeter Drive MS 4264 Moscow, ID 83844-4264 [email protected] uidaho.edu
Phone: 208-885-6111
Fax: 208-885-9119

University of Idaho College of Engineering students work directly with state-of-the-art mobile and full-size robots used in a variety of applications — from industrial to therapeutic!
Students train alongside faculty in multiple disciplines, including computer science, electrical engineering and mechanical engineering. Our programs are expanding undergraduate and graduate robotics education and research opportunities in cybersecurity, artificial intelligence, industrial automation, assistive robotics and more, with training facilities statewide.
Explore Degrees & Certificates
Offering robotics programs in computer science and mechanical engineering as well as Idaho’s first industrial robotics certificates.
View Programs
Center for Intelligent Industrial Robotics
Integrating new robotics research and training labs across the state to prepare students to fill the global manufacturing labor shortage.
Explore Center
Assistive Robotics Laboratory
Develop robotic devices that evaluate neurological impairment after stroke and gather data to refine therapeutic medicine and improve treatment.
Explore Lab
Vandal Robotics Club
Apply your skills and experience in programming, design, fabrication and critical thinking outside of the classroom!
Duke School of Nursing and Pratt School of Engineering Launch Collaborative Research Program
New trans-disciplinary partnership with Pratt School of Engineering provides pilot funding to support innovative, collaborative research projects that will contribute to the transformation of biomedical solutions to health inequities.
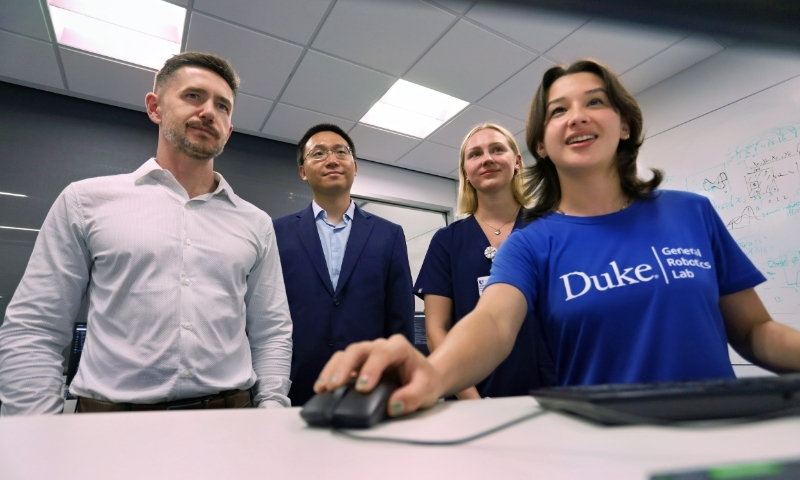
Duke University School of Nursing and Pratt School of Engineering have awarded funding to faculty investigators for four interdisciplinary projects bridging nursing and engineering science. The goal of this pilot program is to support research that accelerates knowledge and technology development to tackle health inequities, create innovative solutions considering social determinants of health, and evaluate results to improve patient care.
“Over the last several years, researchers at the School of Nursing had become increasingly interested in collaborating with researchers in Pratt because the changing healthcare landscape in the US requiring innovative solutions to the delivery of care,” said Dr. Sharron L. Docherty, Vice Dean for Research, Duke University School of Nursing .
After meetings between Dr. Docherty, and Dr. Sharon Gerecht, Associate Dean for Research & Infrastructure at Pratt , the teams decided to move forward to develop a collaborative partnership. The partnership's short-term objectives are to develop mutual awareness and orientation to the research programs of both schools, identify key collaboration opportunities, support team building and provide funding for pilot projects. In the long term, the goals are to develop large-scale research initiatives to support a center grant proposal and explore opportunities for joint scientific training programs with NIH and NSF.
“Interdisciplinary partnerships between the Pratt School of Engineering and the Duke School of Nursing are crucial for advancing healthcare innovation,” said Dr. Gerecht. By combining engineering expertise with clinical insights from nurse scientists, we can develop cutting-edge solutions that improve patient outcomes and drive the future of medical technology.
“Collaborations between nurse and engineering scientists can create transdisciplinary research teams who can move beyond merely the crossing of disciplines, to holistically integrate the fields of nursing and engineering knowledge and create solutions to problems related to health inequities,” added Dr. Docherty.
In July, each team, comprised of investigators from both schools, were awarded up to $75,000 for their pilot project. The four awarded pilot projects include:
Proof of Concept: Use of a soft wireless device placed at the suprasternal notch to support communication in people with ALS, their care-partner and a member of their social network
Xiaoyue Ni, PhD , Assistant Professor, Pratt School of Engineering Donald Bailey , PhD, RN, FAAN, Chair, Healthcare in Adult Populations Division, Associate Professor, School of Nursing
Amyotrophic Lateral Sclerosis (ALS) severely impacts motor functions related to speech, hindering social engagement. Research highlights the importance of maintaining social networks for adults with chronic illnesses to support coping and quality of life. Augmentative and alternative communication (AAC) devices help compensate for speech loss and improve quality of life. However, a systematic review found that complex AAC devices are mainly used for therapeutic interactions rather than everyday conversations, missing an opportunity to reduce social isolation. This collaboration is a proof-of-concept study to establish the acceptability, feasibility and benefit to a person with ALS and their care-partner of a skin-compliant, lightweight and wireless communication device that sits at the suprasternal notch—the divot between where the two sides of the collarbone and throat tendons meet—that measures body vibrations and movements to support and enhance communication.
Advancing Virtual Care through Nurse-Guided Telerobotics
Ryan Shaw , PhD, RN, Associate Professor and Director, Health Innovation Lab, Duke School of Nursing Boyuan Chen , PhD, MS, Assistant Professor and Director, General Robotics Lab, Duke Pratt School of Engineering
Virtual care nursing, a telehealth service, uses video conferencing to provide remote care to patients at home and in hospitals, supporting bedside care teams. It includes activities like assessments, admissions, discharges, counseling, and care support. This service helps address staffing shortages and workload issues, improving patient outcomes and satisfaction, especially in underserved rural areas. However, it can’t handle tasks requiring physical presence. Robotics could help, however, integrating this into nursing is complex. Robots need to operate safely in varied environments, learn new skills, and adapt to different users. This collaboration aims to advance nursing and robotics by recruiting nurses to train AI models to instruct robots in performing basic care tasks remotely.
Anticipating future heatwave impacts on rural mental health and substance use: implications for the development of nurse-led interventions
Marta Zaniolo , PhD, MS, Assistant Professor, Pratt School of Engineering Devon Noonan , PhD, MPH, FNP-BC, Associate Professor, Duke School of Nursing
It’s been well established that urban areas have pockets where a lack of vegetation and permeable surfaces, along with many other factors, create “urban heat islands” where temperatures soar much higher than surrounding areas. While cities are starting to allocate more resources to help these residents, rural areas face rising temperatures and lack city resources. This combination is linked to mental health issues and substance use. Researchers are working to see if they can use AI and climate data to predict heat-related thresholds that lead to an increase in mental health and substance use related hospitalizations. The team plans to put together a community advisory board to incorporate community input when building the model, but also to translate their findings into actionable solutions for rural communities throughout eastern North Carolina.
Sensor System for Monitoring Communication Difficulties at Home
Darina Petrovsky , PhD, RN, Assistant Professor, Duke School of Nursing Xiaoyue Ni , PhD, Assistant Professor, Pratt School of Engineering
Persons living with dementia experience social isolation and dysfunction, often relying on their care partner as their disease progresses while experiencing breakdowns in communication with their families and care partners. Improving relationship quality between the caregiver and the person can help lessen the caregiver’s burden and improve other related outcomes. However, we must first identify those who are experiencing communication difficulties using unobtrusive wearable technology. This proposal aims to examine the feasibility and acceptability of a low-cost, at-home, wearable sensor for the detection of communication difficulties between dementia patients and their care partner.

COMMENTS
Helping robots practice skills independently to adapt to unfamiliar environments. A new algorithm helps robots practice skills like sweeping and placing objects, potentially helping them improve at important tasks in houses, hospitals, and factories. August 8, 2024. Read full story.
Artificial Intelligence and Decision-making combines intellectual traditions from across computer science and electrical engineering to develop techniques for the analysis and synthesis of systems that interact with an external world via perception, communication, and action; while also learning, making decisions and adapting to a changing environment.
Robotics is poised to revolutionize work, education, and everyday life in much the same way the Internet did over past decades. Today, some of the most innovative and foundational robotics work is being done at SEAS and across Harvard by collaborative teams of computer scientists, mechanical engineers, electrical engineers, material scientists, applied mathematicians, designers, and medical ...
Robotics is forecast to grow at an annual growth rate of 28 percent between 2021 and 2030, a rate higher than average [1]. The average annual robotics engineering salary in the US is $101,428, including average base pay and additional pay such as profit sharing and bonuses, according to Glassdoor [2].
Robotics research focuses on understanding and designing intelligent robotic systems through rigorous analysis, system development, and field deployment. Hopkins researchers develop techniques and tools for kinematics, dynamics, control, estimation, and motion planning for deterministic and stochastic systems.
Robotics engineers help create robotic systems for human and nonhuman duties. As a robotics engineer, you'll be doing the following responsibilities: Design and build robots. Maintain and repair robots. Develop new applications for existing robots. Research to expand the potential of robotics. As a robotics engineer, you could contribute to ...
Stanford Engineering Research Introductions (SERIS) Fee Waivers; Graduate school frequently asked questions ... So the kinds of robots that we work in the research world with are more geared towards like, oh, when can we bring them into someone's home, uh, or have them at least work alongside a person in warehouses or things like that. Um, and ...
Before robotics can be broadly useful in helping with practical day-to-day tasks in people-centered spaces — spaces designed for people, not machines — they need to be able to safely & competently provide assistance to people. In 2022, we focused on challenges that come with enabling robots to be more helpful to people: 1) allowing robots ...
Discover Mechanical Engineering - This review paper provides a literature survey of collaborative robots, or cobots, and their use in various industries. ... Technical approaches: collaborative robot research can involve a range of technical approaches, including control algorithms, sensing technologies, and human-robot interface design. ...
Robotics. Having a machine learning agent interact with its environment requires true unsupervised learning, skill acquisition, active learning, exploration and reinforcement, all ingredients of human learning that are still not well understood or exploited through the supervised approaches that dominate deep learning today.
Digging Deep: Inspired by nature, the burrowing mole crab robot is a feat of engineering with real-world applications. Insect-sized robot navigates mazes with the agility of a cheetah. The 'Iron Man' body armour many of us may soon be wearing. UC Berkeley researchers create robotic guide dog for visually impaired people.
Purdue's School of Mechanical Engineering is one of the largest in the country, conducting world-class research in manufacturing, propulsion, sustainable energy, nanotechnology, acoustics, materials, biomedicine, combustion, computer simulation, HVAC and smart buildings, human-machine interaction, semiconductors, transportation, thermodynamics ...
Space robotics plays a critical role in current and future space exploration missions and enables mission-defined machines that are capable of surviving in the space environment and performing exploration, assembly, construction, maintenance, or service tasks. Modern space robotics represents a multidisciplinary emerging field that builds on ...
The Robotics Minor consists of four undergraduate ROB courses. The minor teaches the fundamentals of robotics: kinematics, dynamics, manipulation, locomotion, planning, vision, and human-robot interaction. Students will have hands-on experience. Interested students should allow 4 semesters (two years) to complete the four courses for the minor ...
Robots and Artificial Intelligence. From babybots to surprisingly accomplished robots, read all the latest news and research in robotics here.
Robotics. Home. Enable robots and other agents to develop broadly intelligent behavior through learning and interaction. Exploring the intersection of machine learning and robotic control, including. end-to-end learning of visual perception and robotic manipulation skills, deep reinforcement learning of general skills from autonomously ...
The lead author is Anand Mishra, a research associate in the Organic Robotics Lab led by Rob Shepherd, professor of mechanical and aerospace engineering at Cornell University, and the paper's ...
CONTACT. Location: Unity Hall. Phone: 508-831-6665. [email protected]. WPI is at the forefront of integrated robotics research and education with the Robotics Engineering Department, which is the first-of-its-kind in the nation and the most comprehensive in the world and with the largest student population seeking BS, MS, and PhD degrees.
On land, in air, in space, and underwater, as individuals and as teams, autonomous machines increasingly impact infrastructure, healthcare, security, manufacturing, and the environment. Our research in robotics and autonomy drives fundamental advances in materials, actuation, and sensing and control; sensorimotor integration; locomotion ...
Robots that walk, run, jump, creep, roll, and fly. Researchers explore legged robotics to design better controllers for stable, energy efficiency, fast locomotion as well as the ability to reliably travel over unstructured terrain - like the moon. Our faculty are creating technologies for infrastructure inspection, aerial load transportation ...
Mechanical and Aerospace Engineering. Robotic devices and systems. Autonomous air, sea, undersea, and land vehicles. Space exploration and development. Intelligent control systems. Biomimetic modeling, dynamics, and control. Cooperating robots for manufacturing and assembly. Cooperative control of natural and engineered groups.
The magnetically actuated miniature robot is shown in Fig. 2, which is fabricated using 3D printing with photosensitive resin through projection micro-stereolithography (nanoArch S140, Boston Micro Fabrication, China).The helical shell measures approximately 7 mm in length and 3 mm in diameter, with helical blade heights and thicknesses of 0.3 mm and 0.1 mm, respectively.
Research in Robotics at the USC Viterbi Department of Aerospace and Mechanical Engineering spans many different areas including motion planning, robot learning and control, robot manipulation, bio-inspired robotics, and human-robot collaboration. Browsing through the websites of our faculty and research labs, you will find a wide range of ...
It Starts with Research—and Leads to Virtually Endless Opportunities Scientific inquiry can end up in an unlimited number of destinations. But invariably, it all begins at the same starting point: research. Tireless research helped WPI identify the growth and proliferation of robotics in increasing areas of society. This led to the recognition of the burgeoning demand for qualified robotics ...
A wheeled bot rolls across the floor. A soft-bodied robotic star bends its five legs, moving with an awkward shuffle. Powered by conventional electricity via plug or battery, these simple robotic ...
Engineering innovations transform our lives and energize the economy. The U.S. National Science Foundation announces a five-year investment of $104 million, with a potential 10-year investment of up to $208 million, in four new NSF Engineering Research Centers (ERCs) to create technology-powered solutions that benefit the nation for decades to come.
Fostering students' competence in applying interdisciplinary knowledge to solve problems has been recognized as an important and challenging issue globally. This is why STEM (Science, Technology, Engineering, Mathematics) education has been emphasized at all levels in schools. Meanwhile, the use of robotics has played an important role in STEM learning design. The purpose of this study was ...
Robotics Research Scientist: Engages in scientific research to advance the field of robotics, contributing to the development of new technologies and methodologies. Bio-Inspired Robotics Engineer: Draws inspiration from biological systems to design robots that mimic or are inspired by natural organisms, exploring biomimicry in robotics.
University of Idaho College of Engineering students work directly with state-of-the-art mobile and full-size robots used in a variety of applications - from industrial to therapeutic! Uidaho, #uidaho ... Center for Intelligent Industrial Robotics. Integrating new robotics research and training labs across the state to prepare students to fill ...
Advancing Virtual Care through Nurse-Guided Telerobotics . Ryan Shaw, PhD, RN, Associate Professor and Director, Health Innovation Lab, Duke School of Nursing Boyuan Chen, PhD, MS, Assistant Professor and Director, General Robotics Lab, Duke Pratt School of Engineering. Virtual care nursing, a telehealth service, uses video conferencing to provide remote care to patients at home and in ...